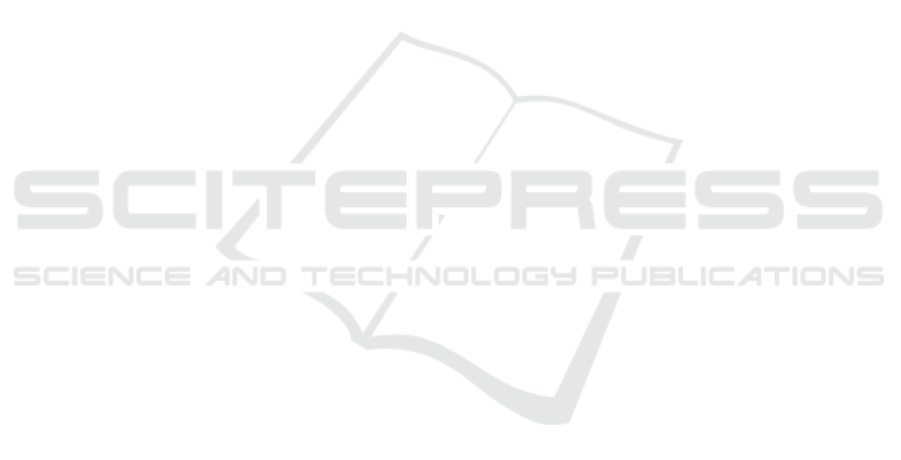
5 CONCLUSION AND REMARKS
In this work we have presented an unsupervised ob-
ject cosegmentation approach that overcomes certain
limitations that other state-of-the-art methods exhibit.
We have shown, that most of the current methods
based on MRFs or dense (exact) correspondences are
limited by the fact that they cannot leverage knowl-
edge to new images that need to be segmented.
Our approach is capable of object cosegmenta-
tion yielding state-of-the-art performance while be-
ing scalable to larger image sets and using less in-
formation to infer labels on yet unseen images. Our
results indicate that carefully choosing representative
object class clusters that account for the object class’
intrinsic variability can compensate for information
that needs to be present when cosegmentation is per-
formed over a whole image set. We do note, however,
that the current choice of the transfer set T is based
on simple assumptions about global image statistics
that might not work for images on which the common
foreground is among other objects or on very cluttered
background. Furthermore, the results are promising
and we plan to test our approach on larger image sets
incorporating dynamic updating of the transfer set T
when images are added to the set one after the other.
ACKNOWLEDGEMENTS
This research was partially funded by the project Vi-
sual Analytics in Public Health (TO 166/13-2), which
is part of the Priority Program 1335: Scalable Visual
Analytics of the German Research Foundation.
REFERENCES
Achanta, R., Shaji, A., Smith, K., Lucchi, A., Fua, P., and
S
¨
usstrunk, S. (2012). Slic superpixels compared to
state-of-the-art superpixel methods. IEEE transac-
tions on pattern analysis and machine intelligence,
34(11):2274–2282.
Arbelaez, P. (2006). Boundary extraction in natural images
using ultrametric contour maps. In 2006 Conference
on Computer Vision and Pattern Recognition Work-
shop (CVPRW’06), page 182.
Barnes, C., Shechtman, E., Finkelstein, A., and Goldman,
D. B. (2009). Patchmatch: A randomized corre-
spondence algorithm for structural image editing. In
Funkhouser, T. and Hoppe, H., editors, ACM SIG-
GRAPH 2009 papers, page 1.
Barnes, C., Shechtman, E., Goldman, D. B., and Finkel-
stein, A. (2010). The generalized patchmatch corre-
spondence algorithm. In Daniilidis, K., Maragos, P.,
and Paragios, N., editors, Computer Vision – ECCV
2010, volume 6313 of Lecture Notes in Computer
Science, pages 29–43. Springer Berlin Heidelberg,
Berlin, Heidelberg.
Batra, D., Kowdle, A., Parikh, D., Luo, J., and Chen, T.
(2010). icoseg: Interactive co-segmentation with in-
telligent scribble guidance. In 2010 IEEE Conference
on Computer Vision and Pattern Recognition (CVPR),
pages 3169–3176.
Boykov, Y. Y. and Jolly, M.-P. (2001). Interactive graph
cuts for optimal boundary & region segmentation of
objects in n-d images. In Eighth IEEE International
Conference on Computer Vision, pages 105–112.
Cheng, M.-M., Zhang, G.-X., Mitra, N. J., Huang, X., and
Hu, S.-M. (2011). Global contrast based salient re-
gion detection. In 2011 IEEE Conference on Com-
puter Vision and Pattern Recognition (CVPR), pages
409–416.
Faktor, A. and Irani, M. (2012). “clustering by composi-
tion” – unsupervised discovery of image categories.
In Daniilidis, K., Maragos, P., and Paragios, N., edi-
tors, Computer Vision – ECCV 2012, volume 7578 of
Lecture Notes in Computer Science, pages 474–487.
Springer Berlin Heidelberg, Berlin, Heidelberg.
Faktor, A. and Irani, M. (2013). Co-segmentation by com-
position. In 2013 IEEE International Conference on
Computer Vision (ICCV), pages 1297–1304.
Felzenszwalb, P. F., Girshick, R. B., McAllester, D., and
Ramanan, D. (2010). Object detection with discrim-
inatively trained part-based models. Pattern Analy-
sis and Machine Intelligence, IEEE Transactions on,
32(9):1627–1645.
Gould, S. (2012). Darwin: A framework for machine learn-
ing and computer vision research and development.
Journal of Machine Learning Research, 13(1):3533–
3537.
Gould, S. and Zhang, Y. (2012). Patchmatchgraph: Build-
ing a graph of dense patch correspondences for label
transfer. In Daniilidis, K., Maragos, P., and Paragios,
N., editors, Computer Vision – ECCV 2012, volume
7576 of Lecture Notes in Computer Science, pages
439–452. Springer Berlin Heidelberg, Berlin, Heidel-
berg.
Gould, S., Zhao, J., He, X., and Zhang, Y. (2014). Super-
pixel graph label transfer with learned distance metric.
In Fleet, D., Pajdla, T., Schiele, B., and Tuytelaars, T.,
editors, Computer Vision – ECCV 2014, volume 8689
of Lecture Notes in Computer Science, pages 632–
647. Springer International Publishing, Cham.
Hochbaum, D. S. and Singh, V. (2009). An efficient al-
gorithm for co-segmentation. In 2009 IEEE 12th In-
ternational Conference on Computer Vision (ICCV),
pages 269–276.
Joulin, A., Bach, F., and Ponce, J. (2010). Discriminative
clustering for image co-segmentation. In 2010 IEEE
Conference on Computer Vision and Pattern Recogni-
tion (CVPR), pages 1943–1950.
Joulin, A., Bach, F., and Ponce, J. (2012). Multi-class
cosegmentation. In 2012 IEEE Conference on Com-
puter Vision and Pattern Recognition (CVPR), pages
542–549.
Information Efficient Automatic Object Detection and Segmentation using Cosegmentation, Similarity based Clustering, and Graph Label
Transfer
405