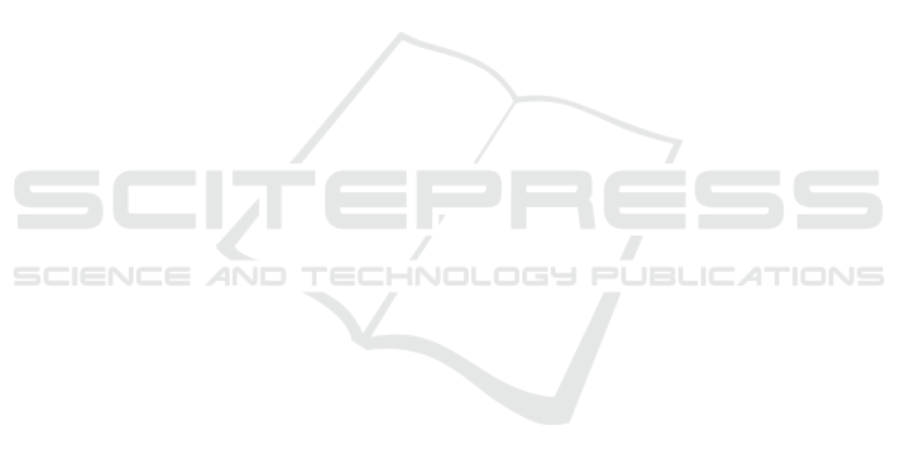
Costa, E., Pedreira, C. E., Barrena, S., Lecrevisse, Q., Flo-
res, J., Quijano, S., Almeida, J., del Carmen Garcia-
Macias, M., Bottcher, S., Van Dongen, J., et al. (2010).
Automated pattern-guided principal component anal-
ysis vs expert-based immunophenotypic classification
of b-cell chronic lymphoproliferative disorders: a step
forward in the standardization of clinical immunophe-
notyping. Leukemia, 24(11):1927–1933.
Dworzak, M. N., Gaipa, G., Ratei, R., Veltroni, M., Schu-
mich, A., Maglia, O., Karawajew, L., Benetello, A.,
P
¨
otschger, U., Husak, Z., et al. (2008). Standardiza-
tion of flow cytometric minimal residual disease eval-
uation in acute lymphoblastic leukemia: Multicentric
assessment is feasible. Cytometry Part B: Clinical Cy-
tometry, 74(6):331–340.
Finak, G., Bashashati, A., Brinkman, R., and Gottardo, R.
(2009). Merging mixture components for cell popu-
lation identification in flow cytometry. Advances in
Bioinformatics, 2009.
Gonzalez-Garcia, A., Vezhnevets, A., and Ferrari, V.
(2015). An active search strategy for efficient object
class detection. In Conference on Computer Vision
and Pattern Recognition. IEEE.
Hariharan, B., Arbel
´
aez, P., Girshick, R., and Malik,
J. (2014). Hypercolumns for object segmenta-
tion and fine-grained localization. arXiv preprint
arXiv:1411.5752.
He, K., Zhang, X., Ren, S., and Sun, J. (2014). Spatial pyra-
mid pooling in deep convolutional networks for visual
recognition. In European Conference of Computer Vi-
sion. Springer.
Hofmanninger, J. and Langs, G. (2015). Mapping visual
features to semantic profiles for retrieval in medical
imaging. In Conference on Computer Vision and Pat-
tern Recognition. IEEE.
Krizhevsky, A., Sutskever, I., and Hinton, G. E. (2012). Im-
agenet classification with deep convolutional neural
networks. In Advances in neural information process-
ing systems, pages 1097–1105.
Lo, K., Brinkman, R. R., and Gottardo, R. (2008). Auto-
mated gating of flow cytometry data via robust model-
based clustering. Cytometry Part A, 73(4):321–332.
Naim, I., Datta, S., Rebhahn, J., Cavenaugh, J. S., Mos-
mann, T. R., and Sharma, G. (2014). Swift - scal-
able clustering for automated identification of rare cell
populations in large, high-dimensional flow cytometry
datasets, part 1: Algorithm design. Cytometry Part A,
85(5):408–421.
Pyne, S., Hu, X., Wang, K., Rossin, E., Lin, T.-I.,
Maier, L. M., Baecher-Allan, C., McLachlan, G. J.,
Tamayo, P., Hafler, D. A., et al. (2009). Auto-
mated high-dimensional flow cytometric data analy-
sis. Proceedings of the National Academy of Sciences,
106(21):8519–8524.
Qureshi, T. A., Hunter, A., and Al-Diri, B. (2014). A
bayesian framework for the local configuration of reti-
nal junctions. In Conference on Computer Vision and
Pattern Recognition. IEEE.
Ramanathan, V., Li, C., Deng, J., Han, W., Li, Z., Gu, K.,
Song, Y., Bengio, S., Rossenberg, C., and Fei-Fei, L.
(2015). Learning semantic relationships for better ac-
tion retrieval in images. In Conference on Computer
Vision and Pattern Recognition. IEEE.
Staal, J., Abr
`
amoff, M. D., Niemeijer, M., Viergever, M.,
Van Ginneken, B., et al. (2004). Ridge-based vessel
segmentation in color images of the retina. Medical
Imaging, IEEE Transactions on, 23(4):501–509.
Toedling, J., Rhein, P., Ratei, R., Karawajew, L., and Spang,
R. (2006). Automated in-silico detection of cell popu-
lations in flow cytometry readouts and its application
to leukemia disease monitoring. BMC bioinformatics,
7:282–282.
Vincent, P., Larochelle, H., Lajoie, I., Bengio, Y., and
Manzagol, P.-A. (2010). Stacked denoising autoen-
coders: Learning useful representations in a deep net-
work with a local denoising criterion. The Journal of
Machine Learning Research, 11:3371–3408.
Yoo, I., Alafaireet, P., Marinov, M., Pena-Hernandez, K.,
Gopidi, R., Chang, J.-F., and Hua, L. (2012). Data
mining in healthcare and biomedicine: a survey of the
literature. Journal of medical systems, 36(4):2431–
2448.
Zhang, Y., Sohn, K., Villegas, R., Pan, G., and Lee, H.
(2015). Improving object detection with deep convo-
lutional networks via bayesian optimization and struc-
tured prediction. In Conference on Computer Vision
and Pattern Recognition. IEEE.
Zheng, L., Wang, S., Tian, L., He, F., Liu, Z., and Tian, Q.
(2015). Query-adaptive late fusion for image search
and person re-identification. In Conference on Com-
puter Vision and Pattern Recognition. IEEE.
Zhou, Y., Chang, H., Barner, K., Spellman, P., and Parvin,
B. (2014). Classification of histology sections via
multispectral convolutional sparse coding. In Con-
ference on Computer Vision and Pattern Recognition.
IEEE.
Zhu, X., Suk, H.-I., and Shen, D. (2014). Matrix-
similarity based loss function and feature selection for
alzheimer’s disease diagnosis. In Conference on Com-
puter Vision and Pattern Recognition. IEEE.
VISAPP 2016 - International Conference on Computer Vision Theory and Applications
312