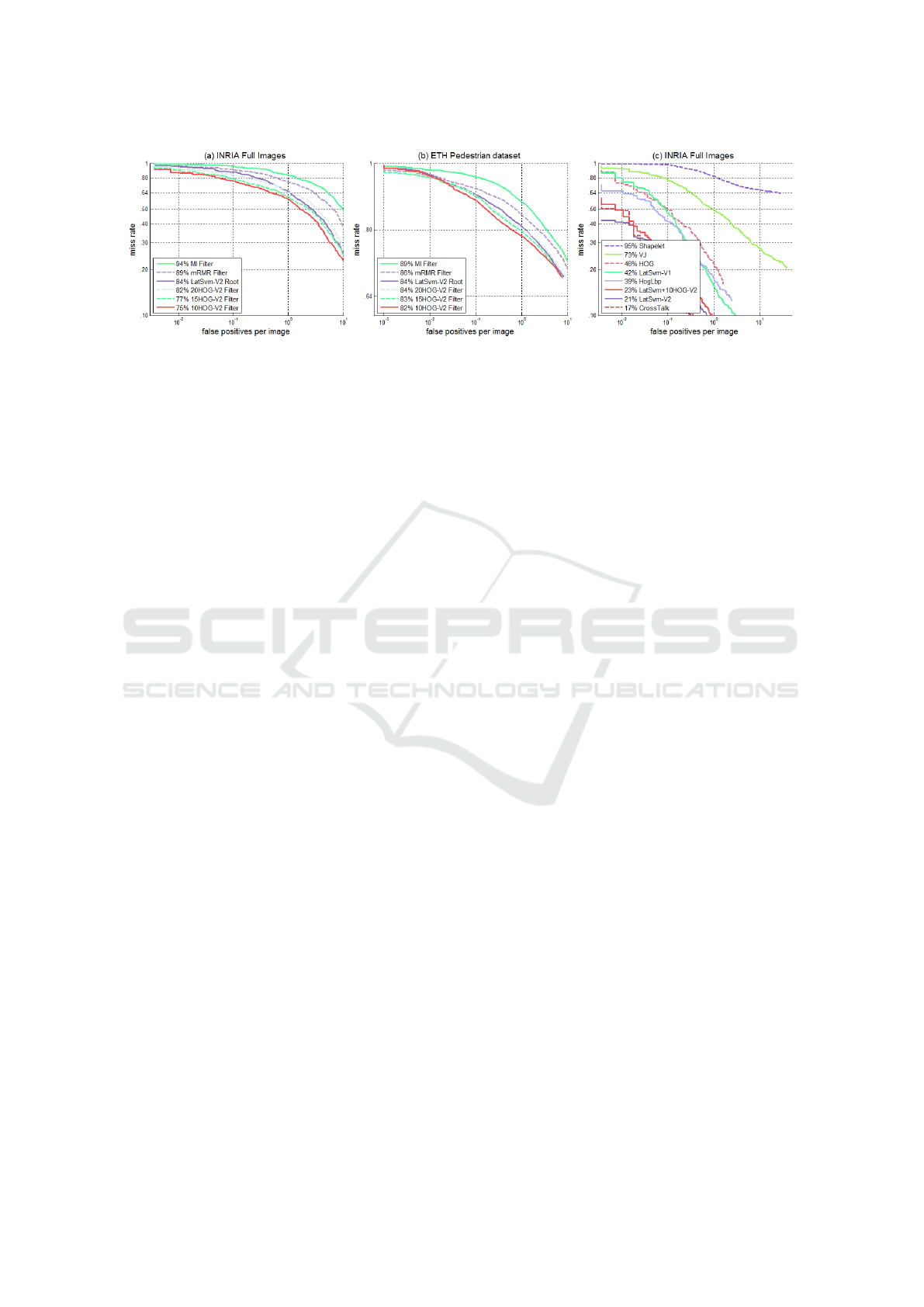
Figure 6: Performance comparison: (a) Filter detection performance comparison on the 288 INRIA Full Images. (b) Filter
detection performance on the ETH Bahnhof sequence, (c) Comparison to the state-of-the-arts on the 288 INRIA Full Images.
have demonstrated the chosen feature subset can be
used to improve the human detection system, which
relies on the classifier performance, in both speed and
accuracy. It has also been shown that the optimal fea-
tures represent the object shape. Base on this obser-
vation, we have demonstrated that the optimal feature
vector can be directly used to form the appearance
models. This approach does not require highly ac-
curate annotation data of objects to generate models.
