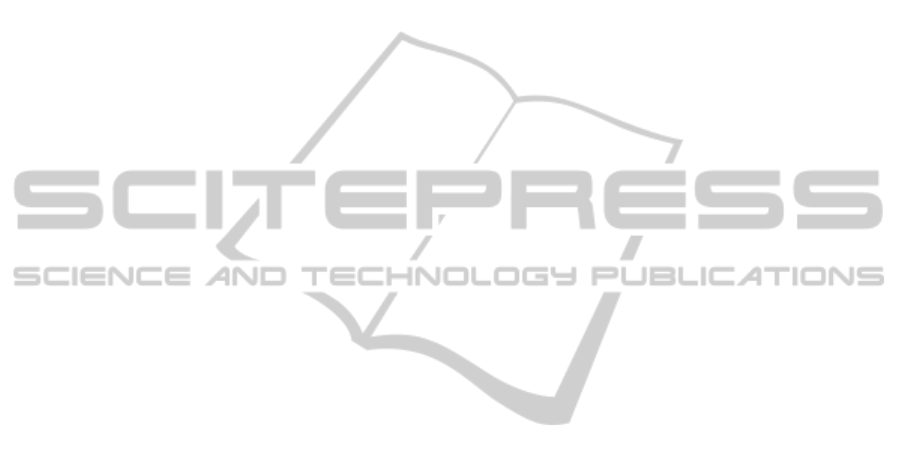
task. Note that in general there is not a linear relation
between interface and skills parameters, and the map
from one to the other can be much more complex than
the examples provided here.
6 CONCLUSIONS
In this paper we proposed a general framework
for the description of interface design for human-
machine interaction tasks involving motor-learning
skills. In particular, two well-established approaches,
the goal-oriented approach and human-centered ap-
proach, have been reviewed. Their advantages and
drawbacks have been analyzed in terms of usabil-
ity and performance, considering also their temporal
evolution.
During the training phase of every long-term
human-machine interaction, a process of neuroplas-
ticity occurs: the user adapts to the interface and
his/her motor skills improve. If, at the same time,
the interface adjusts its characteristic parameters, the
whole process can be referred to as a process of co-
adaptation. The analysis of time-adjusting interfaces
coming from the literature reveals that the temporal
evolution of the parameters related to co-adaptation
(and in particular to the user’s performance) has not
been fully exploited. In particular, the role of time-
related indexes that capture changes in motor and cog-
nitive abilities of the user has been overlooked.
A novel approach named progressive co-
adaptation is proposed to fill the gap. In this
framework, human performance is continuously
monitored and the system makes inferences about
changes in the users’ cognitive and motor skills. As
argued in the paper, progressive co-adaptation at-
tempts to combine the advantages derived from both
the goal-oriented and human-centered approaches,
while it mitigates their drawbacks. Indeed, for a
more effective long-term co-adaptation process, the
interface should be able to predict and adjust its
parameters according to the evolution of human skills
and performance (and not only to the actual ones).
To validate the proposed approach we plan to im-
plement it for two practical applications of teleoper-
ation and assistive technology, namely robotic tele-
manipulation and active vision for the visually im-
paired. For both cases, initial challenges have been
described and potential solutions based on progres-
sive co-adaptation have been discussed. We are rea-
sonably confident that future research in this direc-
tion will highlight the advantages of the proposed ap-
proach.
ACKNOWLEDGEMENTS
We thank the EPSRC Network on Visual Image Inter-
pretation in Humans and Machines for fostering this
collaboration.
REFERENCES
Bajcsy, R. (1988). Active perception. Proc. of the IEEE,
76(8):966–1005.
Bellotto, N. (2013). A multimodal smartphone interface for
active perception by visually impaired. In IEEE SMC
Int. Workshop on Human-Machine Systems, Cyborgs
and Enhancing Devices (HUMASCEND).
Bi, L., Fan, X.-A., and Liu, Y. (2013). Eeg-based brain-
controlled mobile robots: A survey. IEEE Transac-
tions on Human-Machine Systems, 43(2):161–176.
Bryan, M., Martin, S., Cheung, W., and Rao, R. (2013).
Probabilistic co-adaptive brain-computer interfacing.
Journal of Neural Engineering, 10(6).
Carlson, T. and Demiris, Y. (2012). Collaborative control
for a robotic wheelchair: Evaluation of performance,
attention, and workload. IEEE Trans. on Systems,
Man, and Cybernetics – Part B, 42(3):876–888.
Chan, L., Naghdy, F., and Stirling, D. (2014). Applica-
tion of adaptive controllers in teleoperation systems:
A survey. IEEE Transactions on Human-Machine Sys-
tems, 44(3):337–352.
Chen, Z., Liang, B., Zhang, T., Zhang, B., and Song, H.
(2014). An adaptive force reflection scheme for bilat-
eral teleoperation. Robotica.
Christoudias, C., Saenko, K., Morency, L.-P., and Darrell,
T. (2006). Co-adaptation of audio-visual speech and
gesture classifiers. In ICMI’06: 8th International
Conference on Multimodal Interfaces, pages 84–91.
Cui, Y. and Hua, J. (2013). Human behavior characteristics
analysis in teleoperation system. Applied Mechanics
and Materials, 373-375:163–166.
Dixon, J. (2012). Human Factors in Reliable Design, pages
137–155. John Wiley & Sons, Inc.
Furuta, K. (2003). Control of pendulum: From super
mechano-system to human adaptive mechatronics. In
Proceedings of the IEEE Conference on Decision and
Control, volume 2, pages 1498–1507.
Furuta, K., Kado, Y., Shiratori, S., and Suzuki, S. (2011).
Assisting control for pendulum-like juggling in hu-
man adaptive mechatronics. Proceedings of the In-
stitution of Mechanical Engineers. Part I: Journal of
Systems and Control Engineering, 225(6):709–720.
G
¨
urel, T. and Mehring, C. (2012). Unsupervised adapta-
tion of brain-machine interface decoders. Frontiers in
Neuroscience, 6(16).
Harashima, F. and Suzuki, S. (2010). State-of-the-art in-
telligent mechatronics in human-machine interaction.
IEEE Industrial Electronics Magazine, 4(2):9–13.
Hoey, J., Poupart, P., von Bertoldi, A., Craig, T., Boutilier,
C., and Mihailidis, A. (2010). Automated hand-
washing assistance for persons with dementia using
ProgressiveCo-adaptationinHuman-MachineInteraction
367