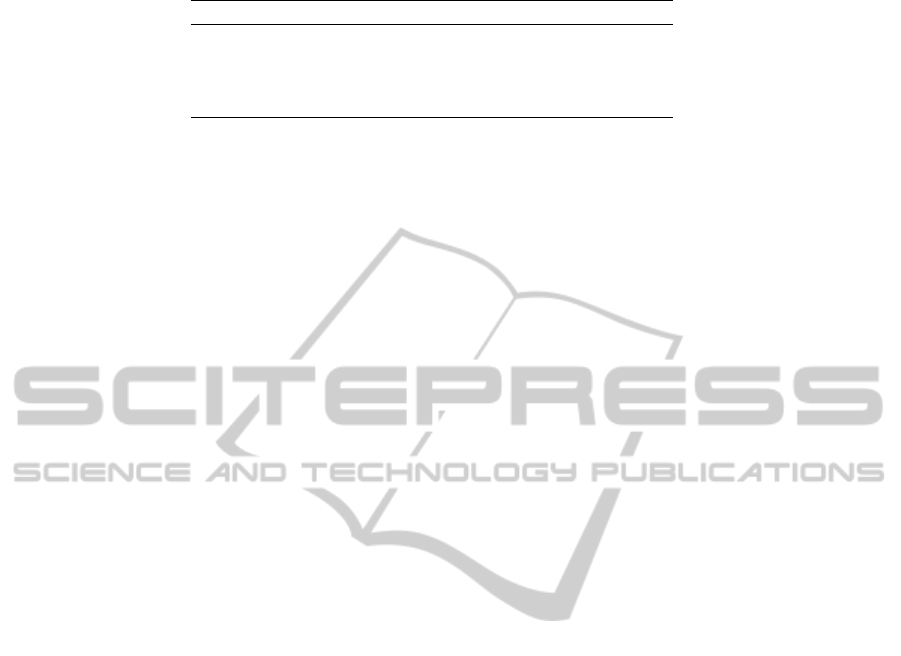
Table 1: Results of the clustering for K = 4 for the first two sections of the exhibition.
Animals Cluster0 Cluster1 Cluster2 Cluster3
A 14 11 4 22
B 4 0 1 27
F 7 72 4 2
G 16 9 30 30
columns) and sections 1 − 7 (blue columns) of the
cultural heritage event. For the first 2 sections, we ob-
serve that only the ∼ 7.5% of the users are As, while
the ∼ 12.5% are classified as Bs. Moreover, the users
classified as Fs and Gs are the same amount of the
∼ 33%. The remaining ∼ 12.5% of users are not clas-
sified. Furthermore, from the section 1 to the section
4 we note that all the users become Fs (∼ 63%) or
Gs (∼ 37%). Finally, by observing the blue columns
of the histogram in Figure 3, from the section 1 to the
section 7 (i.e., the entire exhibition) we note that there
is an adjustment of these metamorphosis of the users
in F (∼ 69.5%) and G (∼ 30.5%).
From these experiments we deduce that as the
time spent in the exhibit grows, visitors choose to not
use the available technology in an intensive way. We
deduce that if the event had lasted only 2 sections,
54% of users would continue to use the supplied tech-
nology, instead of the 30.5% on the entire exhibition.
5 CONCLUSIONS
In this paper we have described a framework that re-
flects the computational methodology adopted to infer
information about visitors in a cultural heritage con-
text. Our challenge is to match, in a realistic way, the
biological morphology of a neuron and its behaviour
in this application scenario. In the model we propose,
the (R, C) couple represents the sensitivity of an user
respect to an artwork. Accordingly, we compared two
different strategies for tuning model parameters in or-
der to find an accurate approach that is able to pro-
vide the best setting for the neuronal model. In this
respect, we shown experimental results for standard
Bayesian classifier and a novel clustering methodol-
ogy to obtain starting groups from which these elec-
trical parameters can be tuned. From our experiments,
it has been highlighted that clustering task is able to
produce a more accurate setting.
Starting from the state-of-art about the Museum
visitors’ behaviour patterns, we have investigated how
the use of technological tools within cultural spaces
can affect visitors’ behaviour, causing behavioural
changes also during the same visit. In this particular
case, we have analysed such behaviour modification,
introducing the concept of metamorphosis and show-
ing the analysis results in visitors’ styles.
An interesting observation and challenge for fu-
ture works is to adapt, in a smart way, this computa-
tional framework to many different application topics,
such as the context-aware profiling, feedback based
and/or recommendation systems.
ACKNOWLEDGEMENTS
Authors thank DATABENC, a High Technology Dis-
trict for Cultural Heritage management of Regione
Campania (Italy), and ENEA Portici Research Center,
UTICT-HPC Department, for supporting the paper.
REFERENCES
Bracco, G., Podda, S., Migliori, S., D’Angelo, P., Quintil-
iani, A., Giammattei, D., De Rosa, M., Pierattini, S.,
Furini, G., Guadagni, R., Simoni, F., Perozziello, A.,
De Gaetano, A., Pecoraro, S., Santoro, A., Scio’, C.,
Rocchi, A., Funel, A., Raia, S., Aprea, G., Ferrara, U.,
Novi, D., and Guarnieri, G. (2009). CRESCO HPC
System Integrated into ENEA-GRID Environment. In
Proc. of the Final Workshop of the Grid Projects of
the Italian National Operational Program 2000-2006
– Call 1575, pages 151–155.
Cuomo, S., De Michele, P., and M., P. (2014a). A biologi-
cally inspired model for describing the user behaviors
in a cultural heritage environment. In Proceedings
- Advanced Database Systems (SEBD), 22nd Italian
Symposium on, pages 292–302.
Cuomo, S., De Michele, P., Ponti, G., and M., P. (2014b).
A clustering-based approach for a finest biological
model generation describing visitor behaviours in a
cultural heritage scenario. In Proceedings - Data
Management Technologies and Applications (DATA),
3rd International Conference on, pages 427–433.
Jain, A. and Dubes, R. (1988). Algorithms for Clustering
Data. Prentice-Hall.
Kleinberg, J. (2008). The convergence of social and tech-
nological networks. Commun. ACM, 51(11):66–72.
Kumar, R., Novak, J., and Tomkins, A. (2010). Link Min-
ing: Models, Algorithms, and Applications, chapter
Structure and Evolution of Online Social Network,
pages 337–357. J. Am. Soc. Inf. Sci. Technol.
DATA2015-4thInternationalConferenceonDataManagementTechnologiesandApplications
342