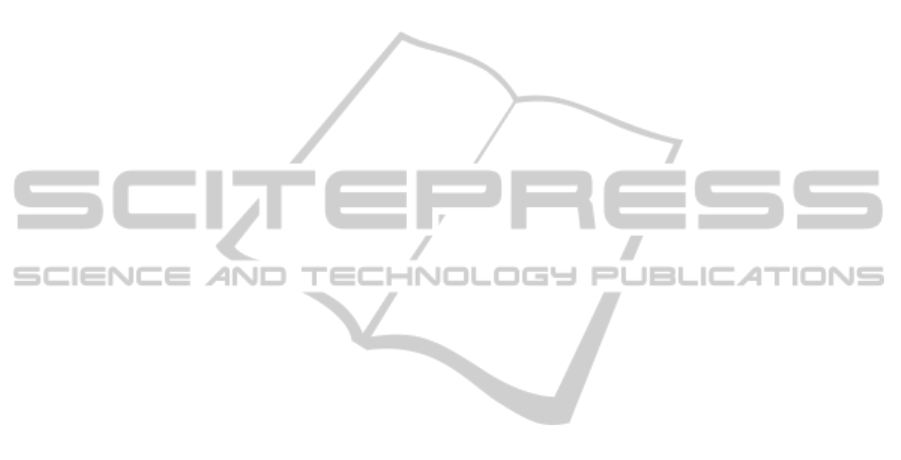
5 RECOMMENDATIONS
We have established two global indicators of positive
impact and implementation complexity, to measure
the business value of data accuracy improvement
projects.
According to the values of these indicators and to
the targeted accuracy level (to-be), two business cases
may be considered:
The first one is to improve the processes
(reengineering, control, etc.), by enhancing
their execution accuracy. This is a short term
option that is generally less expensive, but
requires change management because it affects
the working methods;
The second one is based on the improvement of
data accuracy by determining and analyzing the
sources of low quality, such as uncontrolled
data acquisition, update problems, etc.
Since the automation of business processes
guarantees, in a way, the quality of their execution,
actions must be directed towards the improvement of
the accuracy of the data used by these processes. Our
approach highlights the most cost-effective data
accuracy improvement projects.
6 CONCLUSIONS AND FUTURE
WORK
The result of the work accomplished thus far shows
how to measure in a quantitative manner, the business
value of data quality improvement projects, by
establishing two global indicators of positive impact
and implementation complexity.
In this paper, only the assessment of data accuracy
projects was covered. One or more case studies’
validation is necessary.
Furthermore, and in order to recommend the
optimal business case to improve data accuracy and
thus, the overall organization’s performance, an
optimization algorithm is under development to
identify the optimal data accuracy level, taking
account of: 1) – the initial data accuracy level (as-is),
2) – the positive impact of the key process that uses
the data, 3) – the implementation complexity of data
accuracy improvement initiative, and 4) - the targeted
data accuracy (to-be).
REFERENCES
Aladwani, A. M., & Palvia, P. C., 2002. Developing and
validating an instrument for measuring user-perceived
web quality. Information & management, 39(6), 467-
476.
Batini, C., Comerio, M., & Viscusi, G., 2012. Managing
quality of large set of conceptual schemas in public
administration: Methods and experiences. In Model and
Data Engineering (pp. 31-42). Springer Berlin
Heidelberg.
Eppler, M., & Helfert, M.,2004. A classification and
analysis of data quality costs. In International
Conference on Information Quality.
Gartner, Oct 2011. Measuring the Business Value of Data
Quality. https://www.data.com/export/sites/data/com
mon/ assets/pdf/DS_Gartner.pdf.
Haug, A., Zachariassen, F., & Van Liempd, D.,2011. The
costs of poor data quality. Journal of Industrial
Engineering and Management, 4(2), 168-193.
International Association for Information and Data Quality,
2015. IQ/DQ glossary.
http://iaidq.org/main/glossary.shtml.
Narman, P., Johnson, P., Ekstedt, M., Chenine, M., &
Konig, J., 2009. Enterprise architecture analysis for
data accuracy assessments. In Enterprise Distributed
Object Computing Conference, 2009. EDOC'09. IEEE
International (pp. 24-33). IEEE.
Otto, B., Hüner, K. M., & Österle, H. (2009). Identification
of Business Oriented Data Quality Metrics. In ICIQ
(pp. 122-134).
Wang, R. Y., & Strong, D. M.,1996. Beyond accuracy:
What data quality means to data consumers. Journal of
management information systems, 5-33.
Pipino, L. L., Lee, Y. W., & Wang, R. Y., 2002. Data
quality assessment. Communications of the ACM,
45(4), 211-218.
DATA2015-4thInternationalConferenceonDataManagementTechnologiesandApplications
194