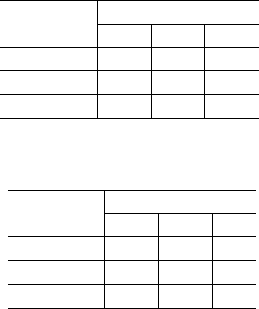
Table 2: Classification matrix for the PNB classifier.
Database
PNB
1 2 3
1 156 43 1
2 50 113 37
3 2 34 164
Table 3: Classification matrix for the NB classifier.
Database
NB
1 2 3
1 173 27 0
2 87 106 7
3 25 88 87
It is possible to see by Tables 1, 2, 3 and by Kappa
coefficients that the performance of the FPNB clas-
sifier is better than both other classifiers. In statis-
tical terms, the difference of performance between
those assessment methods can be considered signif-
icant. Observing the computational performance, the
FPNB was faster than the one based on NB, but PNB
is the fastest.
5 CONCLUSIONS
In this paper was presented a new classifier based on
Fuzzy Poisson Naive Bayes. Classifiers based on this
approach can be applied to epidemiological studies as
well as to other areas of human knowledge, as text
classification and neurosciences.
The Fuzzy Poisson Naive Bayes performance was
compared with other classifiers performance based on
Poisson Naive Bayes and Naive Bayes. The results
obtained showed that the first one presents signifi-
cant better classifications than the others. The Pois-
son Naive Bayes classifier provided competitive re-
sults and the Naive Bayes classifier provided the worst
results.
In terms of CPU time, the Fuzzy Poisson Naive
Bayes was faster than the Naive Bayes, but Poisson
Naive Bayes is the fastest. The new classifier pointed
out a competitive approach to solve problems in Epi-
demiology.
ACKNOWLEDGEMENTS
This project is partially supported by grants
310561/2012-4 and 310470/2012-9 of the National
Council for Scientific and Technological Develop-
ment (CNPq) and is related to the National Insti-
tute of Science and Technology “Medicine Assisted
by Scientific Computing”(181813/2010-6) also sup-
ported by CNPq.
REFERENCES
Altheneyan, A. S. and Menai, M. E. B. (2014). Naive bayes
classifiers for authorship attribution of arabic texts.
Journal of King Saud University Computer and In-
formation Sciences, 26(4):473–484.
Bielza, C. and Larranaga, P. (2014). Discrete bayesian net-
work classifiers: A survey. ACM Computing Surveys,
47(1):Article 5.
Bishop, C. (2007). Pattern Recognition and Machine
Learning. Springer, Berlin, 1st edition.
Cohen, J. (1960). A coefficient of agreement for nominal
scales. Educat. Psyc. Measurement, 20(1):37–46.
Congdon, C. B. (2000). Classification of epidemiological
data: a comparison of genetic algorithm and decision
tree approaches. In Proceedings of the 2000 Congress
on Evolutionary Computation, pages 442–449.
Dubois, D. and Prade, H. (1983). Unfair coins and neces-
sity measures: Towards a possibilistic interpretation
of histograms. Fuzzy Sets and Systems, 10(1-3):1520.
Duda, R. O., Hart, P. E., and Stork, D. G. (2000). Pat-
tern Classification. Wiley Interscience, New York,
2nd edition.
Feller, W. (1971). An Introduction to Probability Theory
and its Applications. Wiley, 2nd edition.
Johnson, R. A. and Wichern, D. W. (2007). Applied Multi-
variate Statistical Analysis. Pearson, 6th edition.
Kaufmann, M., Meier, A., and Stoffel, K. (2015). Ifc-
filter: Membership function generation for inductive
fuzzy classification. Expert Systems with Applica-
tions, 42:83698379.
Keller, J. M., Gray, M. R., and Givens, J. A. (1985). A
fuzzy k-nearest neighbor algoritm. IEEE Trans. Syst.
Man and Cybernetics, 15(4):580–585.
Kim, S.-B., Seo, H.-C., and Rim, H.-C. (2003). Poisson
naive bayes for text classification with feature weight-
ing. In Proceedings of the Sixth International Work-
shop on Information Retrieval with Asian Languages,
pages 33–40.
Klir, G. J. and Yuan, B. (1995). Fuzzy Sets and Fuzzy Logic:
Theory and Applications. Prentice Hall, 1st edition.
Ma, W. J., Beck, J. M., Latham, P. E., and Pouget, A.
(2006). Bayesian inference with probabilistic popu-
lation codes. Nature Neuroscience, 9(11):1432–1438.
Melo, A. C. O., Moraes, R. M., and Machado, L. S. (2003).
Gaussian mixture models for supervised classifica-
tion of remote sensing muliespectral images. Lecture
Notes in Computer Science, 2905:440–447.
Moraes, R. M. and Machado, L. S. (2012). Online assess-
ment in medical simulators based on virtual reality us-
ing fuzzy gaussian naive bayes. Journal of Multiple-
Valued Logic and Soft Computing, 18(5-6):479–492.
A Fuzzy Poisson Naive Bayes Classifier for Epidemiological Purposes
197