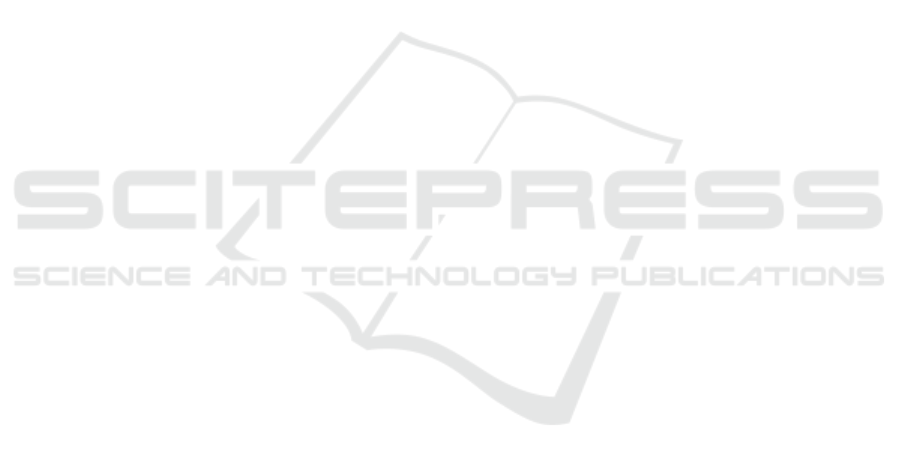
sis from overhead view. Machine vision and applica-
tions, 25(2):345–359.
Association, H. F. M. et al. (2003). Achieving operating
room efficiency through process integration. Health-
care financial management: journal of the Healthcare
Financial Management Association, 57(3):suppl–1.
Bardram, J. E., Hansen, T. R., and Soegaard, M. (2006).
Awaremedia: a shared interactive display supporting
social, temporal, and spatial awareness in surgery. In
Proceedings of the 2006 20th anniversary conference
on Computer supported cooperative work, pages 109–
118. ACM.
Behera, A., Cohn, A., and Hogg, D. (2014). Real-time ac-
tivity recognition by discerning qualitative relation-
ships between randomly chosen visual features. In
Proceedings of the British Machine Vision Confer-
ence. BMVA Press.
Bhatia, B., Oates, T., Xiao, Y., and Hu, P. (2007). Real-time
identification of operating room state from video. In
AAAI, volume 2, pages 1761–1766.
Bishop, C. M. et al. (2006). Pattern recognition and ma-
chine learning, volume 4. springer New York.
Bowman, A. W. and Azzalini, A. (2004). Applied smooth-
ing techniques for data analysis. Clarendon Press.
Brox, T. and Malik, J. (2011). Large displacement optical
flow: descriptor matching in variational motion esti-
mation. Pattern Analysis and Machine Intelligence,
IEEE Transactions on, 33(3):500–513.
Ciechanowicz, S. and Wilson, N. (2011). Delays to operat-
ing theatre lists: observations from a uk centre. The
Internet Journal of Health, 12(2).
Criminisi, A., Reid, I., and Zisserman, A. (2000). Single
view metrology. International Journal of Computer
Vision, 40(2):123–148.
Does, R. J., Vermaat, T. M., Verver, J. P., Bisgaard, S., and
Van den Heuvel, J. (2009). Reducing start time delays
in operating rooms. Journal of Quality Technology,
41(1):95–109.
Ester, M., Kriegel, H.-P., Sander, J., and Xu, X. (1996).
A density-based algorithm for discovering clusters in
large spatial databases with noise. In Kdd, volume 96,
pages 226–231.
Felzenszwalb, P. F., Girshick, R. B., and McAllester, D.
(2010a). Discriminatively trained deformable part
models, release 4.
Felzenszwalb, P. F., Girshick, R. B., McAllester, D., and
Ramanan, D. (2010b). Object detection with discrim-
inatively trained part based models. IEEE Transac-
tions on Pattern Analysis and Machine Intelligence,
32(9):1627–1645.
Hartley, R. and Zisserman, A. (2003). Multiple view geom-
etry in computer vision. Cambridge university press.
Hoiem, D., Efros, A. A., and Hebert, M. (2008). Putting
objects in perspective. International Journal of Com-
puter Vision, 80(1):3–15.
Kodali, B. S., Kim, D., Bleday, R., Flanagan, H., and Ur-
man, R. D. (2014). Successful strategies for the re-
duction of operating room turnover times in a tertiary
care academic medical center. Journal of Surgical Re-
search, 187(2):403–411.
Lange, P. M., Nielsen, K. L. G., Petersen, S. T., and
Bardram, J. E. (2010). Phase recognition in an op-
erating room using sensor technology. IT-university
of Copenhagen.
Lea, C., Facker, J., Hager, G., Taylor, R., and Saria, S.
(2013). 3d sensing algorithms towards building an in-
telligent intensive care unit. AMIA Summits on Trans-
lational Science Proceedings, 2013:136.
Macario, A. (2010). What does one minute of operat-
ing room time cost? Journal of clinical anesthesia,
22(4):233–236.
Nara, A., Izumi, K., Iseki, H., Suzuki, T., Nambu, K., and
Sakurai, Y. (2011). Surgical workflow monitoring
based on trajectory data mining. In New Frontiers in
Artificial Intelligence, pages 283–291. Springer.
Niu, Q., Peng, Q., El Mekkawy, T., Tan, Y. Y., Bruant, H.,
and Bernaerdt, L. (2011). Performance analysis of the
operating room using simulation. Proceedings of the
Canadian Engineering Education Association.
Padoy, N., Mateus, D., Weinland, D., Berger, M.-O., and
Navab, N. (2009). Workflow monitoring based on
3d motion features. In Computer Vision Workshops
(ICCV Workshops), 2009 IEEE 12th International
Conference on, pages 585–592. IEEE.
Pentico, D. W. (2007). Assignment problems: A golden
anniversary survey. European Journal of Operational
Research, 176(2):774–793.
Schuster, M., Pezzella, M., Taube, C., Bialas, E., Diemer,
M., and Bauer, M. (2013). Delays in starting morning
operating lists: An analysis of more than 20 000 cases
in 22 german hospitals. Deutsches
¨
Arzteblatt Interna-
tional, 110(14):237.
the NYP Wall of Knowledge, L. and manager, L. P.-O. Or-
dashboard.
Veres, G., Grabner, H., Middleton, L., and Van Gool, L.
(2011). Automatic workflow monitoring in indus-
trial environments. In Computer Vision–ACCV 2010,
pages 200–213. Springer.
Voulodimos, A., Kosmopoulos, D., Veres, G., Grabner, H.,
Van Gool, L., and Varvarigou, T. (2011). Online clas-
sification of visual tasks for industrial workflow mon-
itoring. Neural Networks, 24(8):852–860.
Xiao, Y., Hu, P., Hu, H., Ho, D., Dexter, F., Mackenzie,
C. F., Seagull, F. J., and Dutton, R. P. (2005). An
algorithm for processing vital sign monitoring data to
remotely identify operating room occupancy in real-
time. Anesthesia & Analgesia, 101(3):823–829.
Modeling Human Motion for Predicting Usage of Hospital Operating Room
335