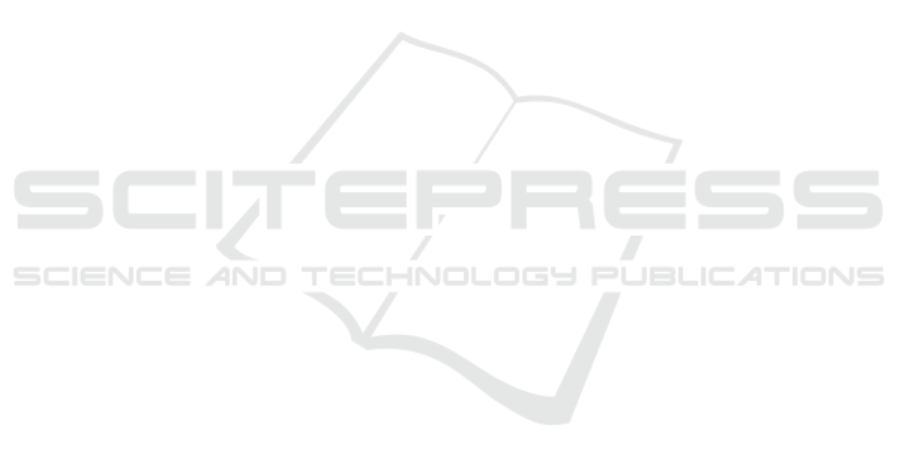
brain tissue structure in an unsupervised setting. Sub-
sequently we have tried to train a random forest to
learn the structure of the subspace clusters.
Subspace Learning and Spatial Features:
Though the classifier can’t discriminate between nor-
mal tissues and tumor spectra, it provides a good seg-
mentation of the tissues and surgical materials in the
scene. The H2NMF hierarchy provides a hierarchy of
low rank sub-matrices that contain pure-pixels, and in
this case correspond to different brain tissues. This
cluster hierarchy can further be used by a surgeon in
loop to search more pertinent subspaces and feed the
results back to the classifier. We have seen the effect
of learning classifiers on different levels of the hier-
archical clustering. Though this produces reasonable
segmentations, it does not approximate the subspace
hierarchy exactly. We will study how to define loss
functions to encode this structure. Given that the dif-
ference in spectrum between tumor tissues and nor-
mal tissues are small, and prone to noise and varia-
tions across patients, building a robust spatial struc-
ture descriptor is important. One of the results of
our study points to the fact that the tissue structures
surrounding a tumor is a key feature. There is al-
ready evidence that in the micro-scale these normal
and cancerous tissues have a different toplogical ar-
rangement (Dvorak, 2003). This structural informa-
tion of tumor is useful to obtain a better detector for
cancerous tisues in hyperspectral images, since spec-
trum alone is not sufficient to classify them robustly.
Spatio-spectral features have been used in (Lu et al.,
2014), though our aim in the future is to use the spatial
features extracted by scattering-transform (Bruna and
Mallat, 2013) on the different abundance maps ex-
tracted by the hierarchical clustering algorithm. This
enables us to perform a principled search for features
across various scales.
ACKNOWLEDGEMENT
This work has been supported in part by the Euro-
pean Commission through the FP7 FET Open pro-
gramme ICT-2011.9.2, by the European Project HE-
LICoiD “HypErspectral Imaging Cancer Detection”
under Grant Agreement 618080.
REFERENCES
Bruna, J. and Mallat, S. (2013). Invariant scattering con-
volution networks. IEEE Trans. Pattern Anal. Mach.
Intell., 35(8):1872–1886.
Dvorak, H. F. (2003). How tumors make bad blood ves-
sels and stroma. The American journal of pathology,
162(6):1747–1757.
Gillis, N., Kuang, D., and Park, H. (2015). Hierarchi-
cal clustering of hyperspectral images using rank-two
nonnegative matrix factorization. IEEE T. Geoscience
and Remote Sensing, 53(4):2066–2078.
Gillis, N. and Luce, R. (2014). Robust near-separable
nonnegative matrix factorization using linear opti-
mization. Journal of Machine Learning Research,
15:1249–1280.
Kuang, D. (2014). Nonnegative matrix factorization for
clustering. PhD thesis, Georgia Institute of Technol-
ogy.
Kukreti, S., Cerussi, A. E., Tanamai, W., Hsiang, D.,
Tromberg, B. J., and Gratton, E. (2010). Characteriza-
tion of metabolic differences between benign and ma-
lignant tumors: High-spectral-resolution diffuse opti-
cal spectroscopy. Radiology, 254(1):277–284.
Liu, Z., Wang, H., and Li, Q. (2011). Tongue tumor de-
tection in medical hyperspectral images. Sensors,
12(1):162–174.
Lu, G. and Fei, B. (2014). Medical hyperspectral imaging:
a review. Journal of biomedical optics, 19(1):010901–
010901.
Lu, G., Halig, L., Wang, D., Qin, X., Chen, Z. G., and Fei,
B. (2014). Spectral-spatial classification for nonin-
vasive cancer detection using hyperspectral imaging.
Journal of biomedical optics, 19(10):106004–106004.
Ma, W.-K., Bioucas-Dias, J., Chan, T.-H., Gillis, N., Gader,
P., Plaza, A., Ambikapathi, A., and Chi, C.-Y. (2014).
A signal processing perspective on hyperspectral un-
mixing: Insights from remote sensing. Signal Pro-
cessing Magazine, IEEE, 31(1):67–81.
Panasyuk, S., Yang, S., Faller, D., Ngo, D., Lew, R., Free-
man, J., and Rogers, A. (2007). Medical hyperspectral
imaging to facilitate residual tumor identification dur-
ing surgery. Cancer Biol Ther, pages 439–46.
Peswani, D. L. (2007). Detection of Positive Can-
cer Margins Intra-operatively During Nephrectomy
and Prostatectomy Using Optical Reflectance Spec-
troscopy. ProQuest.
Sahu, A., McGoverin, C., Pleshko, N., Sorenmo, K., and
Won, C.-H. (2013). Hyperspectral imaging system
to discern malignant and benign canine mammary tu-
mors. In SPIE Defense, Security, and Sensing, pages
87190W–87190W. International Society for Optics
and Photonics.
Schulder, M. and Carmel, P. W. (2003). Intraoperative
magnetic resonance imaging: impact on brain tumor
surgery. Cancer Control, 10(2):115–124.
Tibshirani, R. and Walther, G. (2005). Cluster validation
by prediction strength. Journal of Computational and
Graphical Statistics, 14(3):511–528.
Utzinger, U., Brewer, M., Silva, E., Gershenson, D., Blast,
R. C., Follen, M., and Richards-Kortum, R. (2001).
Reflectance spectroscopy for in vivo characterization
of ovarian tissue. Lasers in surgery and medicine,
28(1):56–66.
BIOIMAGING 2016 - 3rd International Conference on Bioimaging
84