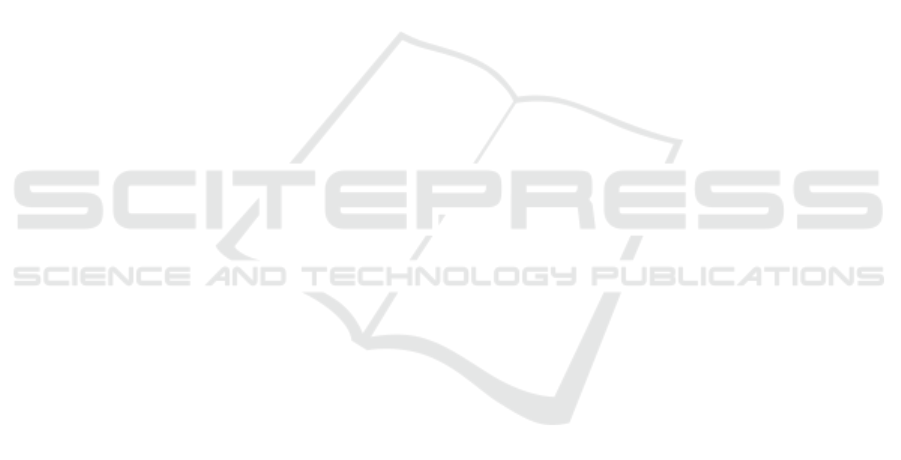
edge, being 12 classes in this experiment). We used
this dataset (MSRA 3D) since it allowed us to com-
pare our closed-set method with the state-of-the-art in
the field. However, we do not expect overfitting was a
problem for the scaling of τ. In particular, the consis-
tency of τ over various dataset splits (figure 6) would
be highly unexpected if overfitting was a serious prob-
lem.
In conclusion, we identify three purposes for our
anomaly detection methodology based on background
models: 1) increased accuracy in closed-set recogni-
tion tasks by acting as a confidence measure, 2) in-
creased robustness against open-set problems by fil-
tering of unknown videos and 3) as a first step to-
wards adaptive learning by closing the learning loop
of figure 1. Due to the large resemblance of human
intelligence, novelty detection can significantly ex-
tend both robotic functionality and human-robot in-
teraction. We intend to implement the novelty de-
tection methodology on our personal robot (Chandarr
et al., 2013) and tackle the challenges posed by un-
constrained motions and environments.
ACKNOWLEDGEMENTS
The authors want to thank Tim van Erven and David
Tax for the useful discussions.
REFERENCES
Aggarwal, J. and Xia, L. (2014). Human activity recog-
nition from 3D data: A review. Pattern Recognition
Letters, 48:70–80.
Chandarr, A., Bruinink, M., Gaisser, F., Rudinac, M., and
Jonker, P. (2013). Towards bringing service robots to
households: Robby ,Lea smart affordable interactive
robots. In IEEE/RSJ International Conference on Ad-
vanced Robotics (ICAR 2013).
Gales, M. and Young, S. (2008). The application of hidden
Markov models in speech recognition. Foundations
and trends in signal processing, 1(3):195–304.
Jiang, H. (2005). Confidence measures for speech recog-
nition: A survey. Speech communication, 45(4):455–
470.
Johansson, G. (1973). Visual perception of biological mo-
tion and a model for its analysis. Perception &
psychophysics, 14(2):201–211.
Kamppari, S. O. and Hazen, T. J. (2000). Word and phone
level acoustic confidence scoring. In ICASSP, pages
1799–1802. IEEE.
Kemp, T. and Schaaf, T. (1997). Estimating confidence us-
ing word lattices. In Kokkinakis, G., Fakotakis, N.,
and Dermatas, E., editors, EUROSPEECH. ISCA.
Li, W., Zhang, Z., and Liu, Z. (2008). Expandable data-
driven graphical modeling of human actions based on
salient postures. Circuits and Systems for Video Tech-
nology, IEEE Transactions on, 18(11):1499–1510.
Li, W., Zhang, Z., and Liu, Z. (2010). Action recognition
based on a bag of 3D points. In Computer Vision and
Pattern Recognition Workshops (CVPRW), 2010 IEEE
Computer Society Conference on, pages 9–14.
Markou, M. and Singh, S. (2003). Novelty detection: a
review–part 1: statistical approaches. Signal Process-
ing, 83(12):2481–2497.
Masud, M. M., Chen, Q., Khan, L., Aggarwal, C. C., Gao,
J., Han, J., Srivastava, A. N., and Oza, N. C. (2013).
Classification and adaptive novel class detection of
feature-evolving data streams. IEEE Trans. Knowl.
Data Eng., 25(7):1484–1497.
Nowozin, S. and Shotton, J. (2012). Action points: A repre-
sentation for low-latency online human action recog-
nition. Microsoft Research Cambridge, Tech. Rep.
MSR-TR-2012-68.
Pinker, S. (1984). Language Learnability and Language
Development. Cambridge, MA: Harvard University
Press.
Popoola, O. P. and Wang, K. (2012). Video-based abnor-
mal human behavior recognition—A review. Systems,
Man, and Cybernetics, Part C: Applications and Re-
views, IEEE Transactions on, 42(6):865–878.
Rahim, M. G., Lee, C.-H., and Juang, B.-H. (1997). Dis-
criminative utterance verification for connected dig-
its recognition. Speech and Audio Processing, IEEE
Transactions on, 5(3):266–277.
Raptis, M., Kirovski, D., and Hoppe, H. (2011). Real-
time classification of dance gestures from skeleton
animation. In Proceedings of the 2011 ACM SIG-
GRAPH/Eurographics Symposium on Computer An-
imation, SCA ’11, pages 147–156, New York, NY,
USA. ACM.
Rose, R. C., Juang, B.-H., and Lee, C.-H. (1995). A training
procedure for verifying string hypotheses in continu-
ous speech recognition. In ICASSP, pages 281–284.
IEEE Computer Society.
Shotton, J., Sharp, T., Kipman, A., Fitzgibbon, A., Finoc-
chio, M., Blake, A., Cook, M., and Moore, R. (2013).
Real-time human pose recognition in parts from sin-
gle depth images. Communications of the ACM,
56(1):116–124.
Sukkar, R., Lee, C.-H., et al. (1996). Vocabulary indepen-
dent discriminative utterance verification for nonkey-
word rejection in subword based speech recognition.
Speech and Audio Processing, IEEE Transactions on,
4(6):420–429.
Vieira, A. W., Nascimento, E. R., Oliveira, G. L., Liu, Z.,
and Campos, M. F. (2012). STOP: Space-time occu-
pancy patterns for 3D action recognition from depth
map sequences. In Progress in Pattern Recognition,
Image Analysis, Computer Vision, and Applications,
pages 252–259. Springer.
Wang, J., Liu, Z., Wu, Y., and Yuan, J. (2014). Learn-
ing actionlet ensemble for 3D human action recogni-
VISAPP 2016 - International Conference on Computer Vision Theory and Applications
326