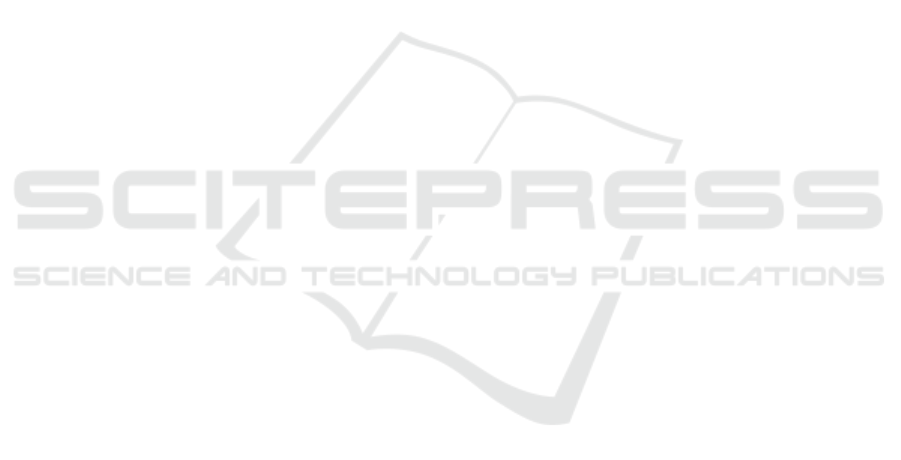
Simulation of Stochastic Activity Networks
Bajis M. Dodin
1
and Abdelghani A. Elimam
2
1
College of Business, Alfaisal University, P.O. Box 50926, Riyadh, 11533, Saudi Arabia
2
School of Science and Engineering, Mechanical Engineering Department, American University in Cairo, Cairo, Egypt
Keywords: Probabilistic Durations, Network Structure, Simulation, Sample Size, Project Variance.
Abstract: Stochastic Activity Networks (SANs) are used in modeling and managing projects that are characterized by
uncertainty. SANs are primarily managed using Monte Carlo Sampling (MCS). The accuracy of the results
obtained from MCS depends on the sample size. So far the required sample size has been determined
arbitrarily and independent of the characteristics of the SAN such as the number of activities and their
underlying distributions, number of paths, and the structure of the SAN. In this paper we show that the
accuracy of the SANs simulation results would depend on the sample size. Contrary to existing practices,
we show that such sample size must reflect the project size and structure, as well as the number of activities.
We propose an optimization-based approach to determine the project variance, which in turn is used to
determine the number of replications in SAN simulations.
1 INTRODUCTION
Activity networks (AN) are known to be useful
models for managing many real world projects. In
routine projects such as construction projects, the
time and resources required by each activity are
known with certainty. In none-routine projects, the
time or resources requirements of an activity may
be, at best, characterized by a random variable with
a given probability distribution function. Such
networks are known as stochastic activity networks
(SANs).
Many of the measures required for managing the
SAN projects are hard to calculate using analytical
methods. To illustrate the difficulty in calculating
activity or project completion times consider the
problem of calculating the probability distribution
function (pdf) of the completion time of a project
represented by the AN of Figure 1. The duration of
activity i is represented by an independent random
variable Y
i
for i = 1, 2, 3, 4, 5, each with a specified
pdf. The completion time of the project, represented
by the random variable T, is the maximum of the
duration of the three paths in SAN. Therefore,
T = max {T
1
,T
2
,T
3
} where T
j
is the duration of path j
= 1, 2, 3; or explicitly
T
1
=Y
1
+ Y
4
, T2
=Y
1
+ Y
3
+ Y
5
, and T
3
=Y
2
+ Y
5.
In general T = max
{T(k) over all k ε P} where P is
the set of all paths in SAN. Consequently, T is the
maximum over many dependent random variables.
While it is possible to calculate the exact pdf of T
for small size SANs, such as the one in Figure 1, and
for some underlying activity pdfs, it is not possible
to calculate the exact pdf of T for larger size SANs
and for various underlying activity distributions.
This difficulty led to most of the research in SANs
starting with the development of the well-known
PERT procedure, Clark (1961). The research is
focused on approximating or bounding the pdf of T
or its statistics, Adlakha and Kulkarni (1989); and
Herroelen and Leus (2005).
The difficulty in calculating pdf of T or any of
the other measures stated above has led to the use of
Monte Carlo sampling (MCS). It is assumed that as
the number of simulation runs, known as sample size
n, increases the resulting pdf of T and all of its
statistics improve in their accuracy, and eventually
converge to the exact values as n → ∞. How large n
should be to guarantee a certain level of accuracy in
the estimated measures? The Central Limit
Theorem (CLT) has been used to answer this
question. For instance, in case of the mean value for
the T, denoted by μ
T
, if μ
s
denotes the corresponding
simulated value, then the level of accuracy is
measured by the absolute difference ε = | μ
s
- μ
T
|,
where it is desired to have the difference to be ≤ ε
with a very high probability. Let α =1 - Pr(| μ
s
- μ
T
| ≤
ε), then from the CLT it is concluded that
205
M. Dodin B. and A. Elimam A..
Simulation of Stochastic Activity Networks.
DOI: 10.5220/0005561502050211
In Proceedings of the 5th International Conference on Simulation and Modeling Methodologies, Technologies and Applications (SIMULTECH-2015),
pages 205-211
ISBN: 978-989-758-120-5
Copyright
c
2015 SCITEPRESS (Science and Technology Publications, Lda.)