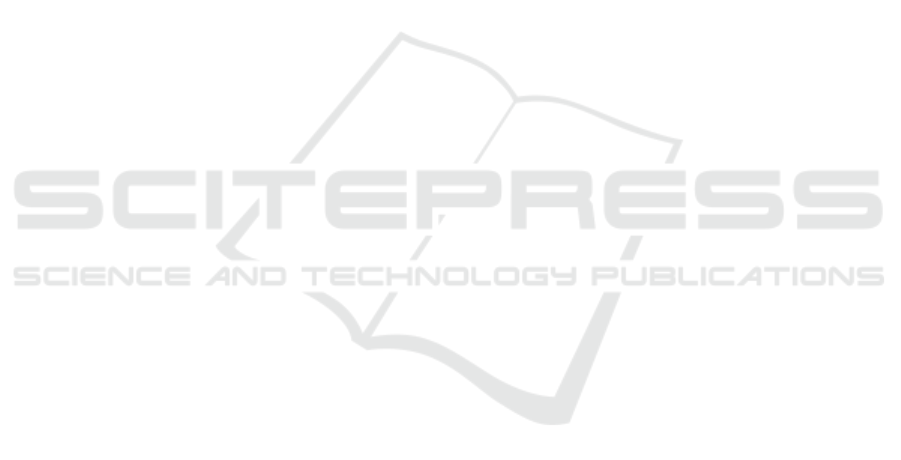
a different manner: rising graphs are seen by the sub-
jects to be more optimistic than they really are, while
the subjects interpret a declining graph in a nega-
tive manner. As our subjects were not questioned on
whether they found it easier to find a certain graph
type, we cannot conclude whether the graph types
have absolutely no effect on users in terms of positive
or negative perception and correct decision-making.
6 CONCLUSION
Our findings can be applied to a whole number of
different domains e.g. web design. While not be-
ing classic dashboards, web pages, as well as other
user interfaces, often carry out the same tasks as dash-
boards (display information graphs, allow users to
make immediate decisions, convey important infor-
mation). The paper could be used as one of the guide-
lines for both web and dashboard designers, when
making choices about graph placements and graph
sizes relative to the screen. Many websites could ben-
efit from the idea that the amount of graphs that is
being displayed simultaneously is not as important as
the size of those graphs (or any other images, text-
blocks or information).
When designing the dashboard (or a dashboard
screen) designers should note that the graphs that oc-
cupy a relatively small amount of the active screen
convey information quicker than larger ones.
It also important to note that during the first ex-
periment a change in a visual searching strategy by
the subjects was noted. This is an interesting concept
that can be explored further in future works.
The paper deals with the idea of whether bigger
graphs are better for perception speed and whether
a greater number of graphs makes information per-
ception difficult. Our findings showed that the useful
screen area corresponds to the speed of the problem
solving. This means that dashboard designers should
sacrifice screen area, use smaller graphs and leave
empty spaces if the dashboard (dashboard screen they
are designing) is used for basic immediate assess-
ment. If a more in-depth assessment is required,
larger graphs are recommended.
The paper also contributes to the studies of infor-
mation perception in terms of both user interfaces and
infographics. The paper may serve as the base for a
more in-depth study of information perception. As
a follow-up of the experiments, an eye-tracking study
should be carried out on other types of graphs, such as
bar graphs or pie charts, in order to find out whether
our conclusions are true for other types of information
graphs. Adding graphs that are more complicated or
information from a specific domain, greater distract-
ing points of interest or longer and more demanding
tasks (such as exploring, concluding, comparing and
clustering (Yost and North, 2006)) could also be in-
troduced. This would help to understand how design-
ers should act, when their dashboard needs to convey
more information (not just help to identify a graph
with the correct trend relative to the task) or when
only brief and immediate information needs to be dis-
played.
REFERENCES
Bera, P. (2014). Do Distracting Dashboards Matter? Ev-
idence from an Eye Tracking Study. In Information
Systems: Education, Applications, Research, pages
65–74. Springer.
Brath, R. and Peters, M. (2004). Dashboard Design: Why
Design is Important. Data Mining Review/Data Min-
ing Direct, (October).
Bridgeman, B., Van der Heijden, A. H. C., and
Velichkovsky, B. M. (1994). A theory of visual sta-
bility across saccadic eye movements. Behavioral and
Brain Sciences, 17(02):247–258.
Burgert, O.,
¨
Orn, V., Velichkovsky, B. M., Gessat, M.,
Joos, M., Strauss, G., Tietjen, C., Preim, B., and Her-
tel, I. (2007). Evaluation of Perception Performance
in Neck Dissection Planning using Eye Tracking and
Attention Landscapes. In Jiang, Y. and Sahiner, B.,
editors, SPIE Proceedings, page 9 pages. Society of
Photo-Optical Instrumentation Engineers.
Cleveland, W. S. and McGill, R. (1985). Graphical per-
ception and graphical methods for analyzing scientific
data. Science, 229(4716):828–833.
Cleveland, W. S. and McGill, R. (1987). Graphical per-
ception: The visual decoding of quantitative infor-
mation on graphical displays of data. Journal of the
Royal Statistical Society. Series A (General), pages
192–229.
Duchowski, A. (2007). Eye Tracking Methodology.
Springer Science+Business Media, London.
Few, S. (2006). Information dashboard design. O’Reilly.
Goldberg, J. H. and Helfman, J. I. (2010). Comparing In-
formation Graphics: A Critical Look at Eye Tracking.
In 3rd BELIV’10 Workshop: Beyond time and errors:
Novel evaluation methods for information visualiza-
tion., pages 71–78. ACM.
Harel, E. C. and Sitko, T. D. (2003). Driving Higher Edu-
cation Decisions. Research Bulletin, 1(19):2–10.
Jacob, R. J. K. and Karn, K. S. (2003). Eye tracking in
HumanComputer interaction and usability research:
Ready to deliver the promises. The Mind’s Eye:
Cognitive and Applied Aspects of Eye Movement Re-
search, pages 573–605.
Newman, G. E. and Scholl, B. J. (2012). Bar graphs de-
picting averages are perceptually misinterpreted: The
IVAPP 2016 - International Conference on Information Visualization Theory and Applications
212