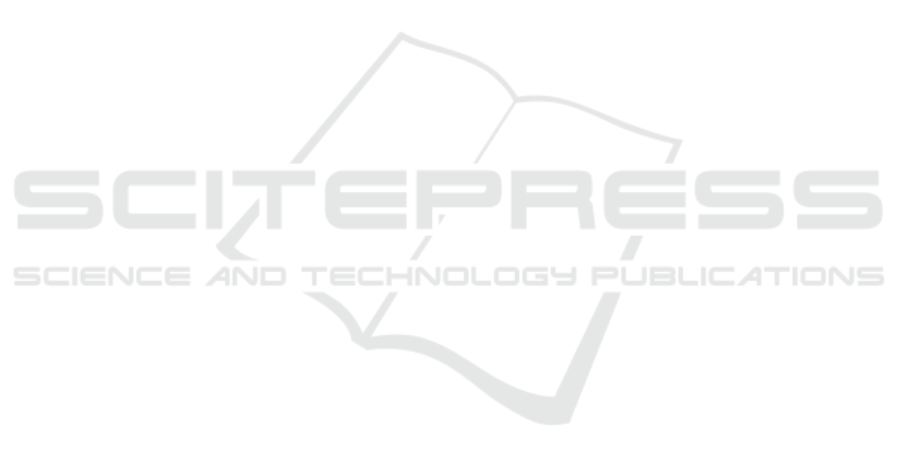
ACKNOWLEDGEMENTS
I would like to thank the state Saxony-Anhalt for the
financial support of this work.
REFERENCES
Auclair, A., Vincent, N., and Cohen, L. (2006). Hash func-
tions for near duplicate image retrieval. In Applica-
tions of Computer Vision (WACV), pages 7–8.
Beecks, C. and Seidl, T. (2009). Visual exploration of large
multimedia databases. In Data Management and Vi-
sual Analytics Workshop.
Chu, L., Jiang, S., Wang, S., Zhang, Y., and Huang, Q.
(2013). Robust spatial consistency graph model for
partial duplicate image retrieval. In Multimedia, IEEE
Transactions on, pages 1982–1996.
Chum, O., Philbin, J., Isard, M., and Zisserman, A. (2007).
Scalable near identical image and shot detection. In
Proc. CIVR.
Chum, O., Philbin, J., and Zisserman, A. (2008). Near du-
plicate image detection: min-hash and tf-idf weight-
ing. In British Machine Vision Conference.
Grauman, K. and Darrell, T. (2005). Pyramid match ker-
nels: Discriminative classification with sets of image
features. In Proc. ICCV.
Grauman, K. and Darrell, T. (2007). The pyramid match
kernel: Efficient learning with sets of features. In The
Journal of Machine Learning Research, pages 725–
760.
J
`
egou, H., Douze, M., Schmid, C., and P
`
erez, P. (2010).
Aggregating local descriptors into a compact image
representation. In Proc. IEEE Conf. Computer Vision
and Pattern Recognition.
Jiang, M., Zhang, S., Li, H., and Metaxas, D. N. (2015).
Computer-aided diagnosis of mammographic masses
using scalable image retrieval. In Biomedical Engi-
neering, IEEE Transactions on, pages 783–792.
Ke, Y. and Sukthankar, R. (2004). Pca-sift: A more distinc-
tive representation for local image descriptors. In in:
CVPR, issue 2, page 506513.
Khan, N., McCane, B., and Wyvill, G. (2011). Sift and
surf performance evaluation against various image de-
formations on benchmark dataset. In Digital Image
Computing Techniques and Applications (DICTA).
Li, J., Qian, X., Li, Q., Zhao, Y., Wang, L., and Tang,
Y. Y. (2014). Mining near duplicate image groups.
In Springer Science and Business Media New York.
Low, T., Hentschel, C., Stober, S., Sack, H., and
N
¨
urnberger, A. (2014). Visual berrypicking in large
image collections. In Proceedings of the 8th Nordic
Conference on Human-Computer Interaction: fun,
fast, foundational, pages 1043–1046. New York, NY :
ACM.
Lowe, D. (2004). Distinctive image features from scale-
invariant keypoints. In Journal of Computer Vision,
pages 91–110.
Nist
`
er, D. and Stew
`
enius, H. Recognition benchmark
images. In available at http://www.vis.uky.edu/
∼stewe/ukbench/.
Nist
`
er, D. and Stew
`
enius, H. (2006). Scalable recognition
with a vocabulary tree. In CVPR, pages 2161–2168.
Steffen, J., Christian, H., Alyosef, A. A., T
¨
onnies, K., and
N
¨
urnberger, A. (2012). Rotational invariance at fixa-
tion points - experiments using human gaze data. In
Proceedings of the 1st International Conference on
Pattern Recognition Applications and Methods, pages
451–456.
Steinbach, M., Ertoz, L., and Kumar (2003). The challenges
of clustering high dimensional data. In Wille LT, ed-
itor. New Vistas in Statistical Physics-Applications in
Econophysics, Bioinformatics, and Pattern Recogni-
tion. Springer-Verlag.
Xu, D., Cham, T., Yan, S., Duan, L., and Chang, S. (2010).
Near duplicate identification with spatially aligned
pyramid matching. In IEEE Trans. Circuits and Sys-
tems for Video Technology, pages 1068–1079.
Yang, Y. and Newsam, S. (2008). Comparing sift descrip-
tors and gabor texture features for classification of re-
mote sensed imagery. In Proceedings of the 15th IEEE
on Image Processing, San Diego, pages 1852–1855.
USA.
Zhang, C., Wang, S., Huang, Q., Liu, J., Liang, C., and Tian,
Q. (2013). Image classification using spatial pyramid
robust sparse coding. In Pattern Recognition letters,
pages 1046–1052.
Zhang, D. Q. and et al. (2004). Detecting image
near-duplicate by stochastic attribute relational graph
matching with learning. In Proceedings of the 12th
annual ACM international conference on Multimedia.
ICPRAM 2016 - International Conference on Pattern Recognition Applications and Methods
64