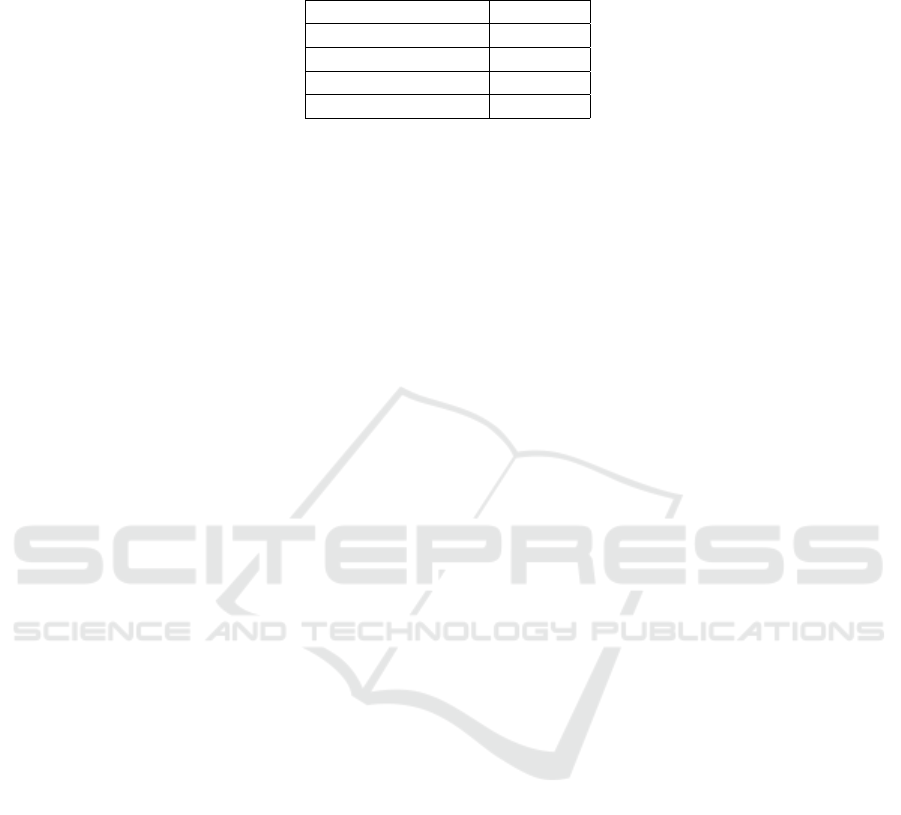
Table 4: Comparison of the proposed approach (geometric features) with respect to other techniques: an early standard
approach based on grey-valued images analysis (Proietti et al., 2014), the approach based only on contour analysis (Di Claudio
et al., 2015) and an approach based on binary template matching (Proietti et al., 2015).
Algorithms Error rate
Geometric features 2%
Grey-valued images 15%
Contour analysis 4%
Template matching 9%
Table 4. Observe that the performance of the present
approach exceeds the performance of the said ones by
a sizeable margin.
As far as the computational costs are concerned,
the time required by all these algorithms for perform-
ing the classification task is comparable. They are less
than 100µs, referring to a x64 Intel(R) Core(TM) i7-
2600K CPU running at 3.40 GHz with 8 GB, 1333
MHz RAM.
Moreover, let us mention that an approach based
on binary template matching (Proietti et al., 2015)
was also tried in previous works (Proietti et al., 2015).
However, this technique yielded a misclassification
error greater than 9% with a much higher computa-
tional time.
5 CONCLUSIONS
A new approach for the classification of dust on the
basis of their size and typology (particles and fibres)
based on geometric features extracted from binary im-
ages was presented. The approach represents an ef-
fective choice in terms of speed and accuracy and re-
quires a very simple acquisition device.
Since the involved algorithms are essentially
multiplication-free, the global classification tech-
nique is very fast and energy saving. Hence, it is ideal
in distributed sensor networks and especially in wire-
less scenarios, where the processing power consump-
tion is a major problem, since the classification task
competes with the energy spent for communication
among sensing devices and image acquisition.
The aim of this paper is to propose a novel ap-
proach to features selection for fibres classification.
In the paper, encouraging results on real dataset by us-
ing well-known classification models have been pre-
sented. A deep assessment of more complex scenar-
ios, with different datasets and different classifiers,
will be considered in future contributions. Also, fu-
ture works will entail the use of enhanced optics over
the CMOS sensors to capture even smaller particles
and more detailed classification.
REFERENCES
Baron, P. (2001). Measurement of airborne fibers: A review.
Industrial Health, 39(2):39–50.
Bogomolny, A. (1987). On the perimeter and area of fuzzy
sets. Fuzzy Sets and Systems, 23(2):257 – 269.
Camuffo, D. (2013). Microclimate for Cultural Heritage:
Conservation, Restoration, and Maintenance of In-
door and Outdoor Monuments. Elsevier Science,
Boston.
Cover, T. and Hart, P. (1967). Nearest neighbor pattern clas-
sification. Information Theory, IEEE Transactions on,
13(1):21–27.
Di Claudio, E., Jacovitti, G., Orlandi, G., and Proietti, A.
(2015). Fast classification of dust particles from shad-
ows. In ICPRAM 2015 - 4th International Confer-
ence on Pattern Recognition Applications and Meth-
ods, Proceedings, volume 2, pages 241–247.
Drolon, H., Druaux, F., and Faure, A. (2000). Particles
shape analysis and classification using the wavelet
transform. Pattern Recognition Letters, 21(67):473 –
482.
Fisher, R. A. (1938). The statistical utilization of multiple
measurements. Annals of Eugenics, 8(4):376–386.
Ghedini, N., Ozga, I., Bonazza, A., Dilillo, M., Cachier, H.,
and Sabbioni, C. (2011). Atmospheric aerosol moni-
toring as a strategy for the preventive conservation of
urban monumental heritage: The florence baptistery.
Atmospheric Environment, 45(33):5979 – 5987.
Golub, G. and Loan, C. V. (1989). Matrix Computations.
John Hopkins University Press, Baltimore, USA, 2nd
edition.
Gonzalez, R. C., Woods, R. E., and Eddins, S. L. (2004).
Digital image processing using matlab. Upper Saddle
River, N. J: Pearson Prentice Hall.
ISO (2010). Cleanrooms and associated controlled envi-
ronments - part 1: Classification of air cleanliness by
particle concentration. ISO/DIS 14644-1.
Krzanowski, W. J., editor (1988). Principles of Multivariate
Analysis: A User’s Perspective. Oxford University
Press, Inc., New York, NY, USA.
Langley, P., Iba, and, W., and Thompson, K. (1992). An
analysis of bayesian classifiers. In Proceedings of the
Tenth National Conference on Artificial Intelligence,
AAAI’92, pages 223–228. AAAI Press.
Lawrence, R. L. and Wright, A. (2001). Rule-based classifi-
cation systems using classification and regression tree
(cart) analysis. Photogrammetric engineering and re-
mote sensing, 67(10):1137–1142.
Classification of Dust Elements by Spatial Geometric Features
253