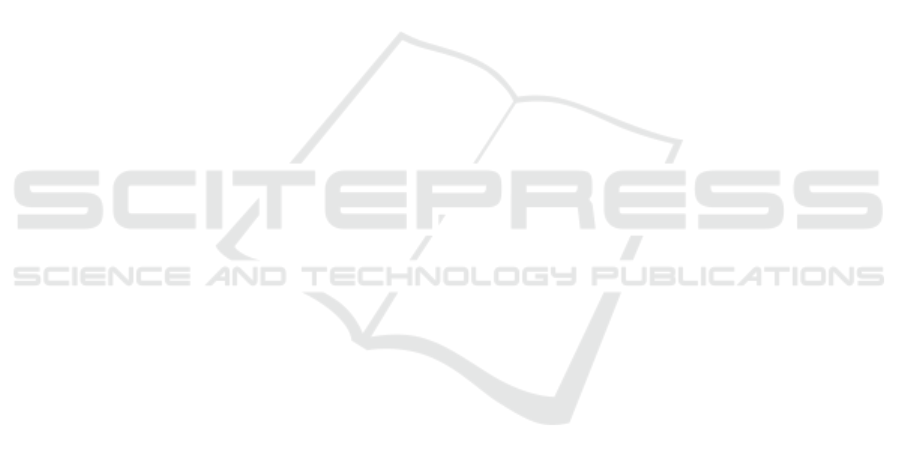
Liu, B., Xiao, Y., Cao, L., Hao, Z., and Deng, F.
(2013). Svdd-based outlier detection on uncer-
tain data. Knowledge and Information Systems,
34(3):597–618.
Lukovic, S., Kaitovic, I., Mura, M., and Bondi, U. (2010).
Virtual power plant as a bridge between distributed en-
ergy resources and smart grid. Hawaii International
Conference on System Sciences, 0:1–8.
McArthur, S., Davidson, E., Catterson, V., Dimeas, A.,
Hatziargyriou, N., Ponci, F., and Funabashi, T. (2007).
Multi-agent systems for power engineering applica-
tions – Part I: Concepts, approaches, and technical
challenges. IEEE Transactions on Power Systems,
22(4):1743–1752.
Neugebauer, J., Kramer, O., and Sonnenschein, M. (2015).
Classification cascades of overlapping feature ensem-
bles for energy time series data. In Proceedings of
the 3rd International Workshop on Data Analytics for
Renewable Energy Integration (DARE’15), ECML/
PKDD 2015. Springer.
Nieße, A., Beer, S., Bremer, J., Hinrichs, C., L
¨
unsdorf,
O., and Sonnenschein, M. (2014). Conjoint dynamic
aggrgation and scheduling for dynamic virtual power
plants. In Ganzha, M., Maciaszek, L. A., and Paprzy-
cki, M., editors, Federated Conference on Computer
Science and Information Systems - FedCSIS 2014,
Warsaw, Poland.
Nieße, A., Lehnhoff, S., Tr
¨
oschel, M., Uslar, M., Wiss-
ing, C., Appelrath, H.-J., and Sonnenschein, M.
(2012). Market-based self-organized provision of ac-
tive power and ancillary services: An agent-based ap-
proach for smart distribution grids. In COMPENG,
pages 1–5. IEEE.
Nieße, A. and Sonnenschein, M. (2013). Using grid related
cluster schedule resemblance for energy rescheduling
- goals and concepts for rescheduling of clusters in
decentralized energy systems. In Donnellan, B., Mar-
tins, J. F., Helfert, M., and Krempels, K.-H., editors,
SMARTGREENS, pages 22–31. SciTePress.
Nikonowicz, Ł. B. and Milewski, J. (2012). Virtual power
plants – general review: structure, application and op-
timization. Journal of Power Technologies, 92(3).
Park, J., Kang, D., Kim, J., Kwok, J. T., and Tsang, I. W.
(2007). Svdd-based pattern denoising. Neural Com-
puting, 19(7):1919–1938.
