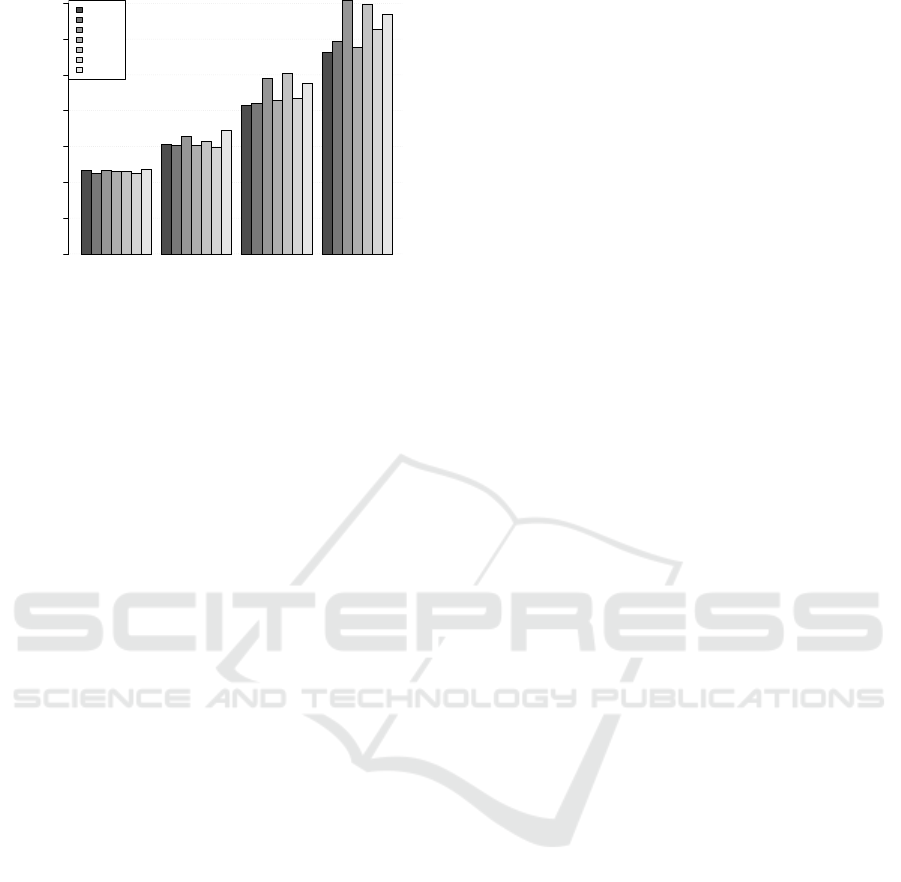
h7 h14 h28 h56
Forecast horizon (days)
sMAPE
0
10
20
30
40
50
60
70
h7 h14 h28 h56
0
10
20
30
40
50
60
70
Figure 4: Comparison of variations based on the underly-
ing time series models. sMAPE for CP1 under all forecast
horizons, 7, 14, 28 and 56 days out.
forecasting horizons. Simpler models such as HA and
ES have also shown to be consistent and performed
well, and for longer horizons they claimed top spots.
4 CONCLUSION
In this paper, we have presented 28 variations of
the pickup method. Experiments were conducted on
reservations data from four airport carparks of a major
airport in UK. The performance of each model vari-
ation was evaluated under three different error mea-
sures and for different forecast horizons, spanning
from 1 week to 8 weeks out. Our study has shown
that the best practice is to use a combination of mod-
els; perhaps a highly sophisticated model for short-
term forecasting and a simple weighted moving aver-
age for longer forecasting.
Our research aims to examine further the role of
the training set in forecast performance. More pre-
cisely, the training set can be of two types; “fixed”
for a pre-determined training size that rolls for-
ward as time progresses and updates accordingly, or
“growing” that increases with time and thus always
uses all the available data. Moreover, already under
progress is the examination of regression forecasting
models which we aim to compare against the best
pickup methods. Regression model variations relate
the total arrivals to the on-hand ones with the possibil-
ity to include other factors such as weekly seasonality,
linear trends or even flight information. Finally, we
are intrigued in exploiting the potential of combining
two or more forecasts to ultimately obtain a more ac-
curate combined forecast.
REFERENCES
Cleveland, R., Cleveland, W., McRae, J., and Terpenning,
I. (1990). Seasonal-trend decomposition procedure
based on loess. Journal of official satistics, 6(1):3–73.
Hyndman, R. and Khandakar, Y. (2007). Automatic time
series forecasting: the forecast package for r. URL
http://www. jstatsoft. org/v27/i03.
Hyndman, R., Koehler, A. B., Ord, J. K., and Snyder, R. D.
(2008). Forecasting with exponential smoothing: the
state space approach. Springer.
Hyndman, R. J., Koehler, A. B., Snyder, R. D., and Grose,
S. (2002). A state space framework for automatic fore-
casting using exponential smoothing methods. inter-
national Journal of Forecasting, 18(3):439–454.
Lee, A. O. (1990). Airline reservations forecasting; prob-
abilistic and statistical models of the booking pro-
cess. PhD thesis, Massachusetts Institute of Technol-
ogy, MIT.
Makridakis, S., Wheelwright, S. C., and Hyndman, R. J.
(2008). Forecasting methods and applications. John
Wiley & Sons.
Papayiannis, A., Johnson, P., Yumashev, D., and Duck,
P. (2013). Continuous-time revenue management in
carparks - part two: Refining the pde. In Proceed-
ings of the 2nd International Conference on Opera-
tions Research and Enterprise Systems, pages 76–81.
ICORES, SciTePress Digital Library.
Papayiannis, A., Johnson, P., Yumashev, D., Howell, S.,
Proudlove, N., and Duck, P. (2012). Continuous-
time revenue management in carparks. In Proceed-
ings of the 1st International Conference on Opera-
tions Research and Enterprise Systems, pages 73–82.
ICORES, SciTePress Digital Library.
P
¨
olt, S. (1998). Forecasting is difficult–especially if it
refers to the future. In Reservation and Yield Man-
agement Study Group Annual Meeting Proceedings,
Melbourne, Australia. AGIFORS.
Rojas, D. (2006). Revenue management techniques ap-
plied to the parking industry. Master’s thesis, School
of Industrial and Systems Engineering, University of
Florida.
Sa, J. (1987). Reservations forecasting in airline yield man-
agement. Technical report, Flight Transportation Lab-
oratory, Massachusetts Institute of Technology.
Shumway, R. H., Stoffer, D. S., and Stoffer, D. S. (2000).
Time series analysis and its applications: with R ex-
amples. Springer.
Weatherford, L. and P
¨
olt, S. (2002). Better unconstraining
of airline demand data in revenue management sys-
tems for improved forecast accuracy and greater rev-
enues. Journal of Revenue and Pricing Management,
1:234–254.
Weatherford, L. R. and Kimes, S. E. (2003). A comparison
of forecasting methods for hotel revenue management.
International Journal of Forecasting, 19(3):401–415.
Weatherford, L. R., Kimes, S. E., and Scott, D. A. (2001).
Forecasting for hotel revenue management: Testing
aggregation against disaggregation. The Cornell Ho-
A Comparative Analysis of Pickup Forecasting Methods for Customer Arrivals in Airport Carparks
23