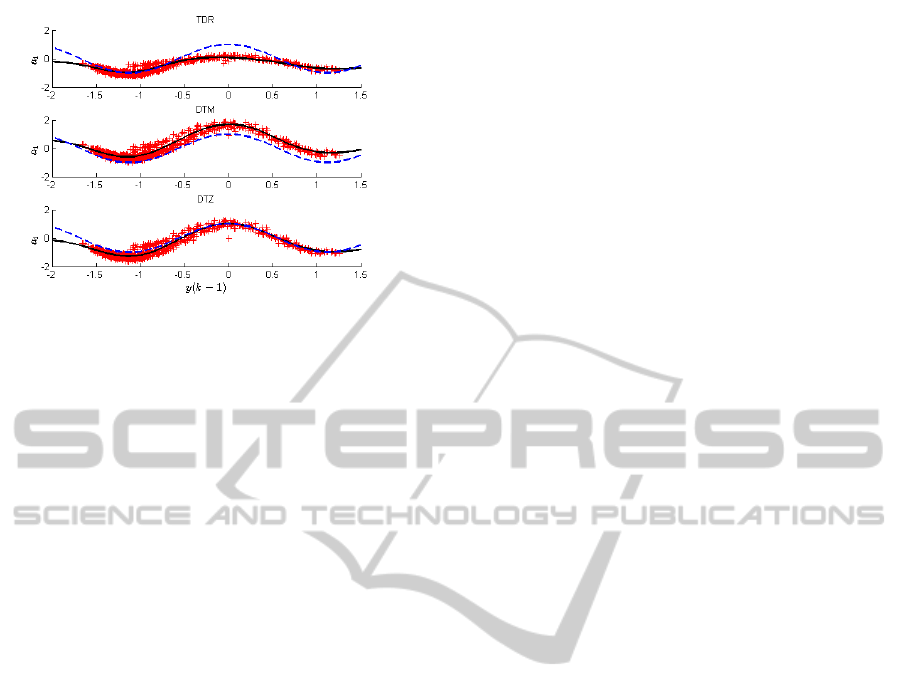
Figure 7: SVR parameterization for C = 400 and ε = 0.2
(black solid lines). The real state-parameter relationship
(blue dotted lines).
promising the good performance in the estimation of
the output.
In DTM case the state values y(k − 1) are trans-
formed to its mean, for ψ = E{X
i
(k)}. When the data
are reordered some bias appears, as we can see in
second plot in Fig. 7. The bias can be subtracted
by adding the difference between the mean of the
reordered data and the original data. Now DTM-
unbiased and DTZ methods results in similar perfor-
mances to estimate the output, see Table 1.
5 CONCLUSIONS
Parameter estimation methods based on recursive
least square are inefficient when the model presents
SDP. It is because the parameter and his respective
state vary very fast. Young shows an approximated
solution based on temporal data reordering and fixed
interval smoothing TDR-FIS. This TDR smooths the
state and the associated parameter. Both signals, state
and parameter, are assumed smoothed since the de-
pendence exists. Numerical example shows that TDR
is efficient only with an optimal FIS algorithm instead
of an SFIS, see Fig. 3 and Fig. 4. Unlike the TDR,
our two proposed transformation brings the data to a
constant value. The first brings the state to the mean
and the other to zero. These methods are called DTM
and DTZ respectively. The supposed constant param-
eter in the transformed space allow the use of SFIS
algorithm instead of an optimal FIS.
Numerical example shows that DTM and DTZ
both with SFIS estimation algorithm are equally effi-
cient to detect dependence on the SDP. SVR methods
was employed to parametrize and to obtain an error
measure for each transformation case. Based on the
result of Table 1, we can conclude that DTM-SFIS
and DTZ-SFIS are equally efficient to detect depen-
dence among state and parameter and both are better
than the estimation using TDR-SFIS. Based on nu-
merical complexity or computational cost, we also
can conclude that the DTZ is better than DTM be-
cause a state is converted to zero and it simplifies a
lot the algorithm. A final and more accurate model
structure was obtained using parametrization based
on SVR on the equation 18. Although our results
are goods, other examples to demonstrate our prac-
tical proposal are necessary, e.g. implement our algo-
rithm in models with exogenous inputs or multi-state
parameter dependency.
ACKNOWLEDGEMENTS
We thank Brazilian agency CAPES for the financial
support.
REFERENCES
Alegria, E. J. (2015). Off-line state-dependent parame-
ter models identification using simple fixed interval
smoothing. In 12th International Conference on Infor-
matics in Control, Automation and Robotics ICINCO.
Dolby, J. L. (1963). A quick method for choosing a trans-
formation. Technometrics, 5:317–325.
Hu, J., Kumamura, K., and Hirasawa, K. (2001). A quasi-
ARMAX approach to modeling of nonlinear systems.
International Journal of Control, 74:1754–1766.
Nørgaard, M., Ravn, O., Poulsen, N. K., and Hansen, L. K.
(2000). Neural Networks for Modelling and Control
of Dynamic Systems. Springer Verlag, London.
Priestley, M. (1988). Non-linear and non-stationary time
series analysis. Academic Press, London.
Sch
¨
olkopf, B. and Smola, A. J. (2001). Learning with Ker-
nels: Support Vector Machines, Regularization, Opti-
mization, and Beyond. MIT Press, Cambridge, MA,
USA.
Smola, A. J. and Sch
¨
olkopf, B. (2004). A tutorial on support
vector regression. Statistics and Computing, 14:199–
222.
Taylor, C., Pedregal, D., Young, P., and Tych, W. (2007).
Environmental time series analysis and forecasting
with the captain toolbox. Environmental Modelling
& Software, 22(6):797–814.
Vapnik, V. N. (1995). The nature of statistical learning the-
ory. Springer-Verlag, New York.
Young, P. (2006). Nonlinear system modeling based on non-
parametric identification and linear wavelet stimation
of sdp models. 45th IEEE Conference on Decision &
Control, pages 2523–2528.
Young, P. C. (2011). Recursive Estimation and Time-Series
Analysis. Springer-Verlag, 2 edition.
State-parameterDependencyEstimationofStochasticTimeSeriesusingDataTransformationandParameterizationby
SupportVectorRegression
347