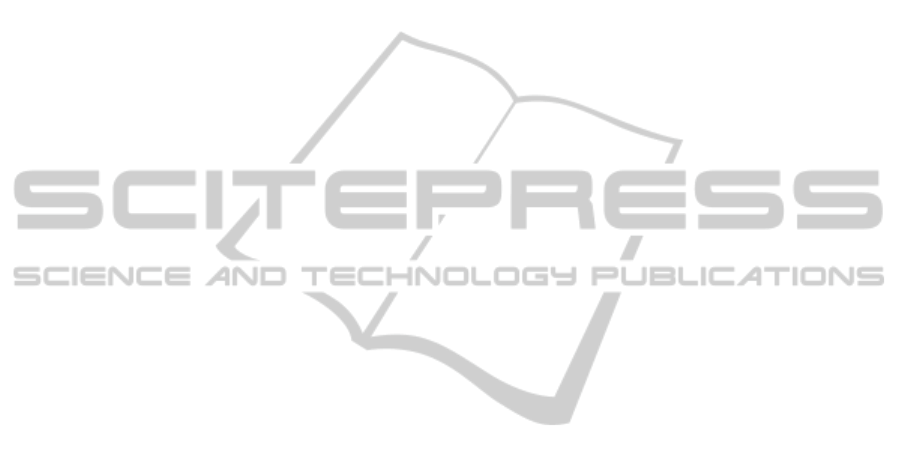
International Publishing, 2014. p. 3-15.
Bizer, Christian. The emerging web of linked data.
Intelligent Systems, IEEE, 2009, vol. 24, no 5, p. 87-
92.
Claerbout, Jon F., Karrenfach, Martin. Electronic
documents give reproducible research a new meaning.
In: 1992 SEG Annual Meeting. Society of Exploration
Geophysicists, 1992.
Chesbrough, Henry, Vanhaverbeke, Wim, West, Joel
(ed.). Open innovation: Researching a new paradigm.
Oxford university press, 2006.
Eaton, John W. Gnu octave and reproducible research.
Journal of Process Control, 2012, vol. 22, no 8, p.
1433-1438.
Fecher, Benedikt, Friesike, Sascha. Open Science: one
term, five schools of thought. In : Opening Science.
Springer International Publishing, 2014. p. 17-47.
Feller, Joseph, Fitzgerald, Brian. Understanding open
source software development. London : Addison-
Wesley, 2002.
Floca, Ralf. Challenges of Open Data in Medical
Research. In : Opening Science. Springer International
Publishing, 2014. p. 297-307.
Geuna, Aldo, Nesta, Lionel JJ. University patenting and its
effects on academic research: The emerging European
evidence. Research Policy, 2006, vol. 35, no 6, p. 790-
807.
Jomier, Julien, Bailly, Adrien, Le Gall, Mikael, et al. An
open-source digital archiving system for medical and
scientific research. Open Repositories, 2010, vol. 7.
Kilgarriff, Adam. Googleology is bad science.
Computational linguistics, 2007, vol. 33, no 1, p. 147-
151.
Kauppinen, Tomi, de Espindola, Giovana Mira. Linked
open science-communicating, sharing and evaluating
data, methods and results for executable papers.
Procedia Computer Science, 2011, vol. 4, p. 726-731.
Lindman, Juho, Tammisto, Yulia. Open Source and Open
Data: Business Perspectives from the Frontline. In :
Open Source Systems: Grounding Research. Springer
Berlin Heidelberg, 2011. p. 330-333.
Miller, Paul, Styles, Rob, Heath, Tom. Open Data
Commons, a License for Open Data. LDOW, 2008,
vol. 369.
Murray-Rust, Peter. Open data in science. Serials Review,
2008, vol. 34, no 1, p. 52-64.
Paolacci, Gabriele, Chandler, Jesse, & Ipeirotis, Panagiotis
G. Running experiments on amazon mechanical turk.
Judgment and Decision making, 2010, vol. 5, no 5, p.
411-419.
Penev, Lyubomir, Sharkey, Michael, Erwin, Terry, et al.
Data publication and dissemination of interactive keys
under the open access model. ZooKeys, 2009, vol. 21,
p. 1-17.
Piwowar, Heather A., Day, Roger S., et Fridsma, Douglas
B. Sharing detailed research data is associated with
increased citation rate. PloS one, 2007, vol. 2, no 3,
p.
e308.
Reichman, O. J., Jones, Matthew B., et Schildhauer, Mark
P. Challenges and opportunities of open data in
ecology. Science, 2011, vol. 331, no 6018.ank-
Spektrum, 14(15-21), 48.
Schildhauer, Thomas, Voss, Hilger. Open Innovation and
Crowdsourcing in the Sciences. In Opening Science.
Springer International Publishing, 2014. p. 255-269.
Sitek, Dagmar, Bertelmann, Roland. Open Access: A State
of the Art. In: Opening Science. Springer
International Publishing, 2014. p. 139-153.
Stodden, Victoria. The legal framework for reproducible
scientific research: Licensing and copyright.
Computing in Science & Engineering, 2009, vol. 11,
no 1, p. 35-40.
Teif, Vladimir B. Science 3.0: Corrections to the Science
2.0 paradigm. arXiv preprint arXiv:1301.2522, 2013.
Vandewalle, Patrick et al. Reproducible research in signal
processing. Signal Processing Magazine, IEEE, 2009,
vol. 26, no 3, p. 37-47.
Viseur, Robert, Charlier, Etienne, Van de Borne Michael.
Cloud Computing and Technological Lock-in:
Literature Review, Data Technologies and
Applications, Vienna, Austria, 2014.
Viseur Robert. Extraction of Biographical Data from
Wikipedia, Data Technologies and Applications,
Reykjavik, Iceland, 2013.
DATA2015-4thInternationalConferenceonDataManagementTechnologiesandApplications
206