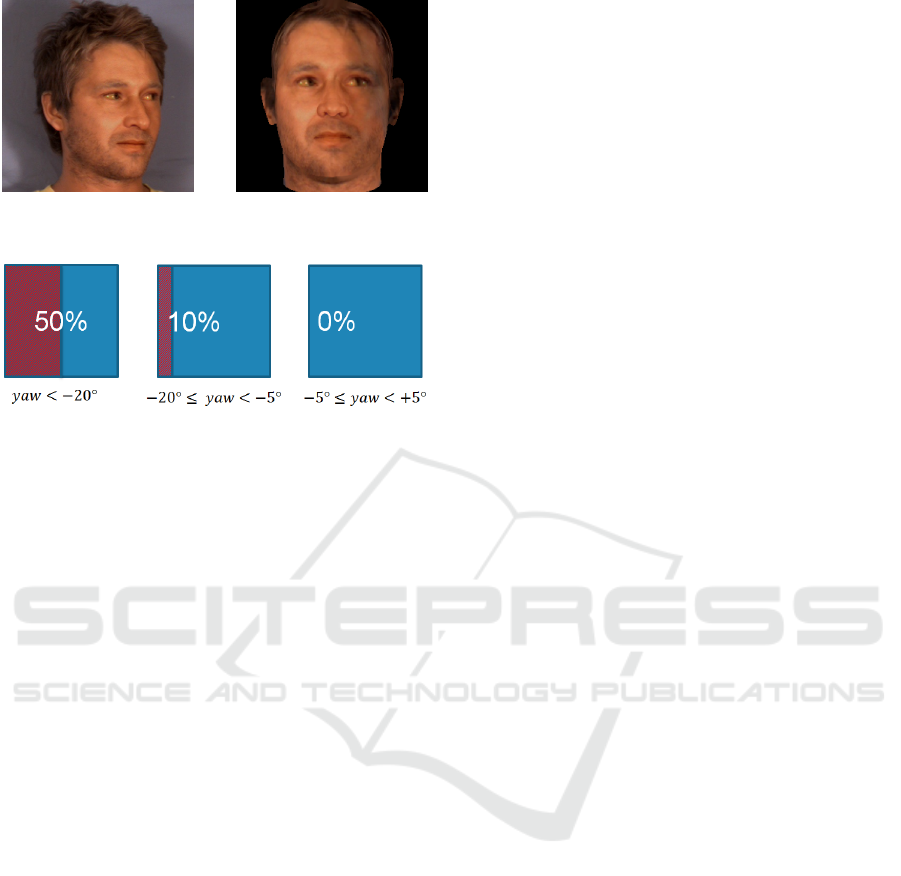
Figure 5: Original (left) and frontalized version (right) of
an image from MUCT.
Figure 6: Masks associated with 3 of the 5 bins of yaw an-
gle. The proportions of discarded pixels (hatched areas) are
written in white.
comparison process because they are poorly visible
on the original non-frontal image. These masks are
transformed into uncertainty matrices on the feature
vectors and are provided to our methods exactly as
explained in Section 5.2.2 for random occlusions.
We use the FRGC images to learn the parameters
of our model (µ, W , S
µ
and S
w
) and test our similar-
ity function on two face datasets with large variations
in pose: PUT (9971 images) and MUCT (3755 im-
ages). We compare our method to standard PCA +
Joint Bayesian by looking at the FNR for a FPR of
0.1%. On PUT, our method obtains a FNR of 2.7%
whereas the baseline achieves 3.1%. On MUCT, the
FNR are respectively 3.4% and 3.6%. First, we re-
mark that error rates on databases with pose variations
are not much higher than those we report on FRGC in
the previous section. This is due to the frontalization
scheme used in this section, FNR are much higher if
comparisons are performed on the original images.
Second, we observe that using an uncertainty-aware
similarity function leads to a notable improvement in
performance on both databases despite the simple and
coarse correspondence between yaw angles and pixel
masks we use.
6 CONCLUSION
In this paper, we have introduced a novel similarity
learning method which, unlike previous approaches,
can take advantage of uncertainty information made
available by the feature extraction process. The two
stages of our method are based on probabilistic mod-
els and we provided EM algorithms to estimate their
parameters.
Our experimental results show the benefit of ex-
plicitly accounting for uncertainty information in sim-
ilarity function learning. We demonstrate the effec-
tiveness of our method on various challenging tasks
such as dealing with images of various resolutions,
pose variations or occlusion.
The main limitation of our work is that our method
requires to be provided uncertainty information about
the data. An interesting direction for future research
is to automatize this task. This could be achieved by
designing a method to make the link between some
image quality measures (for example, local signal-to-
noise ratio at the pixel level) and the data uncertainty
matrices on extracted features.
REFERENCES
Belhumeur, P. N., ao P. Hespanha, J., and Kriegman, D. J.
(1997). Eigenfaces vs. fisherfaces: Recognition us-
ing class specific linear projection. IEEE Transactions
on Pattern Analysis and Machine Intelligence, pages
711–720.
Bi, J. and Zhang, T. (2004). Support vector classification
with input data uncertainty. In NIPS, pages 1651–
1659.
Blanz, V., Grother, P., Phillips, J. P., and Vetter, T. (2005).
Face recognition based on frontal views generated
from non-frontal images. In CVPR, pages 454–461.
Bohn
´
e, J., Ying, Y., Gentric, S., and Pontil, M. (2014).
Large margin local metric learning. In ECCV.
Cao, X., Wipf, D., Wen, F., and Duan, G. (2013). A practi-
cal transfer learning algorithm for face verification. In
ICCV.
Chen, D., Cao, X., Wang, L., Wen, G., and Sun, J. (2012).
Bayesian face revisited: a joint formulation. In ECCV.
Cormode, G. and McGregor, A. (2008). Approximation al-
gorithms for clustering uncertain data. In PODS.
Davis, J. V., Kulis, B., Jain, P., Sra, S., and Dhillon, I. S.
(2007). Information-theoretic metric learning. In
ICML, pages 209–216.
Guillaumin, M., Verbeek, J., and Schmid, C. (2009). Is that
you? metric learning approaches for face identifica-
tion. In ICCV, pages 498–505.
Huang, G. B., Ramesh, M., Berg, T., and Learned-Miller,
E. (2007). Labeled faces in the wild: A database for
studying face recognition in unconstrained environ-
ments. Technical Report 07-49, University of Mas-
sachusetts, Amherst.
K
¨
ostinger, M., Hirzer, M., Wohlhart, P., Roth, P. M., and
Bischof, H. (2012). Large scale metric learning from
equivalence constraints. In CVPR, pages 2288–2295.
Kriegel, H.-P. and Pfeifle, M. (2005). Hierarchical density-
based clustering of uncertain data. In ICDM.
Similarity Function Learning with Data Uncertainty
139