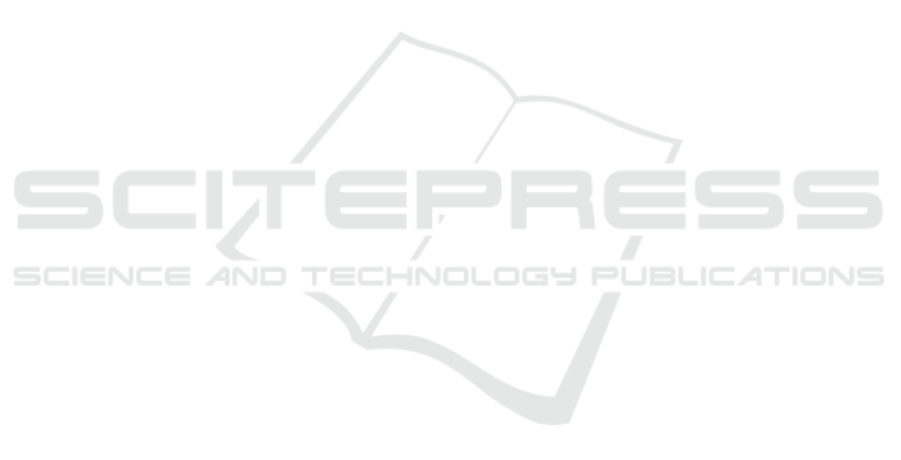
5 CONCLUSION
In this paper, criteria are proposed for assessing the
number of clusters in a mixture-model clustering ap-
proach with side-information. The side-information
defines constraints, grouping some points in the same
cluster.
Three criteria used for assessing the number of
clusters in a mixture-model clustering approach with-
out side-information have been modified. These cri-
teria are the Bayesian information criterion (BIC), the
Akaike information criterion (AIC) and the entropy
criterion (NEC). For adapting the criteria, the com-
putation of the log-likelihood has been modified. It
takes into account that some points arise from the
same source. In addition the criteria depend on the
number of chunklets but not on the total number of
points.
Experiments have been done with simulated prob-
lems: Gaussian mixtures and Gamma processes, and
with a real problem. The simulations allowed to com-
pare the efficiency of the criteria to determine the right
number of clusters. The climatic data problem has
given an application example.
The side-information helps to determine the clus-
ters mainly when the clusters overlap. Thus the cri-
teria fitted to such situations are the most efficient.
The experiments have shown the best behavior of BIC
compared with the two other criteria. AIC presents
a slight tendency to overestimate the correct number
of clusters while NEC has an underestimating ten-
dency. Because NEC is strongly efficient when the
mixture components are well separated, its perfor-
mance is quite poor for the considered experimental
cases.
The influence of point number per chunklet on the
performance of the proposed criteria has also been
studied. The larger the chunklet size is, the better the
clustering algorithm performances are, and the better
the estimated number of clusters is.
REFERENCES
Akaike, H. (1974). A new look at the statistical model iden-
tification. IEEE Transactions on Automatic Control,
19(6):716–723.
Biernacki, C., Celeux, G., and Govaert, G. (1999). An im-
provement of the nec criterion for assessing the num-
ber of clusters in a mixture model. Pattern Recogni-
tion Letters, 20(3):267–272.
Celeux, G. and Govaert, G. (1992). A classification EM
algorithm for clustering and two stochastic versions.
Computational statistics & Data analysis, 14(3):315–
332.
Celeux, G. and Govaert, G. (1995). Gaussian parcimonious
clustering models. Pattern Recognition, 28:781–793.
Celeux, G. and Soromenho, G. (1996). An entropy crite-
rion for assessing the number of clusters in a mixture
model. Journal of classification, 13(2):195–212.
Fonseca, J. R. and Cardoso, M. G. (2007). Mixture-model
cluster analysis using information theoretical criteria.
Intelligent Data Analysis, 11(1):155–173.
Grall-Ma
¨
es, E. (2014). Spatial stochastic process cluster-
ing using a local a posteriori probability. In Proceed-
ings of the IEEE International Workshop on Machine
Learning for Signal Processing (MLSP 2014), Reims,
France.
Lebarbier, E. and Mary-Huard, T. (2006). Le crit
`
ere BIC:
fondements th
´
eoriques et interpr
´
etation. Research re-
port, INRIA.
McLachlan, G. and Basford, K. (1988). Mixture models. in-
ference and applications to clustering. Statistics: Text-
books and Monographs, New York: Dekker, 1988, 1.
Schwarz, G. (1978). Estimating the dimension of a model.
Annals of Statistics, pages 461–464.
Shental, N., Bar-Hillel, A., Hertz, T., and Weinshall, D.
(2003). Computing gaussian mixture models with EM
using side-information. In Proc. of the 20th Interna-
tional Conference on Machine Learning. Citeseer.
Van Noortwijk, J. (2009). A survey of the application of
gamma processes in maintenance. Reliability Engi-
neering & System Safety, 94(1):2–21.
Assessing the Number of Clusters in a Mixture Model with Side-information
47