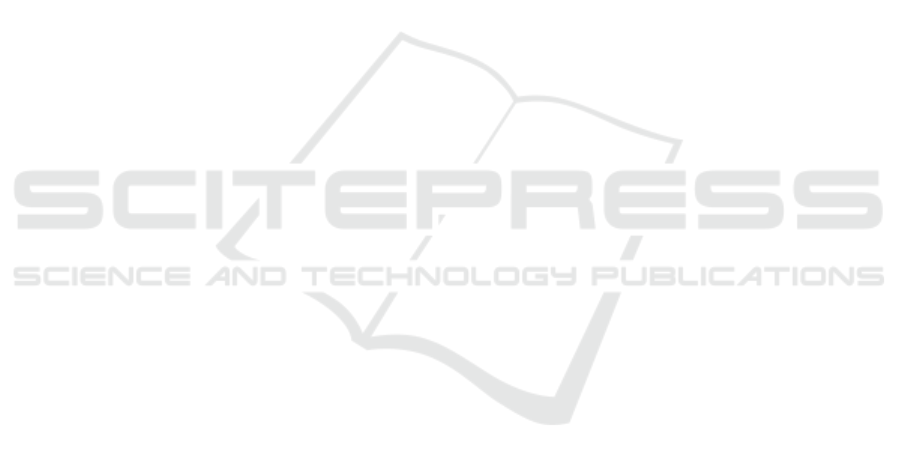
In comparison to other leading researches on the
topic of webcam eye gaze tracking (Ishikawa et al.,
2004; Kim and Kim, 2007; Valenti et al., 2012), we
have demonstrated that our system achieves better ac-
curacy. An average error of 2.25 degrees measured
with significant head movements is better than other
systems reporting an accuracy between 3 and 5 de-
grees.
4 CONCLUSIONS
We have presented a novel eye gaze tracking method
that requires only webcam quality RGB images.
We have demonstrated that this method performs
favourably to other systems presented in literature.
We believe that extending the idea further with more
accurate sensor readings, such as a depth sensor, can
lead to an even more accurate eye gaze tracking al-
gorithm which will be used in commercial systems in
the near future.
A further advantage of our approach is its capa-
bility to run in real time on contemporary mobile de-
vices. We have ported the algorithm to the Galaxy S4
smartphone and were able to obtain a speed of 15 fps
at a camera resolution of 640x480 pixels, when the
user was approximately 40cm away from the smart-
phone.
REFERENCES
Cootes, T. F., Edwards, G. J., and Taylor, C. J. (2001). Ac-
tive appearance models. IEEE Trans. Pattern Anal.
Mach. Intell., 23(6):681–685.
Czuprynski, B. and Strupczewski, A. (2014). High accu-
racy head pose tracking survey. In Active Media Tech-
nology, volume 8610 of Lecture Notes in Computer
Science, pages 407–420. Springer International Pub-
lishing.
Daugman, J. (2002). How iris recognition works. IEEE
Transactions on Circuits and Systems for Video Tech-
nology, 14:21–30.
Dodgson, N. A. (2004). Variation and extrema of human
interpupillary distance.
Goss, D. A. and West, R. (2002). Introduction to the optics
of the eye. Butterworth-Heinemann.
Hansen, D. W. and Ji, Q. (2010). In the eye of the beholder:
A survey of models for eyes and gaze. IEEE Trans.
Pattern Anal. Mach. Intell., 32(3):478–500.
Hennessey, C., Noureddin, B., and Lawrence, P. (2006). A
single camera eye-gaze tracking system with free head
motion. pages 87–94.
Ishikawa, T., Baker, S., Matthews, I., and Kanade, T.
(2004). Passive Driver Gaze Tracking with Active Ap-
pearance Models. Technical Report CMU-RI-TR-04-
08, Robotics Institute, Carnegie Mellon University,
Pittsburgh, PA.
Jang, J. and Kanade, T. (2004). Robust 3d head tracking by
online feature registration.
Jang, J.-S. and Kanade, T. (2010). Robust 3d head tracking
by view-based feature point registration. Available at
www.consortium.ri.cmu.edu/projCylTrack.php.
Kim, J.-T. and Kim, D. (2007). Gaze Tracking with Active
Appearance Models. Technical report, Department of
Computer Science and Engineering, Pohang Univer-
sity of Science and Technology.
Leutenegger, S., Chli, M., and Siegwart, R. Y. (2011).
Brisk: Binary robust invariant scalable keypoints. In
Proceedings of the 2011 International Conference on
Computer Vision, ICCV ’11, pages 2548–2555, Wash-
ington, DC, USA. IEEE Computer Society.
Liao, W.-K., Fidaleo, D., and Medioni, G. (2010). Robust:
Real-time 3d face tracking from a monocular view. J.
Image Video Process., 2010:5:1–5:15.
Lowe, D. G. (2004). Distinctive image features from scale-
invariant keypoints. Int. J. Comput. Vision, 60(2):91–
110.
Lu, F., Okabe, T., Sugano, Y., and Sato, Y. (2014). Learning
gaze biases with head motion for head pose-free gaze
estimation. Image and Vision Computing, 32(3):169 –
179.
Matthews, I. and Baker, S. (2004). Active appearance mod-
els revisited. Int. J. Comput. Vision, 60(2):135–164.
Morency, L., Whitehill, J., and Movellan, J. (2008). Gen-
eralized adaptive view-based appearance model: In-
tegrated framework for monocular head pose estima-
tion. pages 1–8.
Ohno, T., Mukawa, N., and Yoshikawa, A. (2002).
Freegaze: A gaze tracking system for everyday gaze
interaction. pages 125–132.
Riordan-Eva, P., Whitcher, J., Vaughan, D., and Asbury,
T. (2004). Vaughan & Asbury’s General Ophthal-
mology. A Lange medical book. Lange Medical
Books/McGraw Hill Medical Pub. Division.
Vacchetti, L., Lepetit, V., and Fua, P. (2004). Stable real-
time 3d tracking using online and offline informa-
tion. Pattern Analysis and Machine Intelligence, IEEE
Transactions on, 26(10):1385–1391.
Valenti, R., Sebe, N., and Gevers, T. (2012). Combining
head pose and eye location information for gaze es-
timation. Image Processing, IEEE Transactions on,
21(2):802–815.
Valenti, R., Staiano, J., Sebe, N., and Gevers, T. (2009).
Webcam-based visual gaze estimation. In Foggia, P.,
Sansone, C., and Vento, M., editors, Image Analysis
and Processing - ICIAP 2009, volume 5716 of Lecture
Notes in Computer Science, pages 662–671. Springer
Berlin Heidelberg.
Viola, P. and Jones, M. J. (2004). Robust real-time face
detection. Int. J. Comput. Vision, 57(2):137–154.
Wood, E. and Bulling, A. (2014). Eyetab: Model-based
gaze estimation on unmodified tablet computers. In
Proceedings of the Symposium on Eye Tracking Re-
search and Applications, ETRA ’14, pages 207–210,
New York, NY, USA. ACM.
VISAPP 2016 - International Conference on Computer Vision Theory and Applications
456