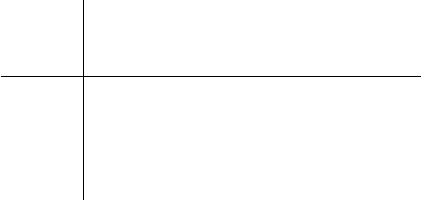
(MARE), the coefficient of determination (d) and the
coefficient of efficiency (e). The descriptive formulas
of each metric is described in Appendix A.
All the predictive approaches, including the one
proposed in this work, have their prediction error cal-
culated with respect to the target data and the results
are resumed in Table 1.
Table 1: Performance comparison by several error metrics.
Error
Metric
Opt.
Value
Gaussian
Process
Art.
Neural
Network
Inflation
NRMSE 0 0, 44833 0, 46320 0, 56246
MARE 0 0, 14830 0, 31021 0, 23222
d 1 0, 82107 0, 89463 0, 92603
e 1 0, 78072 0, 7659 0, 67730
It is important to notice that the overall error in
the Gaussian process prediction showed in Table 1
is mainly concentrated in November. Removing this
month from the error measurements would lead to
NRMSE = 0, 22644, MARE = 0, 12524, d = 0, 94
and e = 0, 94359.
Fig. 7 shows a comparative plot among the target
data and all the predictive approaches side by side.
Finally, the Brazilian government revenue estima-
tion, published by SPU on its annual report (Secre-
taria de Patrimonio da Uni
˜
ao (SPU), 2011), projects
an amount of tax collection by SPU in 2010 of
R$ 444, 085, 000.00, whereas the total amount col-
lected that year was R$ 635, 094, 000.00 - a gross dif-
ference of 38.48% between the estimated and the ex-
ecuted amount of tax collection.
The GPR approach presented in this work, in a
yearly basis, projected a total tax collection amount of
R$ 620, 703, 197.42, resulting in a gross difference of
2.27% between the projected and executed amounts.
6.4 Classification Stage Proposals
The statistical description of the estimated variable,
natively given by Gaussian processes in the regres-
sion stage, can be used to build heuristics to classify a
predicted dataset into regular or possibly fraudulent.
Here, we propose two different heuristics that are suit-
able to fraud detection scenarios. However, given the
limited information publicly available from SPU re-
garding the dataset used in this work, the evaluation
of the proposed schemes is incomplete and deserve to
be better investigated in future studies.
The resulting regression obtained through GPR,
presented in Fig. 6, shows the variance of the esti-
mated variable as a measure of confidence by trans-
lating it into error bars. Since this confidence can be
as large or as small as we desire it to be, it is possible
to optimize a classification stage based on this infor-
mation and, hence, build a trigger where high error
bars means high probability of fraud and vice versa.
In our case, without any doubt this system would clas-
sify May (month number 5) as a possibly fraudulent
one. Despite the high uncertainty level of the predic-
tion of this month, the prediction showed to be accu-
rate when compared to the target data.
Another classification approach using the variance
information can be build simply by confronting the
predicted confidence interval with the real data, when
it becomes available. In our case, this system would
classify November (month number 11) as a possibly
fraudulent one. SPU’s annual report (Secretaria de
Patrimonio da Uni
˜
ao (SPU), 2011) states that an ex-
traordinary revenue of R$ 73, 759, 533.99 happened
in 2010, but it is not possible to precise in which
month it happened. In november, the difference be-
tween the predicted value and the actual revenue was
R$ 55, 015, 235.13.
Whereas the first proposed system returns the
classified data in advance, together with the predicted
values in the regression stage, the second system
needs the real revenue data in order to classify it. On
the other hand, the second approach seeks for sam-
ples that are most dissimilar from the norm, whereas
the first approach needs to be optimized in order to
learn the norm and distinguish anomalous behaviors.
As previously mentioned, it is not possible to eval-
uate the performance of these classification stage pro-
posals due to the limited information regarding our
dataset, but the preliminary results using the statis-
tical description of the estimated variable showed in
this section encourages further studies on this topic.
7 CONCLUSIONS
This paper presented a GPR application, aimed to
model the intrinsic characteristics of a specific finan-
cial series. A unidimensional model for the GPR’s co-
variance function was proposed, and a pre-processing
stage reshaped the original data set based on its cross-
correlation profile. That approach empowered the use
of a unidimensional GPR in a bidimensional environ-
ment by isolating high correlated months in one di-
mension and poor correlated months in another di-
mension.
Although Neural Networks are known for their
flexibilities and reliable results when used for regres-
sion of time series, GPR are a transparent environ-
ment, with a parametric covariance function and no
Gaussian Process for Regression in Business Intelligence: A Fraud Detection Application
47