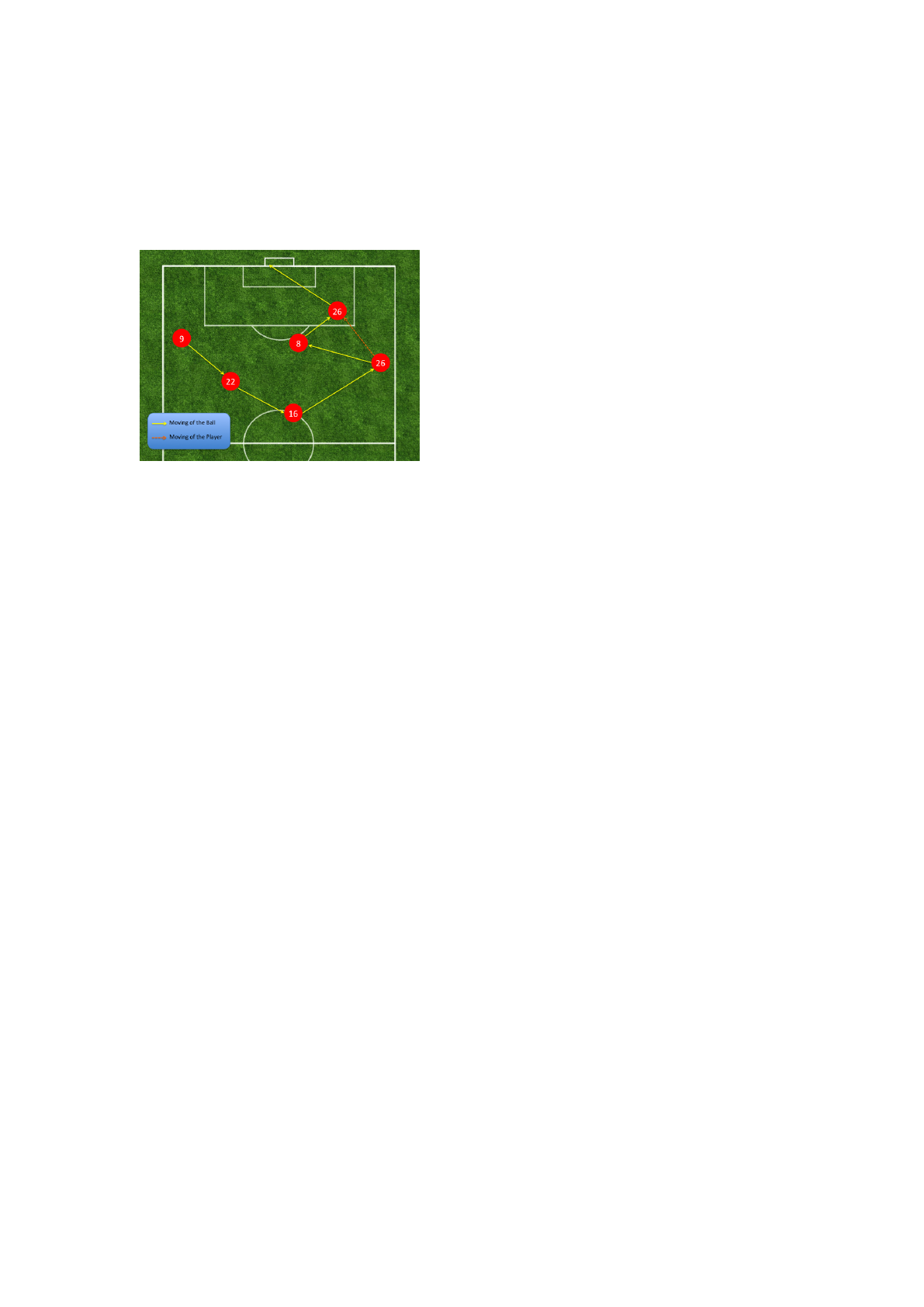
see that the green line is closer to Cluster 2, which has
a lower winning percentage than Cluster 1, although
Urawa Reds finally won the target game. This might
make us think that the result of our experiment is not
as expected.
Figure 15: Goal process in the target game.
However, there were some effective attacks by
Urawa Reds in the second half of the target game.
Figure 15 shows the process of the goal that Urawa
Reds scored. It reveals that Urawa Reds used the
pitch widely, left-side, right-side, and center, a fea-
ture of attacks in Cluster 1. The reason why this oc-
cured is possibility that the distance between the sim-
ilar games and Claster 1 was wrongly calculated to be
long according to the parameters other than the scor-
ing and the used zones. Thus, in the future work, we
will need to define a distance that gives weight to the
parameters which are the features of obtained clus-
ters.
4 CONCLUSION
Recently, data analysis in sports has been developed.
Some systems have already been used to manage and
analyze data in several fields. However, so far, no
analysis method has been applied to soccer games. In
our study, we proposed a method for predicting how
games proceed in the real time.
To this end, we collected zone data (BZD, BID,
and FD) and statistical data of past games. During the
first half of the target game, zone data were collected
and used to extract similar games from past games.
Subsequently, the extracted similar games were clas-
sified by applying a clustering technique to data of
their second halves. This is because effective features
of similar games played well must also be effective in
the target game. Finally, features in the second half
in the clusters were extracted as the parameter having
the lowest variance. The information obtained was
used to discuss the strategy by which the team should
play advantageously in the second half of the target
game.
We evaluated our method by applying it to real
match data and established some points. First, we
confirmed that the method extracted games similar
to the target game. Moreover, these similar games
had similar features of zone data even in their sec-
ond halves. This means that we correctly assumed
that games with similar features in their first halves
will proceed similarly in their second halves. We
succeeded in extracting eight games from 22 past
games similar to the target game. A clustering tech-
nique revealed the difference in winning percentage
between clusters, showing that the parameters we
chose were suitable. Finally, the feature difference
between clusters that have higher and lower winning
rates were found to be closely related to strategies
that should be taken in the second half of the target
game. Though the strategy obtained was not epoch-
making, we quantitatively demonstrated an advanta-
geous strategy that is well known to people who often
watch soccer games.
In the future, we will increase the number of past
games to ensure a variety of games. In this study,
we used only zone data and a few types of statistical
data for clustering. More types of data must be used
for further analyses. Moreover, we will also need to
define a distance that gives weight to the parameters
which are the features of obtained clusters.
ACKNOWLEDGEMENTS
I wish to thank Data Stadium Inc. for providing J-
League data used in this study.
REFERENCES
Data stadium inc. https://www.datastadium.co.jp. [Online;
accessed 26-August-2015].
Gordon S. Linoff, . M. J. B. (1999). Data Mining Tech-
niques: For Marketing, Sales, and Customer Relation-
ship Management. Kaibun-do, 1st edition.
Jo, H., Yokoo, T., Ando, K., Nishijima, N., Kumagai, S.,
Naomoto, H., Suzuki, K., Yamada, Y., Nakano, T.,
and Saito, K. (2014). The attacking indexes of play-
ers and teams in j league. In Research on Sports Data
Analysis, volume 1, pages 21 – 26. The Institute of
Statistical Mathematics.
Shigenaga, K., Nakatsu, T., Naito, T., Kata, T., Saruta, S.,
Hidaka, A., Enomoto, D., Ogura, T., and Kamakura,
M. (2014). The pass analysis in soccer based on graph
KDIR 2015 - 7th International Conference on Knowledge Discovery and Information Retrieval
224