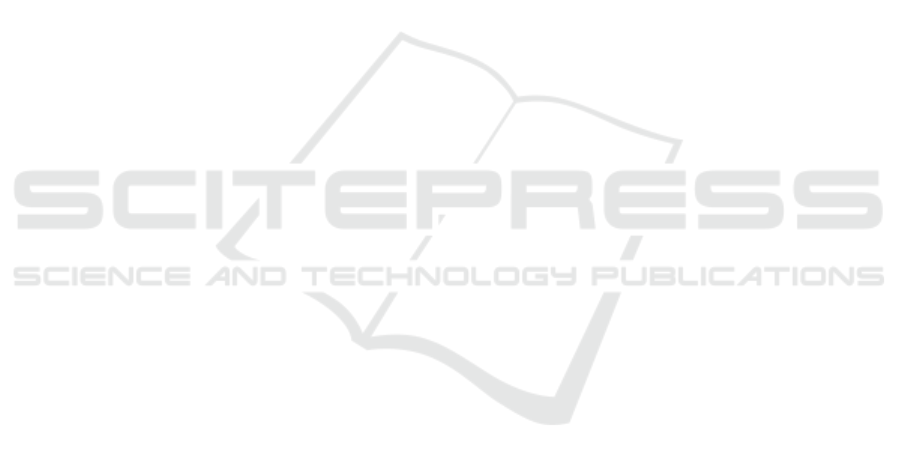
more, this effect seems to be strong for both the full
dimension of the data set and a very small subspace
dimension. The former may be related to the estima-
tion of many parameters while the latter may be due to
in insufficient subspace dimension that does not allow
for a separation of the classes.
In addition to the gain in prediction accuracy, the
runtime of the KNCM is considerably lower than the
runtime of the NCMC, as shown in Fig. 5. The de-
creasing accuracy of the NCMC suggests that the
NCMC method results in the addition of more false
labels to the training set, possibly leading to a larger
total number of samples. However, the opposite is
true. Fig. 5(d) shows the median runtime and the me-
dian of the training set size for an initial training set
comprising 1% of the total data and a dimensionality
of h = 2. This exemplary semi-supervised learning
progress is similar to the other experiments. Fig. 5(d)
clearly shows that the runtime is independent of the
training set size.
6 CONCLUSION
The proposed KNCM shows an increased accuracy
and a lower runtime in comparison to the original
NCMC. The high accuracy is maintained for ex-
tremely small dimensional subspace projections. Fur-
thermore, the KNCM and its ability to reject outliers
has been demonstrated in a semi-supervised learning
scenario of gestures. The semi-supervised KNCM
achieves accuracies that are comparable to a fully su-
pervised learning scenario. Since the proposed algo-
rithm is not limited to gesture data, it is expected to be
applicable to any semi-supervised learning scenario.
REFERENCES
Al-Behadili, H., W
¨
ohler, C., and Grumpe, A. (2014). Semi-
supervised learning of emblematic gestures. AT-
AUTOMATISIERUNGSTECHNIK, 62(10):732–739.
Al-Behadili, H., W
¨
ohler, C., and Grumpe, A. (2015). Non-
Linear Distance Based Large Scale Data Classifica-
tions. In 3’rd International conference on image In-
formation Processing (ICIIP), page In Press. IEEE.
Altman, N. S. (1992). An introduction to kernel and nearest-
neighbor nonparametric regression. The American
Statistician, 46(3):175–185.
Bhuyan, M., Bora, P., and Ghosh, D. (2008). Trajec-
tory guided recognition of hand gestures having only
global motions. International Journal of Computer
Science, Fall.
Boiman, O., Shechtman, E., and Irani, M. (2008). In de-
fense of nearest-neighbor based image classification.
In Computer Vision and Pattern Recognition, 2008.
CVPR 2008. IEEE Conference on, pages 1–8. IEEE.
Cover, T. and Hart, P. (1967). Nearest neighbor pattern clas-
sification. Information Theory, IEEE Transactions on,
13(1):21–27.
Elmezain, M., Al-Hamadi, A., Rashid, O., and Michaelis,
B. (2009). Posture and Gesture Recognition for
Human-Computer Interaction. In Jayanthakumaran,
K., editor, Advanced Technologies, pages 415–440.
InTech, Rijeka, Croatia.
Fothergill, S., Mentis, H., Kohli, P., and Nowozin, S.
(2012). Instructing people for training gestural inter-
active systems. In Proceedings of the SIGCHI Confer-
ence on Human Factors in Computing Systems, pages
1737–1746. ACM.
Guillaumin, M., Mensink, T., Verbeek, J., and Schmid, C.
(2009). Tagprop: Discriminative metric learning in
nearest neighbor models for image auto-annotation. In
Computer Vision, 2009 IEEE 12th International Con-
ference on, pages 309–316. IEEE.
Kung, S. Y. (2014). Kernel Methods and Machine Learning.
Cambridge University Press.
Mensink, T., Verbeek, J., Perronnin, F., and Csurka, G.
(2013a). Distance-based image classification: Gener-
alizing to new classes at near-zero cost. IEEE Trans-
actions on Pattern Analysis and Machine Intelligence,
35(11):2624–2637.
Mensink, T., Verbeek, J., Perronnin, F., and Csurka, G.
(2013b). Large scale metric learning for distance-
based image classification on open ended data sets. In
Advanced Topics in Computer Vision, pages 243–276.
Springer.
Richarz, J. and Fink, G. A. (2011). Visual recognition of
3d emblematic gestures in an hmm framework. Jour-
nal of Ambient Intelligence and Smart Environments,
3(3):193–211.
Schneider, P., Biehl, M., and Hammer, B. (2009). Adap-
tive relevance matrices in learning vector quantiza-
tion. Neural Computation, 21(12):3532–3561.
Theodoridis, S., Pikrakis, A., Koutroumbas, K., and
Cavouras, D. (2010). Introduction to Pattern Recog-
nition: A Matlab Approach. Academic Press.
Webb, A. R. (2003). Statistical pattern recognition. John
Wiley & Sons.
Yoon, H.-S., Soh, J., Bae, Y. J., and Yang, H. S. (2001).
Hand gesture recognition using combined features of
location, angle and velocity. Pattern recognition,
34(7):1491–1501.
Zhu, X. and Goldberg, A. B. (2009). Introduction to Semi-
supervised Learning. Synthesis lectures on artificial
intelligence and machine learning. Morgan & Clay-
pool Publishers.
VISAPP 2016 - International Conference on Computer Vision Theory and Applications
288