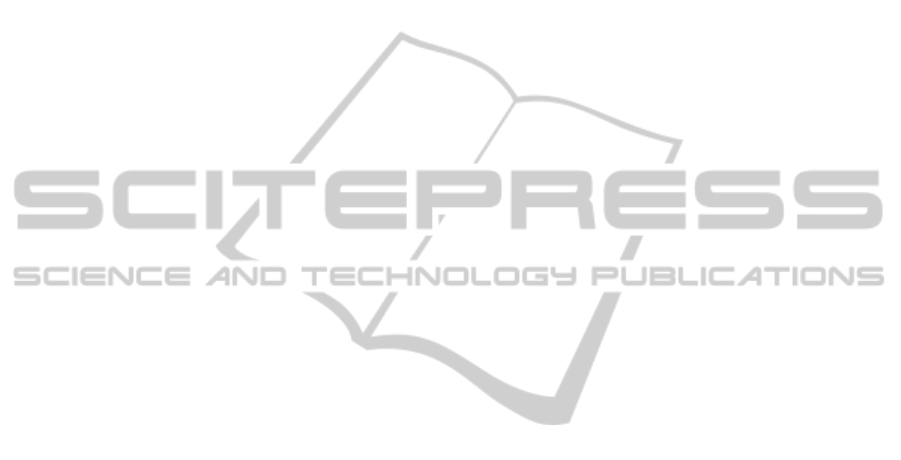
prediction. The execution time differences between
the methodologies analyzed, as expected, are really
big since the MOGUL approach performs a three
level optimization. While FIR needs around 10
minutes to perform a complete 5-CV prediction,
GFS-GPG-R about half an hour, SVM almost 2
hours and the MOGUL approaches need about 24
hours.
Encouraging results are achieved with the FIR
model providing the best performance,
outperforming the rest of the hybrid fuzzy
approaches studied. Moreover, the FIR results are
slightly better than the best ones obtained previously
for the same problem by Cortez et al. (2009), when
using SVM. An important advantage of FIR
methodology with respect SVMs is its reduced
computational time. FIR models are synthesized
rather than trained, allowing a quick modelling and
prediction computation. The difference in
computational time between FIR and SVM is
considerable, as stated before.
5 CONCLUSION
This work aims at the prediction of wine preferences
from physicochemical properties tests that are
available at the wine quality certification step. A
large dataset is accessible which contains white wine
samples from the northwest region of Portugal.
Four powerful hybrid fuzzy techniques that
perform data mining are studied in this research. In
the one hand the Fuzzy Inductive Reasoning (FIR)
methodology that is a non-parametric inductive
technique based on fuzzy logic and machine learning
approaches. On the other hand 3 different Genetic
Fuzzy Systems (GFS) that perform fuzzy rule
learning i.e. GFS-GPG-R, MOGUL-TSK-R and
MOGUL-IRLHC-R.
The GFS are much more computational
expensive than FIR since perform different
optimization levels using evolutionary algorithms.
On the other hand, FIR performs feature
selection during the modeling process, concluding
that the features that have highest relevant causal
relation with the wine quality are: alcohol, fixed
acidity, free sulfur dioxide, residual sugar and
volatile acidity. Citric acid and sulphates are also
variables that have causal relation with the wine
quality but not with the same strength than the
previous ones.
FIR, using the previously mentioned variables,
achieves the best performances, outperforming not
only the hybrid fuzzy techniques studied in this
article, but also other data mining methodologies
reported in other studies (Cortez et a., 2009), such
are Neural Networks (NN), Multiple Regression
(MR) and Support Vector Machines (SVM).
The results obtained using the SVM have very
similar error and accuracy metrics than the FIR
results. However, FIR has a great advantage over
SVM with respect the computational time.
As mentioned in all the studies that deal with
wine quality prediction, the results are really
relevant for different aspects of the wine industry.
On the one hand a good prediction can be very
useful in the certification phase. On the other hand,
such a prediction system can also be useful for
training oenology students or for marketing
purposes.
REFERENCES
Alcalá, R, Alcalá-Fdez. J., Casillas, J., Cordón, O.,
Herrera, F., 2007. Local identification of prototypes
for genetic learning of accurate TSK fuzzy rule-based
systems. International Journal of Intelligent Systems,
22, 909-941.
Alcalá-Fdez, J., Sánchez, L., García, S., del Jesus, M.J.,
Ventura, S., Garrell, J.M., Otero, J., Romero, C.,
Bacardit, J., Rivas, V.M., Fernández, J.C., Herrera, F.,
2009. KEEL: A Software Tool to Assess Evolutionary
Algorithms to Data Mining Problems. Soft Computing,
13:3, 307-318.
Carvajal, R., Nebot, A., 1998. Growth Model for White
Shrimp in Semi-intensive Farming using Inductive
Reasoning Methodology. Computers and Electronics
in Agriculture 19, 187-210.
Cordon, O., Herrera, F., 2001. Hybridizing genetic
algorithms with sharing scheme and evolution
strategies for designing approximate fuzzy rule-based
systems. Fuzzy sets and systems, 118, 235-255.
Cordon, O., Herrera, F., Hoffmann, F., Magdalena, L.,
2001. Genetic Fuzzy Systems. Evolutionary Tuning
and Learning of Fuzzy Knowledge Bases. Vol. 19 of
Advances in Fuzzy Systems - Applications and
Theory. World Scientific.
Cortez, P., Cerdeira, A., Almeida, F., Matos, T., Reis, J.,
2009. Modeling wine preferences by data mining from
physicochemical properties. In Decision Support
Systems, Elsevier, 47(4), 547-553.
Ebeler, S., 1999. Flavor Chemistry: Thirty Years of
Progress, Klumer Academic Publishers, 409-422.
Escobet, A., Nebot., A., Cellier, F.E., 2008. Visual-FIR: A
tool for model identification and prediction of
dynamical complex systems. Simulation Modelling
Practice and Theory 16, 76-92.
Keel Platform,2005. http://sci2s.ugr.es/keel/developpment.
php.
Klir, G., Elias, D., 2002. Architecture of Systems Problem
Solving, Plenum Press. New York, 2
nd
edition.
SIMULTECH2015-5thInternationalConferenceonSimulationandModelingMethodologies,Technologiesand
Applications
506