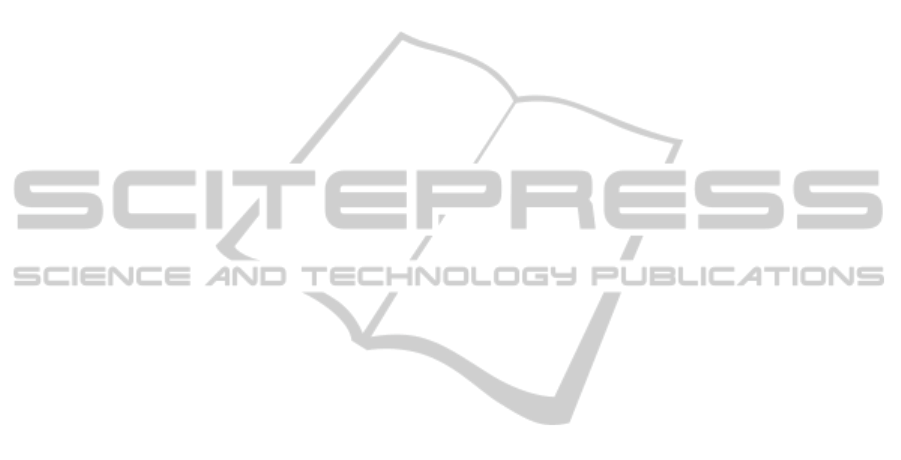
5 CONCLUSIONS
Various classification approaches are used in a vari-
ety of contexts. Some of these contexts involve time-
constraints where the run-time characteristics of the
classification approach becomes especially relevant.
In this work we have studied the higher level task
of detecting a rare video with relevant characteristics
among a large set of irrelevant videos. For such tasks
the run time characteristics can be expected to have
high importance in addition to the classification per-
formance.
In this study Monte-Carlo simulations were per-
formed to evaluate the task completion time as re-
lated to the run-time and classification performances
of six different video classification approaches. Per-
formance using cascading classifiers were also exam-
ined. The presented study reflects only a subset of the
available approaches and has some simplifications. It
nevertheless provides strong support for the statement
that classification performance cannot be the only fac-
tor in considerationwhen designing classification sys-
tems used in time constrained environments. The
results show that high classification performance in
terms of true positive and false positive rates not nec-
essarily lead to high task performance.
ACKNOWLEDGEMENTS
The authors wish to thank Soonhung Jung for sharing
data on the receiver operating conditions from their
study.
REFERENCES
Ameigeiras, P., Ramos-Munoz, J. J., Navarro-Ortiz, J., and
Lopez-Soler, J. M. (2012). Analysis and modelling of
youtube traffic. Transactions on Emerging Telecom-
munications Technologies, 23(4):360–377.
Behrad, A., Salehpour, M., Ghaderian, M., Saiedi, M.,
and Barati, M. N. (2012). Content-based obscene
video recognition by combining 3d spatiotemporal
and motion-based features. EURASIP Journal on Im-
age and Video Processing, 2012(1):1–17.
Briassouli, A. and Ahuja, N. (2007). Extraction and
analysis of multiple periodic motions in video se-
quences. Pattern Analysis and Machine Intelligence,
IEEE Transactions on, 29(7):1244–1261.
Carlsson, N., Dán, G., Mahanti, A., and Arlitt, M. (2012).
A longitudinal characterization of local and global bit-
torrent workload dynamics. In Passive and Active
Measurement, pages 252–262. Springer.
de Castro Polastro, M. and da Silva Eleuterio, P. M. (2012).
A statistical approach for identifying videos of child
pornography at crime scenes. In Availability, Reliabil-
ity and Security (ARES), 2012 Seventh International
Conference on, pages 604–612. IEEE.
Endeshaw, T., Garcia, J., and Jakobsson, A. (2008). Clas-
sification of indecent videos by low complexity repet-
itive motion detection. In Applied Imagery Pattern
Recognition Workshop, 2008. AIPR ’08. 37th IEEE,
pages 1–7.
Garcia, J. (2014). An evaluation of side-information as-
sisted forensic hash matching. In Computer Software
and Applications Conference Workshops (COMP-
SACW), 2014 IEEE 38th International, pages 331–
336.
Jung, S., Youn, J., and Sull, S. (2014). A real-time system
for detecting indecent videos based on spatiotemporal
patterns. Consumer Electronics, IEEE Transactions
on, 60(4):696–701.
Liu, F. and Picard, R. W. (1998). Finding periodicity in
space and time. In Computer Vision, 1998. Sixth In-
ternational Conference on, pages 376–383. IEEE.
Niyogi, S. A. and Adelson, E. H. (1994). Analyzing gait
with spatiotemporal surfaces. In Motion of Non-Rigid
and Articulated Objects, 1994., Proceedings of the
1994 IEEE Workshop on, pages 64–69. IEEE.
Schulze, C., Henter, D., Borth, D., and Dengel, A. (2014).
Automatic detection of csa media by multi-modal fea-
ture fusion for law enforcement support. In Pro-
ceedings of International Conference on Multimedia
Retrieval, ICMR ’14, pages 353:353–353:360, New
York, NY, USA. ACM.
Ulges, A., Schulze, C., Borth, D., and Stahl, A. (2012).
Pornography detection in video benefits (a lot) from
a multi-modal approach. In Proceedings of the 2012
ACM international workshop on Audio and multime-
dia methods for large-scale video analysis, pages 21–
26. ACM.
Viola, P. and Jones, M. (2001). Rapid object detection using
a boosted cascade of simple features. In Computer Vi-
sion and Pattern Recognition, 2001. CVPR 2001. Pro-
ceedings of the 2001 IEEE Computer Society Confer-
ence on, volume 1, pages I–511–I–518 vol.1.
SECRYPT2015-InternationalConferenceonSecurityandCryptography
426