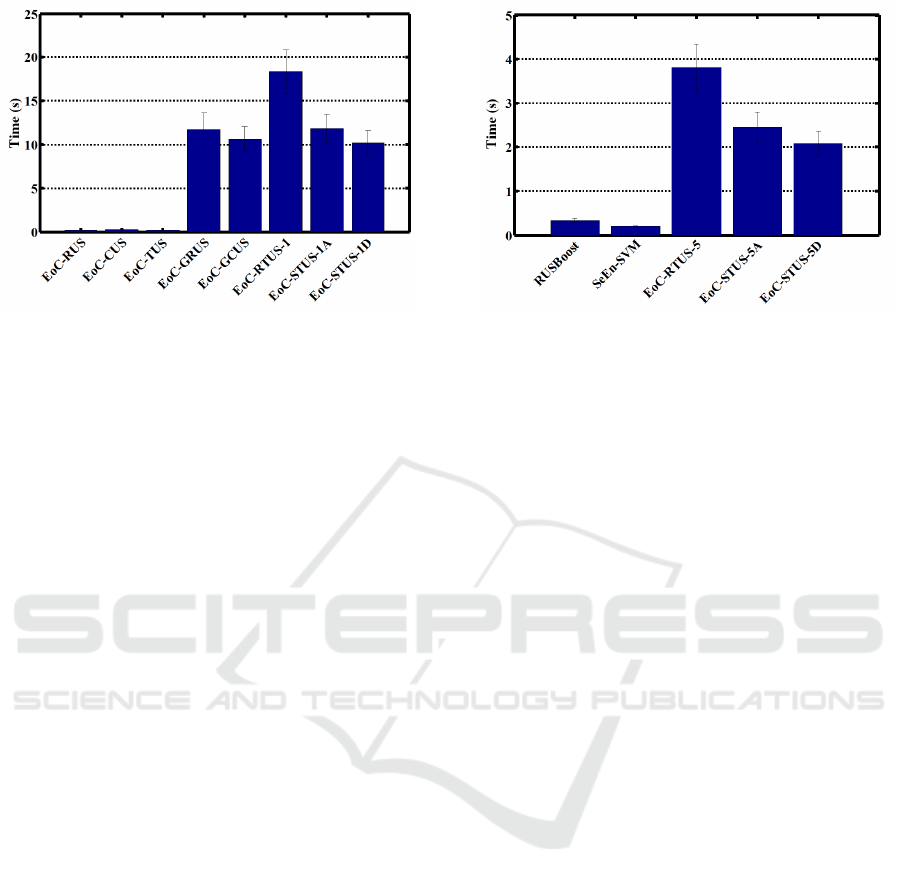
(a) Average training time of ensembles of size N
−
= 100. (b) Average training time of ensembles of size N
e
= 21.
Figure 5: Average of training runtime of proposed and baseline techniques over 10 target individuals and 10 replications.
Dispersion measures are standard errors of the sample mean.
REFERENCES
Bedagkar-Gala, A. and Shah, S. K. (2014). A survey of ap-
proaches and trends in person re-identification. Image
and Vision Computing, 32(4):270–286.
Chang, C.-C. and Lin, C.-J. (2011). Libsvm: a library for
support vector machines. ACM Transactions on Intel-
ligent Systems and Technology, 2(3):27.
Chawla, N. V., Bowyer, K. W., Hall, L. O., and Kegelmeyer,
W. P. (2002). Smote: synthetic minority over-
sampling technique. Journal of artificial intelligence
research, 16(1):321–357.
Chawla, N. V., Lazarevic, A., Hall, L. O., and Bowyer,
K. W. (2003). Smoteboost: Improving prediction of
the minority class in boosting. In Knowledge Discov-
ery in Databases: PKDD 2003, pages 107–119.
De-la Torre, M., Granger, E., Radtke, P. V., Sabourin, R.,
and Gorodnichy, D. O. (2015a). Partially-supervised
learning from facial trajectories for face recognition in
video surveillance. Information Fusion, 24:31–53.
De-la Torre, M., Granger, E., and Sabourin, R. (2015b).
Adaptive skew-sensitive fusion of ensembles and their
application to face re-identification. Pattern Recogni-
tion, 48:3385–3406.
Edgar, G. (2007). Measure, topology, and fractal geometry.
Springer Science & Business Media.
Fawcett, T. (2006). An introduction to roc analysis. Pattern
recognition letters, 27(8):861–874.
Galar, M., Fernandez, A., Barrenechea, E., Bustince, H.,
and Herrera, F. (2012). A review on ensembles for
the class imbalance problem: bagging-, boosting-, and
hybrid-based approaches. Systems, Man, and Cyber-
netics, Part C: Applications and Reviews, IEEE Trans-
actions on, 42(4):463–484.
Goh, R., Liu, L., Liu, X., and Chen, T. (2005). The cmu face
in action (fia) database. In Analysis and Modelling of
Faces and Gestures, pages 255–263.
Kubat, M., Matwin, S., et al. (1997). Addressing the curse
of imbalanced training sets: one-sided selection. In
ICML, volume 97, pages 179–186. Nashville, USA.
Kuncheva, L. I. and Whitaker, C. J. (2003). Measures
of diversity in classifier ensembles and their relation-
ship with the ensemble accuracy. Machine learning,
51(2):181–207.
Li, Q., Yang, B., Li, Y., Deng, N., and Jing, L. (2013). Con-
structing support vector machine ensemble with seg-
mentation for imbalanced datasets. Neural Computing
and Applications, 22(1):249–256.
Li, X., Wang, L., and Sung, E. (2008). Adaboost with
svm-based component classifiers. Engineering Appli-
cations of Artificial Intelligence, 21(5):785–795.
Liu, Y., Yu, X., Huang, J. X., and An, A. (2011). Combining
integrated sampling with svm ensembles for learning
from imbalanced datasets. Information Processing &
Management, 47(4):617–631.
Ojala, T., Pietikainen, M., and Maenpaa, T. (2002). Mul-
tiresolution gray-scale and rotation invariant texture
classification with local binary patterns. Pattern Anal-
ysis and Machine Intelligence, IEEE Transactions on,
24(7):971–987.
Pagano, C., Granger, E., Sabourin, R., Marcialis, G., and
Roli, F. (2014). Adaptive ensembles for face recogni-
tion in changing video surveillance environments. In-
formation Sciences, 286:75–101.
Radtke, P. V., Granger, E., Sabourin, R., and Gorodnichy,
D. O. (2014). Skew-sensitive boolean combination for
adaptive ensembles–an application to face recognition
in video surveillance. Information Fusion, 20:31–48.
Rokach, L. (2009). Taxonomy for characterizing ensemble
methods in classification tasks: A review and anno-
tated bibliography. Computational Statistics & Data
Analysis, 53(12):4046–4072.
Rokach, L. (2010). Ensemble-based classifiers. Artificial
Intelligence Review, 33(1-2):1–39.
Sammon, J. W. (1969). A nonlinear mapping for data
structure analysis. IEEE Transactions on computers,
18(5):401–409.
Classifier Ensembles with Trajectory Under-Sampling for Face Re-Identification
107