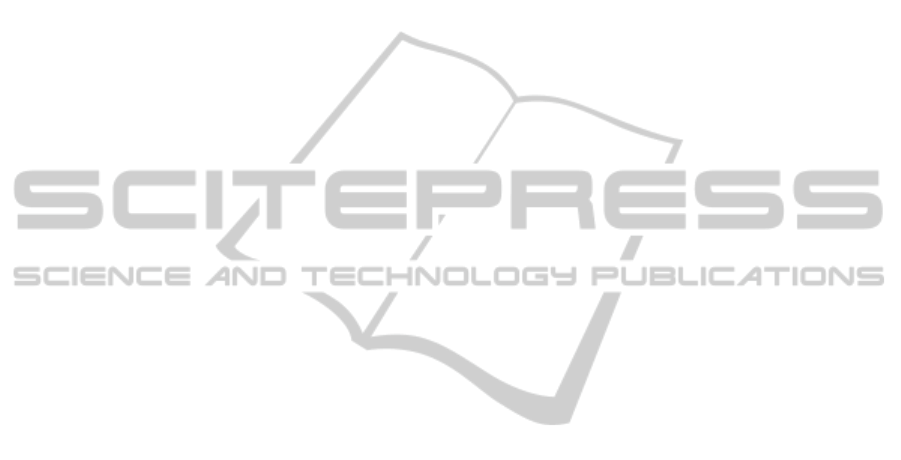
graphs, a second optimization approach known as
the Compromise Programming is proposed and
applied. The basic idea of CP is to identify an ideal
solution (utopia point) where each attribute under
consideration achieves its optimum value (Adeyeye
et. al., 2010,
Anita et. al. , 2012).
This enhanced optimization model in robust
design considers both the product/process bias and
the variance. It was numerically demonstrated that
this proposed model might provide a better solution
in terms of control factor settings in an FMS or other
environments. Many of the previous studies have
concentrated on the minimization of the variance
while keeping the bias at zero. But, it has been
shown (Cho et al, 2000) that there are situations
where the minimum variance with a zero bias may
not provide the minimum expected loss.
When compared to the existing methods for
robust optimization such as Taguchi’s S/N ratio, the
proposed approach has many advantages (Chen et
al. 1998), namely: capability of generating the
efficient solutions, interactive robust design
procedure, significance of the multi-objective
approach to robust design, etc. As a research
strategy however, we suggest that these two methods
be used together, especially when there are
qualitative factors involved. We propose that the
region of investigation be determined by the TM
before using CP. When TM is used alone, the
interaction factors cannot be fully taken into account
due to the limit of the linear graph in the orthogonal
array.
On one hand, the optimization is only done over
the points (three levels in this study) considered in
the design. These points (factor levels) may not lead
the true optimum when continuous variable are
involved. On the other hand the CP approach is
unable to handle qualitative variables. Using the two
methods combined will help designers to determine
what level of the input factors and AGV and
machine dispatching rules will maximize the
Throughput Rate for a specific FMS. Simulation,
Taguchi and CP approaches to RD are powerful
tools to improve the design and performance in the
FMS environment. Further research may consider
multiple performances measures instead of one used
in this study.
REFERENCES
Adeyeye et. al., 2012. Multi-objective methods for
welding flux performance Optimization, RMZ –
Materials and Geoenvironment, Vol. 57, No. 2, pp.
251-270.
Anita et. al. 2012, A Mathematical Analysis of
Compromising Programming Techniques. IOSR
Journal of Mathematics ISSN: 2278-5728. Vol. 3.
Berger, J.O., 1985,.Statistical Decision Theory and
Bayesian Analysis (New York: Springer-Verlag).
Chen, W., Wiecek, M.M., Zhang, J., 1998, Quality Utility-
A Compromise Programming Approach to Robust
Design, ASME Design Technical Conference, paper
no. DAC5601, Atlanta, GA.
Cho, B.-R, Kim, Y.J., Kimbler, D.L., and Philips, M.D,
2000. An Integrated Joint Optimization Procedure for
Robust and Tolerance Design. International Journal of
Production Research, Vol. 38, N°10, 2309-2325
Pignatiello, J. and Ramberg, J.S., 1991, Top Ten Triumphs
and Tragedies of Genichi Taguchi. Quality
Engineering, Vol. 4, N°2, 221-225.
Gharis, 2012. A Compromise Programming Approach to
Effectively Value and Integrate Forest Carbon
Sequestration into Climate Change Policy.
Dissertation, Carolina State University, Department of
Forestry and Environmental Resources , Raleigh, NC.
Gorantiwar et. al., 2010, Multicriteria Decision Making
(Compromise Programming) for Integrated Water
Resources Management in an Irrigation Scheme,
Future State of Water Resources & the Environment,
EWRI-ASCE, Chennai.
Park, T., Lee, H., and Lee H., 2001. FMS Design model
with multiple objectives using compromise
programming, International Journal of Production
Research, Vol.39, N°15, 3513-28.
Randhir T. O., Lee, J. G., Engel, B., 2000. Multiple
Criteria Dynamic Spatial Optimization to Manage
Water Quality on a Watershed Scale. Transactions of
the ASAE, VOL. 43(2): 291-299 © 2000.
Ribeiro, J.L., and Elsayed, E.A., 1995. A Case Study on
Process Optimization Using the Gradient Loss
Function, International Journal of Production
Research, Vol. 33, no. 12, 3233-48.
Shang, J.S., 1995. Robust design and optimization of
material handling in an FMS, International Journal of
production Research, Vol.33, N°9, 2437-2454.
Taguchi, G., 1985. Introduction to Quality Engineering
(MI: American Supplier Institute); 1987, System of
Experimental Design, edited by Don Clausing, Vol.1
and 2 (New York: UNIPUB/Kraus International
Publications).
Tshibangu, WM Anselm, 2013. A Two-Step Empirical-
Analytical Optimization Scheme, A DOE-Simulation
Meta-Modeling Approach, 10
th
International
Conference on Informatics in Controls, Automation
and Robotics.
Tshibangu, WM. Anselm, 2004, Multiple Optimization of
a FMS Using a Multivariate Quadratic Loss Function,
International Journal of Industrial Engineering
Proceedings of the 9th Annual International
Conference on Industrial Engineering Theory,
Applications and Practice, Auckland, New Zealand.
Zeleny, M., 1974, A Concept of compromise solutions and
the method of displaced ideal. Computer and
Operations Research, Vol. 1, 479-496.
ICINCO2015-12thInternationalConferenceonInformaticsinControl,AutomationandRobotics
492