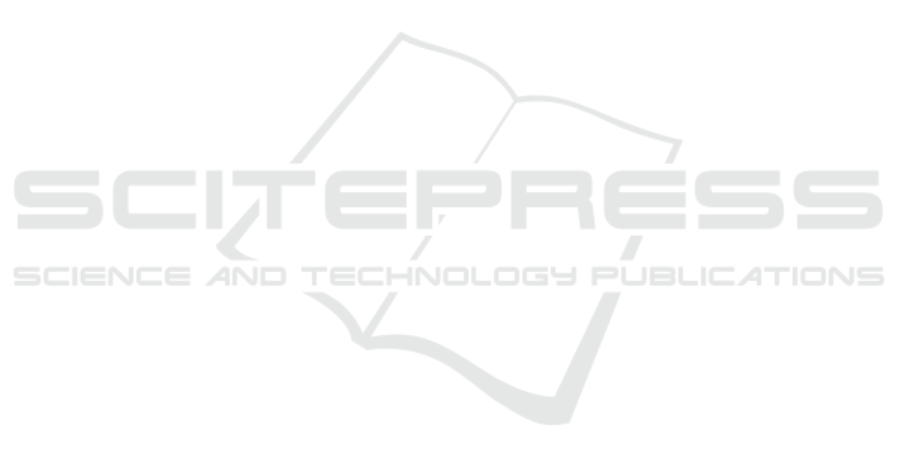
ror, and feature selection. Information and Control,
39(3):227–242.
Bhattacharya, B. K. and Toussaint, G. T. (1982). An upper
bound on the probability of misclassification in terms
of matusita’s measure of affinity. Annals of the Insti-
tute of Statistical Mathematics, 34(1):161–165.
Bhattacharyya, A. (1943). On a measure of divergence
between two statistical populations defined by their
probability distributions. Bull. Calcutta Math. Soc,
35(99-109):4.
Bhattacharyya, A. (1946). On a measure of divergence be-
tween two multinomial populations. Sankhy
˜
a: The In-
dian Journal of Statistics (1933-1960), 7(4):401–406.
Blackwell, D. (1951). Comparison of experiments. In Sec-
ond Berkeley Symposium on Mathematical Statistics
and Probability, volume 1, pages 93–102.
Bradt, R. and Karlin, S. (1956). On the design and compar-
ison of certain dichotomous experiments. The Annals
of mathematical statistics, pages 390–409.
Brody, D. C. and Hughston, L. P. (1998). Statistical geom-
etry in quantum mechanics. Proceedings of the Royal
Society of London. Series A: Mathematical, Physical
and Engineering Sciences, 454(1977):2445–2475.
Budzy
´
nski, R. J., Kondracki, W., and Kr
´
olak, A. (2008).
Applications of distance between probability distribu-
tions to gravitational wave data analysis. Classical
and Quantum Gravity, 25(1):015005.
Burbea, J. and Rao, C. R. (1982). On the convexity of
some divergence measures based on entropy func-
tions. IEEE Transactions on Information Theory,
28(3):489 – 495.
Chernoff, H. (1952). A measure of asymptotic efficiency for
tests of a hypothesis based on the sum of observations.
The Annals of Mathematical Statistics, 23(4):pp. 493–
507.
Choi, E. and Lee, C. (2003). Feature extraction based
on the Bhattacharyya distance. Pattern Recognition,
36(8):1703–1709.
Csiszar, I. (1967). Information-type distance measures
and indirect observations. Stud. Sci. Math. Hungar,
2:299–318.
Csiszar, I. (1975). I-divergence geometry of probability dis-
tributions and minimization problems. The Annals of
Probability, 3(1):pp. 146–158.
DasGupta, A. (2011). Probability for Statistics and Ma-
chine Learning. Springer Texts in Statistics. Springer
New York.
Finn, L. S. (1992). Detection, measurement, and gravita-
tional radiation. Physical Review D, 46(12):5236.
Gibbs, A. and Su, F. (2002). On choosing and bounding
probability metrics. International Statistical Review,
70(3):419–435.
Hellinger, E. (1909). Neue begr
¨
undung der theo-
rie quadratischer formen von unendlichvielen
ver
¨
anderlichen. Journal f
¨
ur die reine und angewandte
Mathematik (Crelle’s Journal), (136):210–271.
Hellman, M. E. and Raviv, J. (1970). Probability of Error,
Equivocation, and the Chernoff Bound. IEEE Trans-
actions on Information Theory, 16(4):368–372.
Jaranowski, P. and Kr
´
olak, A. (2007). Gravitational-wave
data analysis. formalism and sample applications: the
gaussian case. arXiv preprint arXiv:0711.1115.
Kadota, T. and Shepp, L. (1967). On the best finite set
of linear observables for discriminating two gaussian
signals. IEEE Transactions on Information Theory,
13(2):278–284.
Kailath, T. (1967). The Divergence and Bhattacharyya Dis-
tance Measures in Signal Selection. IEEE Transac-
tions on Communications, 15(1):52–60.
Kakutani, S. (1948). On equivalence of infinite product
measures. The Annals of Mathematics, 49(1):214–
224.
Kapur, J. (1984). A comparative assessment of various mea-
sures of directed divergence. Advances in Manage-
ment Studies, 3(1):1–16.
Kullback, S. (1968). Information theory and statistics. New
York: Dover, 1968, 2nd ed., 1.
Kullback, S. and Leibler, R. A. (1951). On information
and sufficiency. The Annals of Mathematical Statis-
tics, 22(1):pp. 79–86.
Kumar, U., Kumar, V., and Kapur, J. N. (1986). Some
normalized measures of directed divergence. Inter-
national Journal of General Systems, 13(1):5–16.
Lamberti, P. W., Majtey, A. P., Borras, A., Casas, M., and
Plastino, A. (2008). Metric character of the quan-
tum Jensen-Shannon divergence . Physical Review A,
77:052311.
Lee, Y.-T. (1991). Information-theoretic distortion mea-
sures for speech recognition. Signal Processing, IEEE
Transactions on, 39(2):330–335.
Lin, J. (1991). Divergence measures based on the shannon
entropy. IEEE Transactions on Information Theory,
37(1):145 –151.
Matusita, K. (1967). On the notion of affinity of several dis-
tributions and some of its applications. Annals of the
Institute of Statistical Mathematics, 19(1):181–192.
Maybank, S. J. (2004). Detection of image structures using
the fisher information and the rao metric. IEEE Trans-
actions on Pattern Analysis and Machine Intelligence,
26(12):1579–1589.
Nielsen, F. and Boltz, S. (2011). The burbea-rao and bhat-
tacharyya centroids. IEEE Transactions on Informa-
tion Theory, 57(8):5455–5466.
Nielsen, M. and Chuang, I. (2000). Quantum computation
and information. Cambridge University Press, Cam-
bridge, UK, 3(8):9.
Peter, A. and Rangarajan, A. (2006). Shape analysis using
the fisher-rao riemannian metric: Unifying shape rep-
resentation and deformation. In Biomedical Imaging:
Nano to Macro, 2006. 3rd IEEE International Sympo-
sium on, pages 1164–1167. IEEE.
Poor, H. V. (1994). An introduction to signal detection and
estimation. Springer.
Qiao, Y. and Minematsu, N. (2010). A study on in-
variance of-divergence and its application to speech
recognition. Signal Processing, IEEE Transactions
on, 58(7):3884–3890.
Quevedo, H. (2008). Geometrothermodynamics of black
holes. General Relativity and Gravitation, 40(5):971–
984.
ICPRAM 2016 - International Conference on Pattern Recognition Applications and Methods
82