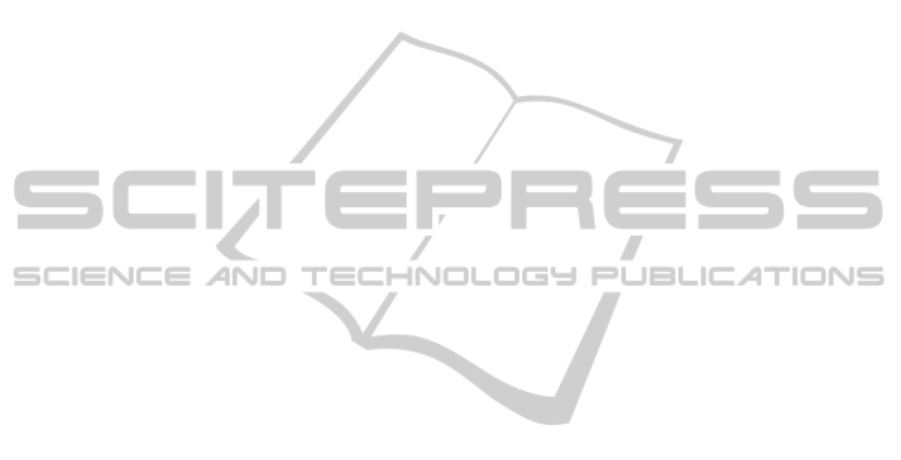
Bessembinder, H. and Kaufman, H. M. (1997). A cross-
exchange comparison of execution costs and informa-
tion flow for NYSE-listed stocks. Journal of Financial
Economics, 46(3):293–319.
Camerer, C. F. (1998). Can asset markets be manipulated?
a field experiment with racetrack betting. Journal of
Political Economy, 106:457–482.
Chakraborty, A. and Yilmaz, B. (2004). Manipulation in
market order models. Journal of Financial Markets,
7(2):187–206.
Corwin, S. A. and Schultz, P. (2012). A simple way to es-
timate bidask spreads from daily high and low prices.
Journal of Finance, 67(2):719–760.
EOG (2006). Tradesports’ bad call.
http://forums.eog.com/showthread.php/39353-
Tradesports-Bad-Call.
Fama, E. F. (1970). Efficient capital markets: A review of
theory and empirical work. The Journal of Finance,
25(2):383–417.
Fidler, M. (2014). Anarchy of Regulation: Controlling the
Global Trade in Zero-Day Vulnerabilities. PhD thesis,
Stanford University.
Glosten, L. R. and Milgrom, P. R. (1985). Bid, ask and
transaction prices in a specialist market with heteroge-
neously informed traders. Journal of Financial Eco-
nomics, 14(1):71–100.
Google (2014). Projectzero.
http://googleprojectzero.blogspot.be/.
Hansen, J., Schmidty, C., and Strobelz, M. (2004). Manip-
ulation in political stock markets - preconditions and
evidence. Applied Economics Letters, pages 459–463.
Hanson, R. (2003). Combinatorial information market de-
sign.
Hanson, R. (2006). Designing real terrorism futures. Public
Choice, 128(1-2):257–274.
Hanson, R., Oprea, R., and Porter, D. (2006). Information
aggregation and manipulation in an experimental mar-
ket. Journal of Economic Behavior & Organization,
60(4):449–459.
Harris, L. (2002). Trading and exchanges: Market mi-
crostructure for practitioners. Oxford University
Press.
Hillion, P. and Suominen, M. (2004). The manipula-
tion of closing prices. Journal of Financial Markets,
7(4):351–375.
Johannesson, P. and Perjons, E. (2014). An Introduction to
Design Science. Springer International Publishing, 1
edition. ISBN: 978-3-319-10631-1.
Kumar, P. and Seppi, D. J. (1992). Futures manipula-
tion with cash settlement. The Journal of Finance,
47(4):1485–1502.
Luckner, S. (2008). Prediction markets: Fundamentals,
key design elements, and applications. The 21st Bled
eConference, eCollaboration: Overcoming Bound-
aries Through Multi-Channel Interaction.
Pandey, P. and Snekkenes, E. (2014a). Applicability of pre-
diction markets in information security risk manage-
ment. In Database and Expert Systems Applications
(DEXA), 2014 25th International Workshop on, pages
296–300.
Pandey, P. and Snekkenes, E. (2014b). Using prediction
markets to hedge information security risks. In Mauw,
S. and Jensen, C., editors, Security and Trust Man-
agement, volume 8743 of Lecture Notes in Computer
Science, pages 129–145. Springer International Pub-
lishing.
Pennock, D. M. (2004). A dynamic pari-mutuel market for
hedging, wagering, and information aggregation. In In
Proc. of the 5th ACM Conf. on Electronic Commerce,
pages 170–179.
Plott, C. R. and Chen, K.-Y. (2002). Information Aggre-
gation Mechanisms: Concept, Design and Implemen-
tation for a Sales Forecasting Problem. W.P. 1131,
California Institute of Technology.
Rhode, P. W. and Strumpf, K. S. (2004). Historical presi-
dential betting markets. The Journal of Economic Per-
spectives, 18(2):127–141.
Spann, M. (2002). Virtuelle B¨orsen Als Instrument Zur
Marktforschung. Deutscher Universit¨ats-Verlag.
Sripawatakul, P. and Sutivong, D. (2010). Decision frame-
work for constructing prediction markets. In The 2nd
IEEE Int. Conf. on Information Management and En-
gineering (ICIME), 2010.
Teschner, F. (2012). Forecasting Economic Indices De-
sign, Performance, and Learning in Prediction Mar-
kets. PhD thesis, Karlsruher Institut fr Technologie.
TradeSports (2015). Tradesports.
https://www.tradesports.com/.
WEF (2014). Global risks 2014. Insight Report 9th Edn.,
No.: 090114, World Eco. Forum, Geneva.
Weinhardt, C. and Gimpel, H. (2007). Market engineering:
An interdisciplinary research challenge. In Jennings,
N., Kersten, G., Ockenfels, A., and Weinhardt, C., ed-
itors, Negotiation and Market Engineering, number
06461. IBFI, Germany.
SECRYPT2015-InternationalConferenceonSecurityandCryptography
284