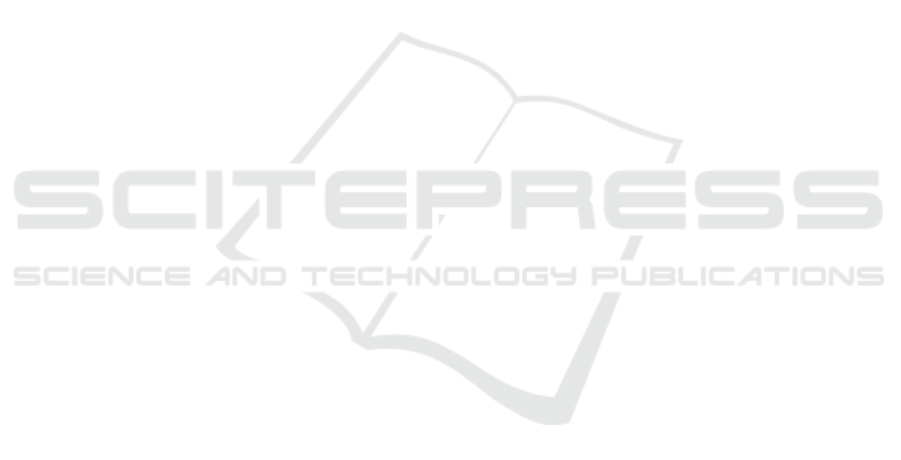
national Conference on, volume 2, pages 1395–1402
Vol. 2.
Broomhead, D. S. and Lowe, D. (1988). Multivari-
able Functional Interpolation and Adaptive Networks.
Complex Systems 2, pages 321–355.
Chen, S., Cowan, C., and Grant, P. (1991). Orthogonal
least squares learning algorithm for radial basis func-
tion networks. Neural Networks, IEEE Transactions
on, 2(2):302–309.
Efros, A., Berg, A., Mori, G., and Malik, J. (2003). Rec-
ognizing action at a distance. In Computer Vision,
2003. Proceedings. Ninth IEEE International Confer-
ence on, pages 726–733 vol.2.
Ellis, C., Masood, S. Z., Tappen, M. F., Laviola, Jr., J. J.,
and Sukthankar, R. (2013). Exploring the trade-off
between accuracy and observational latency in action
recognition. Int. J. Comput. Vision, 101(3):420–436.
Farabet, C., Couprie, C., Najman, L., and LeCun, Y. (2013).
Learning hierarchical features for scene labeling. Pat-
tern Analysis and Machine Intelligence, IEEE Trans-
actions on, 35(8):1915–1929.
Fothergill, S., Mentis, H. M., Kohli, P., and Nowozin, S.
(2012). Instructing people for training gestural inter-
active systems. In Konstan, J. A., Chi, E. H., and
H
¨
o
¨
ok, K., editors, CHI, pages 1737–1746. ACM.
Graves, A., Mohamed, A., and Hinton, G. E. (2013).
Speech recognition with deep recurrent neural net-
works. In IEEE International Conference on Acous-
tics, Speech and Signal Processing, ICASSP 2013,
Vancouver, BC, Canada, May 26-31, 2013, pages
6645–6649.
Hall, M., Frank, E., Holmes, G., Pfahringer, B., Reutemann,
P., and Witten, I. H. (2009). The weka data mining
software: An update. In SIGKDD Explorations, vol-
ume 11.
Hochreiter, S. and Schmidhuber, J. (1997). Long short-term
memory. Neural Comput., 9(8):1735–1780.
Huang, G., Huang, G.-B., Song, S., and You, K. (2015).
Trends in extreme learning machines: A review. Neu-
ral Networks, 61(0):32 – 48.
Huang, G.-B., Zhou, H., Ding, X., and Zhang, R. (2012).
Extreme learning machine for regression and mul-
ticlass classification. Systems, Man, and Cyber-
netics, Part B: Cybernetics, IEEE Transactions on,
42(2):513–529.