Therefore, it can be easily applied to a wide range of
datasets.
REFERENCES
Alpaydin, E. (2004). In Introduction to Machine Learning.
The MIT Press.
Battiti, R. (1994). In Using Mutual Information for Select-
ing Features in Supervised Neural Net Learning. IEEE
Transactions on Neural Networks, Vol. 5, No. 4.
Bermejo, P., Gamez, J. A., and Puerta, J. M. (2011). In
Improving Incremental Wrapper-based Subset Selec-
tion via Replacement and Early Stopping. Interna-
tional Journal of Pattern Recognition and Artificial In-
telligence. Vol. 25.
Chandrashekar, G. and Sahinn, F. (2014). In A survey on
feature selection methods. Journal of Computers and
Electrical Engineering 40, P. 16-28.
Dalal, N. and Triggs, B. (2006). In Histograms of Oriented
Gradients for Human Detection. IEEE Conference on
Computer Vision and Pattern Recognition.
Doll
´
ar, P., Wojek, C., Schiele, B., and Perona, P. (2009).
In Pedestrian Detection: A benchmark. IEEE Confer-
ence on Computer Vision and Pattern Recognition.
Doll
´
ar, P., Wojek, C., Schiele, B., and Perona, P. (2012).
In Pedestrian Detection: An Evaluation of the State
of the Art. IEEE Transactions on Pattern Analysis and
Machine Intelligence.
Ess, A., Leibe, B., Schindler, K., , and van Gool, L. (2008).
In A Mobile Vision System for Robust Multi-Person
Tracking. IEEE Conference on Computer Vision and
Pattern Recognition.
Estevez, P. A., Tesmer, M., Perez, C. A., and Zurada, J. M.
(2009). In Normalized Mutual Information Feature
Selection. IEEE Transactions on Neural Networks,
Vol. 20, No.2.
Everingham, M., Zisserman, A., Williams, C., and Gool,
L. (2007). In The PASCAL visual obiect classes chal-
lenge 2007 results. Technical Report, PASCAL chal-
lenge 2007.
Felzenszwalb, P., Girshick, R., McAllester, D., and Ra-
manan, D. (2010). In Object Detection with Discrim-
inatively Trained Part Based Models. IEEE Confer-
ence on Computer Vision and Pattern Recognition.
Felzenszwalb, P., McAllester, D., and Ramanan, D.
(2008). In A Discriminatively Trained, Multiscale,
Deformable Part Model. IEEE Conference on Com-
puter Vision and Pattern Recognition.
Foithong, S., Pinngern, O., and Attachoo, B. (2012). In
Feature Subset Selection Wrapper based on Mutual
Information and Rough sets. Journal of Expert Sys-
tems with Applications 39, P.574-584, Elsevier.
Gutlein, M., Frank, E., Hall, M., and Karwath, A. (2009). In
Large-scale attribute selection using wrappers. IEEE
Symposium Series on Computational Intelligence and
Data Mining.
Guyon, I. and Elisseeff, A. (2003). In An Introduction to
Variable and Feature Selection. Journal of Machine
Learning Research 3, 1157-1182.
Heng, C. K., Yokomitsu, S., Matsumoto, Y., and Tmura,
H. (2012). In Shrink Boost for Selecting Multi-LBP
Histogram Features in Object Detection. IEEE Con-
ference on Computer Vision and Pattern Recognition.
Javed, K., Babri, H. A., and Saeed, M. (2012). In Fea-
ture Selection Based on Class-Dependent Densities
for High-Dimensional Binary Data. IEEE Transac-
tions on Knowledge and Data Engineering, Vol. 24,
P. 465-477.
Kohavi, R. and John, G. H. (1997). In Wrappers for feature
subset selection. Artificial Intelligence.
Li, K. and Peng, J. (2007). In Neural Input Selection - A fast
model-based approach. Journal of Neurocomputing,
Vol. 70, P. 762-769.
Peng, H., Long, F., , and Ding, C. (2005). In Feature Selec-
tion Based on Mutual Information: Criteria of Max-
Dependency, Max-Relevance, and Min-Redundancy.
k-fold Subsampling based Sequential Backward Feature Elimination
429