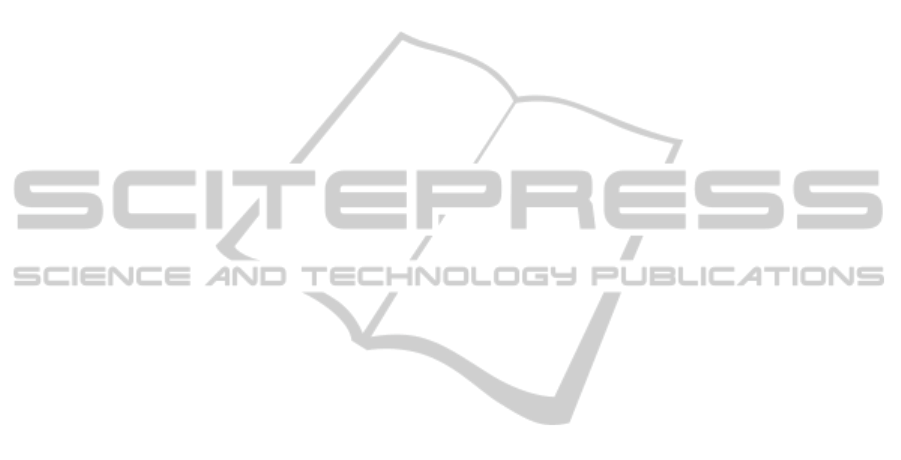
the eight body part equivalent temperatures. MLR
outperformed all other estimation techniques with re-
gard to fast processing time (of 0.23 seconds). These
two factors combined would enable a straightforward
real-time implementation in a car’s engine control
unit in comparison to the other machine learning tech-
niques evaluated.
ACKNOWLEDGEMENTS
The Low Carbon Vehicle Technology Project
(LCVTP) was a collaborative research project be-
tween leading automotive companies and research
partners, revolutionising the way vehicles are pow-
ered and manufactured. The project partners included
Jaguar Land Rover, Tata Motors European Techni-
cal Centre, Ricardo, MIRA LTD., Zytek, WMG and
Coventry University. The project included 15 au-
tomotive technology development work-streams that
will deliver technological and socio-economic out-
puts that will benefit the West Midlands Region. The
£19 million project was funded by Advantage West
Midlands (AWM) and the European Regional Devel-
opment Fund (ERDF).
The authors would like to thank the anonymous
reviewers for their insightful comments.
REFERENCES
Breiman, L. (2001). Random forests. Technical report, Uni-
versity of California Berkeley.
Cover, T. and Hart, P. (1967). Nearest neighbor pattern clas-
sification. IEEE Transactions on Information Theory,
IT-13.
Demsar, J., Curk, T., Erjavec, A., Gorup, C., Hocevar, T.,
Milutinovic, M., Mozina, M., Polajnar, M., Toplak,
M., Staric, A., Stajdohar, M., Umek, L., Zagar, L.,
Zbontar, J., Zitnik, M., and Zupan, B. (2013). Orange:
Data mining toolbox in python. Journal of Machine
Learning Research, 14:2349–2353.
Draper, N. and Smith, H. (1981). Applied Regression Anal-
ysis. Wiley.
Friedman, J. (1991). Multivariate adaptive regression
splines. Annals of Statistics, 19:1–67.
Hall, M., Frank, E., Holmes, G., Pfahringer, B., Reutemann,
P., and Witten, I. (2009). The weka data mining soft-
ware: An update. SIGKDD Explorations, 11.
Hastie, T., Tibshirani, R., and Friedman, J. (2009). The
Elements of Statistical Learning. Springer.
Haykin, S. (1998). Neural Networks: A Comprehensive
Foundation. Prentice Hall.
Hintea, D., Brusey, J., Gaura, E., Beloe, N., and Bridge, D.
(2011). Mutual information-based sensor positioning
for car cabin comfort control. In Proceedings of the
15th international conference on Knowledge-based
and intelligent information and engineering systems
- Volume Part III, KES’11, pages 483–492.
Hintea, D., Kemp, J., Brusey, J., Gaura, E., and Beloe, N.
(2014). Applicability of thermal comfort models to
car cabin environments. In International Conference
on Informatics in Control, Automation and Robotics
(ICINCO), volume 1, pages 769–776.
Quinlan, J. (1986). Introduction of decision trees. Machine
Learning, 1:81–106.
Srivastava, A., Oza, N., and Stroeve, J. (2005). Virtual sen-
sors: Using data mining techniques to efficiently es-
timate remote sensing spectra. IEEE Transactions on
Geoscience and Remote Sensing, 43.
van Rossum, G. and Drake, F. (2001). Python Reference
Manual. PythonLabs.
Way, M. and Srivastava, A. (2006). Novel methods for
predicting photometric redshifts from broadband pho-
tometry using virtual sensors. The Astrophysical Jour-
nal, 647:102–115.
Wenzel, T., Burnham, K., Blundell, M., and Williams, R.
(2007). Kalman filter as a virtual sensor: Applied to
automotive stability systems. Transactions of the In-
stitute of Measurement and Control, 29.
Witten, I. and Frank, E. (2005). Data Mining: Practi-
cal Machine Learning Tools and Techniques. Morgan
Kaufmann.
ICINCO2015-12thInternationalConferenceonInformaticsinControl,AutomationandRobotics
634