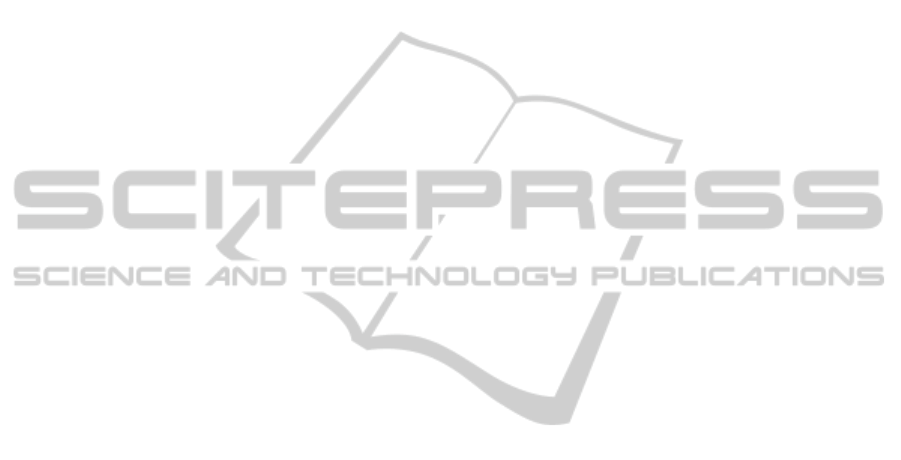
activate the control in order to stabilize the temper-
ature. There are some cases where the control acti-
vation leads to increment the emissions immediately,
which can give us an idea that this kind of control can
also lead to economic growth.
This simulation tool with the fuzzy control pro-
posed is a very powerful instrument in climate change
policy and international agreements. The configura-
tion options allow us to project under certain circum-
stances that answer the questions: When are we tak-
ing action? and, What is our goal stabilization tem-
perature?
The results will give us not just how many GtC
we should decrease or increase every year, but also
the percentage of emissions relative to the previous
year, which could give us an idea of how much it will
cost us (Figure 4).
This instrument is a common ground where spe-
cialists in diverse areas of climate change could con-
tribute in order to set the parameters that we should
explore and simulate so that the we can make the best
decisions.
In future work, we seek to not only project the
amount of carbon emissions that should be changed,
but also the amount which each country should con-
tribute to accomplish the goal, based on a fuzzy infer-
ence system that asses every country possibilities ans
responsibility.
REFERENCES
Boden, T. A., Marland, G., and Andres, R. J. (2013).
Global, regional, and national fossil-fuel co2 emis-
sions.
Javadikia, P., Tabatabaeefar, A., Omid, M., Alimardani, R.,
and Fathi, M. (2009). Evaluation of intelligent green-
house climate control system, based fuzzy logic in
relation to conventional systems. In Artificial Intel-
ligence and Computational Intelligence, 2009. AICI
’09. International Conference on, volume 4, pages
146–150.
King, D. (2011). ”copnhagen and cancun”, internationl cli-
mate change negotiations: Key lessons and next steps.
Smith School of Enterpise and the Environment, Uni-
versity of Oxford, :12.
Maier-Reimer, E. and Hasselman, K. (1987). Transport
and storage of co2 in the ocean - an inorganic ocean-
circulation carbon cycle model. Clim Dynam, (2):63–
90.
Martinez-Lopez, B. and Gay-Garcia, C. (2011). Fuzzy con-
trol of co2 emissions. Proceedings: Eigth Interna-
tional Conference on Fuzzy Systems and Knowledge
Discovery.
Moss, R., Babiker, M., Brinkman, S., Calvo, E., Carter, T.,
Edmonds, J., Elgizouli, I., Emori, S., Erda, L., Hib-
bard, K., Roger, J., Kainuma, M., Kelleher, J., Lamar-
que, J. F., Manning, M., Matthews, B., Meehl, J.,
Meyer, L., Mitchell, J., Nakicenovik, N., O’Neill, B.,
Pichs, R., Riahi, K., Rose, S., Runc, P., Stouffer, R.,
van Vuuren, D., Weyant, J., Wilbanks, T., van Yper-
sele, J. P., and Zurek, M. (2007). Towards new scenar-
ios for analysis of emissions, climate change, impacts,
and response strategies. IPCC Expert meeting report.
NASA (2014). Giss surface temperature analysis (gistemp).
giss.nasa.gov.
Parry, N., Palutikof, J., Hanson, C., and Lowe, J. (2008).
Squaring up to reality. Nature Reports Climate
Change, :68–71.
Tahvonen, O., Von Storch, H., and Von Storch, J. (1994).
Economic efficency of co2 reduction programs. Clim
Res, (4):127–141.
Tong, R. (1977). A control engineering review of fuzzy
systems. Automatica, 13(6):559 – 569.
StabilizingGlobalTemperatureThroughaFuzzyControlonCO2Emissions
531