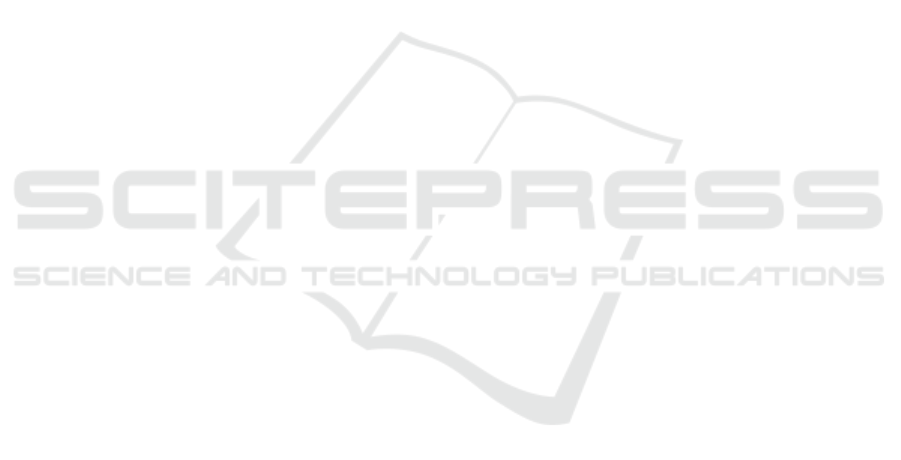
with the whole hand and grasping with two fingers
could be distinguished, e.g. for grasp planning for
robotic arms. Additionally, fine-grained affordances
for grasping actions can include drawers and doors
that can be pulled open or pulled open while rotat-
ing (about the hinge). We are currently looking for
more examples for fine-grained affordances for differ-
ent agents, to generalize our approach of fine-grained
affordances.
REFERENCES
Bar-Aviv, E. and Rivlin, E. (2006). Functional 3d object
classification using simulation of embodied agent. In
BMVC, pages 307–316.
Castellini, C., Tommasi, T., Noceti, N., Odone, F., and Ca-
puto, B. (2011). Using object affordances to improve
object recognition. Autonomous Mental Development,
IEEE Transactions on, 3(3):207–215.
Chemero, A. and Turvey, M. T. (2007). Gibsonian affor-
dances for roboticists. Adaptive Behavior, 15(4):473–
480.
Gibson, J. J. (1986). The ecological approach to visual per-
ception. Routledge.
Grabner, H., Gall, J., and Van Gool, L. (2011). What
makes a chair a chair? In Computer Vision and Pat-
tern Recognition (CVPR), 2011 IEEE Conference on,
pages 1529–1536.
Hermans, T., Rehg, J. M., and Bobick, A. (2011). Affor-
dance prediction via learned object attributes. In In-
ternational Conference on Robotics and Automation:
Workshop on Semantic Perception, Mapping, and Ex-
ploration.
Hinkle, L. and Olson, E. (2013). Predicting object func-
tionality using physical simulations. In Intelligent
Robots and Systems (IROS), 2013 IEEE/RSJ Interna-
tional Conference on, pages 2784–2790. IEEE.
Hornung, A., Wurm, K. M., Bennewitz, M., Stachniss,
C., and Burgard, W. (2013). OctoMap: An effi-
cient probabilistic 3D mapping framework based on
octrees. Autonomous Robots. Software available at
http://octomap.github.com.
Jiang, Y. and Saxena, A. (2013). Hallucinating humans for
learning robotic placement of objects. In Experimen-
tal Robotics, pages 921–937. Springer.
Kjellstr
¨
om, H., Romero, J., and Kragi
´
c, D. (2011). Vi-
sual object-action recognition: Inferring object affor-
dances from human demonstration. Computer Vision
and Image Understanding, 115(1):81–90.
Lopes, M., Melo, F. S., and Montesano, L. (2007).
Affordance-based imitation learning in robots. In
Intelligent Robots and Systems, 2007. IROS 2007.
IEEE/RSJ International Conference on, pages 1015–
1021. IEEE.
Maier, J. R., Ezhilan, T., and Fadel, G. M. (2007). The af-
fordance structure matrix: a concept exploration and
attention directing tool for affordance based design. In
ASME 2007 International Design Engineering Tech-
nical Conferences and Computers and Information in
Engineering Conference, pages 277–287. American
Society of Mechanical Engineers.
Maier, J. R., Mocko, G., Fadel, G. M., et al. (2009). Hierar-
chical affordance modeling. In DS 58-5: Proceedings
of ICED 09, the 17th International Conference on En-
gineering Design, Vol. 5, Design Methods and Tools
(pt. 1), Palo Alto, CA, USA, 24.-27.08. 2009.
Montesano, L., Lopes, M., Bernardino, A., and Santos-
Victor, J. (2008). Learning object affordances: from
sensory–motor coordination to imitation. Robotics,
IEEE Transactions on, 24(1):15–26.
Pan, J., Chitta, S., and Manocha, D. (2012). Fcl: A general
purpose library for collision and proximity queries. In
Robotics and Automation (ICRA), 2012 IEEE Interna-
tional Conference on, pages 3859–3866.
Ridge, B., Skocaj, D., and Leonardis, A. (2009). Unsu-
pervised learning of basic object affordances from ob-
ject properties. In Computer Vision Winter Workshop,
pages 21–28.
S¸ahin, E., C¸ akmak, M., Do
˘
gar, M. R., U
˘
gur, E., and
¨
Uc¸oluk,
G. (2007). To afford or not to afford: A new formal-
ization of affordances toward affordance-based robot
control. Adaptive Behavior, 15(4):447–472.
Seib, V., Wojke, N., Knauf, M., and Paulus, D. (2015). De-
tecting fine-grained affordances with an anthropomor-
phic agent model. In Fleet, D., Pajdla, T., Schiele, B.,
and Tuytelaars, T., editors, Computer Vision - ECCV
2014 Workshops, volume II of LNCS, pages 413–419.
Springer International Publishing Switzerland.
Stark, M., Lies, P., Zillich, M., Wyatt, J., and Schiele, B.
(2008). Functional object class detection based on
learned affordance cues. In Computer Vision Systems,
pages 435–444. Springer.
Sun, J., Moore, J. L., Bobick, A., and Rehg, J. M. (2010).
Learning visual object categories for robot affordance
prediction. The International Journal of Robotics Re-
search, 29(2-3):174–197.
W
¨
unstel, M. and Moratz, R. (2004). Automatic object
recognition within an office environment. In CRV, vol-
ume 4, pages 104–109. Citeseer.
Zadeh, L. A. (1965). Fuzzy sets. Information and control,
8(3):338–353.
VISAPP 2016 - International Conference on Computer Vision Theory and Applications
298