Powers, D. M. W. (2008). Evaluation evaluation. In Pro-
ceedings of the 2008 Conference on ECAI 2008: 18th
European Conference on Artificial Intelligence, pages
843–844, Amsterdam, The Netherlands, The Nether-
lands. IOS Press.
Powers, D. M. W. (2011). Evaluation: From precision, re-
call and f-measure to roc., informedness, markedness
& correlation. Journal of Machine Learning Tech-
nologies, 2(1):37–63.
Ramchurn, S. D., Vytelingum, P., Rogers, A., and Jennings,
N. R. (2012). Putting the ’smarts’ into the smart grid:
A grand challenge for artificial intelligence. Commun.
ACM, 55(4):86–97.
Rapp, B. and Bremer, J. (2012). Design of an event en-
gine for next generation cemis: A use case. In Hans-
Knud Arndt, Gerlinde Knetsch, W. P. E., editor, Envi-
roInfo 2012 – 26th International Conference on Infor-
matics for Environmental Protection, pages 753–760.
Shaker Verlag. ISBN 978-3-8440-1248-4.
Sch
¨
olkopf, B. (1997). Support Vector Learning. Disserta-
tion, Fachbereich 13 Informatik der Technischen Uni-
versit
¨
at Berlin, Oldenbourg Verlag, M
¨
unchen.
Sch
¨
olkopf, B., Mika, S., Burges, C., Knirsch, P., M
¨
uller, K.-
R., R
¨
atsch, G., and Smola, A. (1999). Input space vs.
feature space in kernel-based methods. IEEE Trans-
actions on Neural Networks, 10(5):1000–1017.
Sri, M., Huld, T., Dunlop, E. D., Albuisson, M., Lefevre,
M., and Wald, L. (2007). Uncertainties in photovoltaic
electricity yield prediction from fluctuation of solar
radiation. In 22nd European Photovoltaic Solar En-
ergy Conference.
Stadler, P. D.-I. I. (2005). Demand Response: Nichtelek-
trische Speicher fr Elektrizittsversorgungssysteme mit
hohem Anteil erneuerbarer Energien. Habilitation,
Fachbereich Elektrotechnik, Universitt Kassel.
Tax, D. M. J. and Duin, R. P. W. (2004). Support vector data
description. Mach. Learn., 54(1):45–66.
Wang, J., Botterud, A., Bessa, R., Keko, H., Carvalho,
L., Issicaba, D., Sumaili, J., and Miranda, V. (2011).
Wind power forecasting uncertainty and unit commit-
ment. Applied Energy, 88(11):4014 – 4023.
Wildt, T. (2014). Modelling uncertainty of household deci-
sion – making process in smart grid appliances adop-
tion. In Behave Energy Conference, Oxford, UK.
Witten, I. H., Frank, E., and Hall, M. A. (2011). Data
Mining: Practical Machine Learning Tools and Tech-
niques. Morgan Kaufmann, Amsterdam, 3 edition.
Wu, F., Moslehi, K., and Bose, A. (2005). Power system
control centers: Past, present, and future. Proceedings
of the IEEE, 93(11):1890–1908.
Zhang, J., Hodge, B.-M., Gomez-Lazaro, E., Lovholm, A.,
Berge, E., Miettinen, J., Holttinen, H., and Cutululis,
N. (2013). Analysis of Variability and Uncertainty in
Wind Power Forecasting: An International Compari-
son. Energynautics GmbH.
Zheng, E.-H., Yang, M., Li, P., and Song, Z.-H. (2006).
Fuzzy support vector clustering. In Wang, J., Yi, Z.,
Zurada, J. M., Lu, B.-L., and Yin, H., editors, ISNN
(1), volume 3971 of Lecture Notes in Computer Sci-
ence, pages 1050–1056. Springer.
Zio, E. and Aven, T. (2011). Uncertainties in smart grids
behavior and modeling: What are the risks and vul-
nerabilities? how to analyze them? Energy Policy,
39(10):6308 – 6320. Sustainability of biofuels.
ICAART 2016 - 8th International Conference on Agents and Artificial Intelligence
50