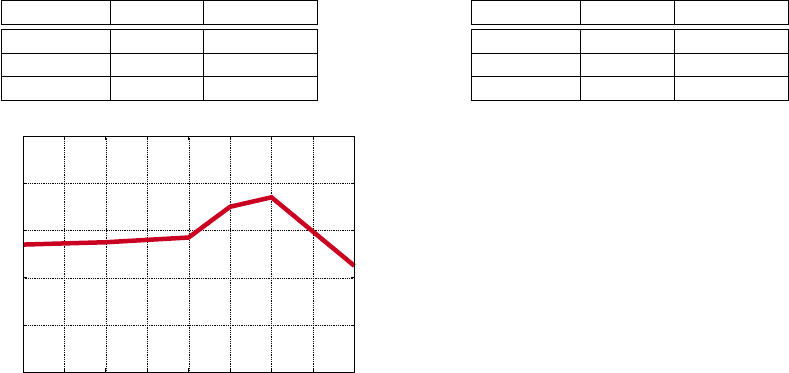
Table 2: Results of the test dataset.
Baseline ArabRelat
Precision 0.59 0.74
Recall 0.21 0.67
F-measure 0.31 0.70
0
0.2
0.4
0.6
0.8
1
0 0.1 0.2 0.3 0.4 0.5 0.6 0.7 0.8
Precision
Confidence Threshold (α)
Figure 5: Effect of confidence threshold α on the system
accuracy.
with subset of the features. We assume the baseline
features are the lexical and syntactic features. We
show the effect of adding the Arabic-specific features
on the system accuracy. Table 2 shows the results of
the system using the evaluation test set. The results
show that while the baseline maintains good preci-
sion, it bitterly decreases the recall. ArabRelat im-
proves the precision by 15% over the baseline, and
improves the recall by 46%.
6.3.2 Effect of Confidence Threshold
Figure 5 shows the effect of the confidence threshold
α on the system accuracy using the test data. As the
value of α increases, the accuracy of the system in-
creases until it reaches its optimal value at α = 0.6.
For larger values of alpha the accuracy decreases be-
cause the number of instances which survive becomes
very small, thus more prone to false positive errors.
We set the default value of α to 0.6.
6.3.3 Human Evaluation Method
Due to the lack of public gold-standard Arabic rela-
tion data, we construct another test dataset tagged by
an Arabic speaker. We extracted 100 sentences of the
test dataset, an Arabic native speaker tagged each sen-
tence to a relation type. The speaker was given each
sentence and the two entities to be tagged, with a set
of relation types. The task was to tag each sentence
with a suitable relation type or none if the sentence
does not express a relation between the two entities.
Table 3: Human Evaluation Results.
Baseline ArabRelat
Precision 0.34 0.50
Recall 0.33 0.43
F-measure 0.34 0.46
We used a subset of 18 relation types. The results are
shown in Table 3. The precision of ArabRelat system
decreases due to the small size of the dataset, how-
ever, it still outperforms the baseline.
7 CONCLUSION
In this paper, we propose a novel Relation Extrac-
tion system for the Arabic language. The system uses
distant supervised learning to build a relation classi-
fier, without the need of prior labeled data. We in-
troduce new Arabic specific features that character-
ize Arabic relations. Our experimental results on sen-
tences extracted from Wikipedia show that the system
achieves 70% overall F-measure for detecting 97 re-
lation types.
REFERENCES
Alsaif, A. and Markert, K. (2011). Modelling discourse re-
lations for arabic. In Proceedings of the Conference on
Empirical Methods in Natural Language Processing.
Association for Computational Linguistics.
Banko, M., Cafarella, M. J., Soderland, S., Broadhead, M.,
and Etzioni, O. (2007). Open information extraction
for the web. In IJCAI.
Diab, M. T., Moschitti, A., and Pighin, D. (2008). Semantic
role labeling systems for arabic using kernel methods.
In ACL.
Fan, M., Zhao, D., Zhou, Q., Liu, Z., Zheng, T. F., and
Chang, E. Y. (2014). Distant supervision for relation
extraction with matrix completion. In Proceedings of
the 52nd Annual Meeting of the Association for Com-
putational Linguistics.
Gabrilovich, E. and Markovitch, S. (2007). Computing se-
mantic relatedness using wikipedia-based explicit se-
mantic analysis. In IJCAI.
Green, S. and Manning, C. D. (2010). Better arabic pars-
ing: Baselines, evaluations, and analysis. In Proceed-
ings of the 23rd International Conference on Compu-
tational Linguistics, pages 394–402. Association for
Computational Linguistics.
Gupta, R., Halevy, A., Wang, X., Whang, S. E., and Wu, F.
(2014). Biperpedia: An ontology for search applica-
tions. Proceedings of the VLDB Endowment.
Hall, M., Frank, E., Holmes, G., Pfahringer, B., Reutemann,
P., and Witten, I. H. (2009). The weka data min-
KEOD 2015 - 7th International Conference on Knowledge Engineering and Ontology Development
416