Huang, G.-B., Zhu, Q.-Y., and Siew, C.-K. (2004). Extreme
learning machine: a new learning scheme of feedfor-
ward neural networks. In Neural Networks, 2004. Pro-
ceedings. 2004 IEEE International Joint Conference
on, volume 2, pages 985–990 vol.2.
Hussein, M. E., Torki, M., Gowayyed, M. A., and El-Saban,
M. (2013). Human action recognition using a tempo-
ral hierarchy of covariance descriptors on 3d joint lo-
cations. In Proceedings of the Twenty-Third Interna-
tional Joint Conference on Artificial Intelligence, IJ-
CAI ’13, pages 2466–2472. AAAI Press.
Iosifidis, A., Tefas, A., and Pitas, I. (2014). Minimum
variance extreme learning machine for human action
recognition. In Acoustics, Speech and Signal Process-
ing (ICASSP), 2014 IEEE International Conference
on, pages 5427–5431.
Jain, A., Gupta, A., Rodriguez, M., and Davis, L. (2013).
Representing videos using mid-level discriminative
patches. In Computer Vision and Pattern Recogni-
tion (CVPR), 2013 IEEE Conference on, pages 2571–
2578.
Kantorov, V. and Laptev, I. (2014). Efficient feature ex-
traction, encoding, and classification for action recog-
nition. In Computer Vision and Pattern Recogni-
tion (CVPR), 2014 IEEE Conference on, pages 2593–
2600.
Lecun, Y., Bottou, L., Bengio, Y., and Haffner, P. (1998).
Gradient-based learning applied to document recogni-
tion. Proceedings of the IEEE, 86(11):2278–2324.
Lefebvre, G., Berlemont, S., Mamalet, F., and Garcia, C.
(2015). Inertial gesture recognition with BLSTM-
RNN. In Koprinkova-Hristova, P., Mladenov, V.,
and Kasabov, N. K., editors, Artificial Neural Net-
works, volume 4 of Springer Series in Bio-/Neuro-
informatics, pages 393–410. Springer International
Publishing.
Minhas, R., Baradarani, A., Seifzadeh, S., and
Jonathan Wu, Q. M. (2010). Human action recogni-
tion using extreme learning machine based on visual
vocabularies. Neurocomput., 73(10-12):1906–1917.
Seidenari, L., Varano, V., Berretti, S., Del Bimbo, A., and
Pala, P. (2013). Recognizing actions from depth cam-
eras as weakly aligned multi-part bag-of-poses. In
Computer Vision and Pattern Recognition Workshops
(CVPRW), 2013 IEEE Conference on, pages 479–485.
Tong, M. H., Bickett, A. D., Christiansen, E. M., and Cot-
trell, G. W. (2007). Learning grammatical structure
with echo state networks. Neural Networks, 20(3):424
– 432. Echo State Networks and Liquid State Ma-
chines.
Vemulapalli, R., Arrate, F., and Chellappa, R. (2014). Hu-
man action recognition by representing 3d skeletons
as points in a lie group. In Computer Vision and Pat-
tern Recognition (CVPR), 2014 IEEE Conference on,
pages 588–595.
Wang, H., Klaser, A., Schmid, C., and Liu, C.-L. (2011a).
Action recognition by dense trajectories. In Computer
Vision and Pattern Recognition (CVPR), 2011 IEEE
Conference on, pages 3169–3176.
Wang, Y., Cao, F., and Yuan, Y. (2011b). A study on effec-
tiveness of extreme learning machine. Neurocomput-
ing, 74(16):2483–2490.
Zhang, Y., Ding, S., Xu, X., Zhao, H., and Xing, W. (2013).
An algorithm research for prediction of extreme learn-
ing machines based on rough sets. Journal of Comput-
ers, 8(5).
Recognizing Human Actions based on Extreme Learning Machines
483