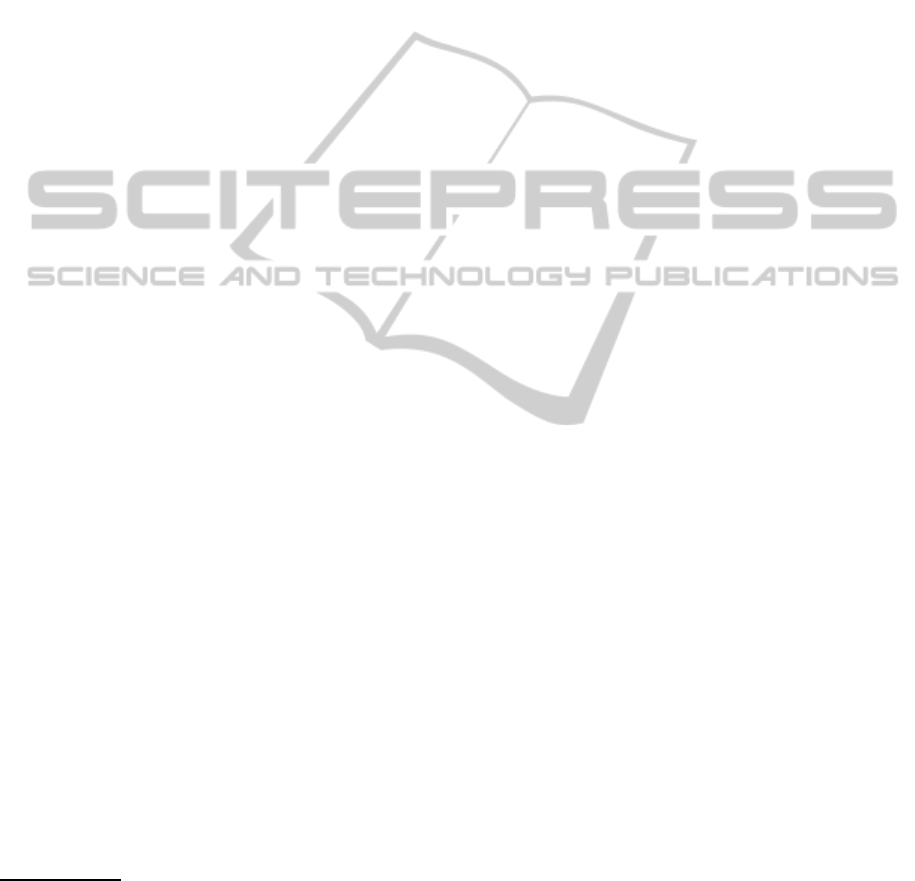
of AHP would represent an added value for classical
Business Intelligence and Analytics applications, as it
sheds the light on the dynamics that form the decision
making process (i.e., the criteria and their relative im-
portance for decisor makers). This result is quite im-
portant as in many real-life domains indicators and
analytics are not sufficient to exhaustively explain the
phenomena under study. To give an example, a vari-
ant of AHP (i.e., ANP
1
) has been applied for select-
ing knowledge management strategies of a company
whose administrators were unable to decide how to
invest the year-profits, even though a lot of indica-
tors and forecasting analyses were available, due to
the personal preferences and beliefs of each on how
to invest profits. Here the ANP was helpful to guide
them in understanding and highlighting the dynam-
ics of the decisions, first by selecting criteria, then by
evaluating each criterion and finally by summarising
a common decision (see, e.g.(Wu and Lee, 2007)).
In this paper the AHP has been applied to
three distinct groups of stakeholders, namely service
providers, over 35, and under 35 users for obtaining
the weighted criteria that would rank the enabling fac-
tors for innovating a smart-mobility service in Mi-
lan. To this end, we have built an AHP model on
the basis of the service innovation enabling factors
emerged at the CityTech meeting.
2
Then, we em-
ployed a number of stakeholders for weighting these
criteria. The main outcome of this work is a weighted
hierarchy of criteria that hold all the knowledge about
this group of stakeholders - in a formal, deterministic
and replicable way - regardless of the employed mo-
bility solutions. Then, a domain analyst may exploit
the weighted hierarchy of criteria to draw up a rank
of the best mobility solutions in Milan, according to
stakeholders weighted criteria.
The paper is arranged as follows. In Sec. 2 we
briefly introduce some backgrounds on AHP tech-
nique and its strong/weak points with respect to our
purposes. In Sec. 3 we describe the AHP model built
in terms of criteria and sub-criteria while in Sec. 4 we
show the results obtained. Finally, the conclusions are
outlined in Sec. 5
1
the Analytic Network Process differs from AHP as
(1) the model is arranged as a network rather than a tree,
thus (2) it allows expressing dependences between criteria
and sub-criteria whilst independence amongst criteria is re-
quired in the AHP model
2
An international forum held in Milan, October 27th,
2014, see http://www.citytech.eu/
2 BACKGROUNDS ON AHP
Roughly speaking, Multi Criteria Decision Making
(MCDM) refers to a set of methods that allows con-
structing a global preference relation for a set of al-
ternatives to be evaluated by using several criteria. A
literature review on MCDM falls out the scope of this
paper, the reader can refer to (Figueira et al., 2005a)
for a survey.
The MDCM approaches are able to deal with
dependence amongst criteria (ANP, see e.g., (Saaty,
2004)), conflicting criteria (ELECTRE, see e.g.,
(Figueira et al., 2005b)), to synthesise compromise
solutions (TOPSIS, see e.g., (Yoon and Hwang, 1995;
Tadi´c et al., 2014)), as well as to deal with uncertainty
over the judgments (Fuzzy sets theory applied to the
previous methods, see, e.g., (Chen et al., 1992)). Even
though the AHP is one of the most widely MCDM
method used in the literature, we employed it as it
is particularly useful for evaluating complex multi-
attribute alternatives involving subjective criteria to
capture stakeholders’ knowledge of phenomena un-
der study. Basically, AHP consists of the following
main steps.
Build up the Criteria/Alternatives Tree. In this
step the analyst identifies the criteria that com-
pose the decision problem and structure them in a
hierarchical fashion, so that a criterion may have
sub-criteria, and so on. The leafs of this tree are
the alternatives that the decision process aims at
selecting.
Pairwise Comparison. In this step the stakeholders
are required to perform a pairwise comparison of
each criterion at each level of the hierarchy, and
the results are collected in a matrix summarizing
the local priorities for each domain-expert. The
main intuition here is that it is easier (and more
accurate) to compare the importance of two crite-
ria at a time than simultaneously evaluating all of
them. AHP uses the Saaty’s 1 to 9 scale to per-
form the pairwise comparison, the scale ranges
from equal important (1) to extreme important (9)
and allows expressing the intensity of importance
of a criterion over another. It is worth noting two
relevant characteristics of AHP. First, the same
preference scale is used to perform the evaluation
of both (quantitative and qualitative) criteria and
alternatives. Second, the expert does not provide
any numerical absolute judgement; instead a com-
parative evaluation is used, which is more familiar
to people.
Comparisons are recorded in a positive reciprocal
matrix, in which a
ij
represents the comparison be-
tween element i and j.
ApplyingtheAHPtoSmartMobilityServices:ACaseStudy
355