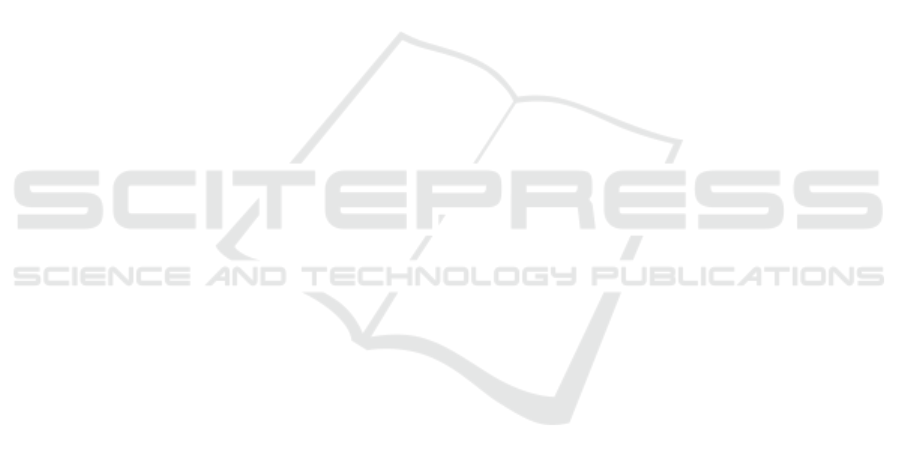
REFERENCES
Anjum, A. and Ilyas, M. U. (2013). Activity recognition us-
ing smartphone sensors. Consumer Communications
and Networking Conference (CCNC), 2013 IEEE.
Bianchi, F., Redmond, S. J., Narayanan, M. R., Cerutti,
S., and Lovell, N. H. (2010). Barometric pressure
and triaxial accelerometry-based falls event detection.
Neural Systems and Rehabilitation Engineering, IEEE
Transactions on, 18(6):619–627.
Cabuz, E. I., Cabuz, C., and Wang, T.-Y. (2009). Piezore-
sistive pressure sensor. US Patent 7,546,772.
Gomes, A. L. (2014). Human activity recognition with ac-
celerometry: Novel time and frequency features. Mas-
ter’s thesis, Faculdade de Ci
ˆ
encias e Tecnologia da
Universidade Nova de Lisboa.
Grankin, M., Khavkina, E., and Ometov, A. (2012). Re-
search of mems accelerometers features in mobile
phones. In Proceedings of the 12th conference of
Open Innovations Association FRUCT; Oulu, Fin-
land, pages 31–36.
Karantonis, D., Narayanan, M., Mathie, M., Lovell, N., and
Celler, B. (2006). Implementation of a real-time hu-
man movement classifier using a triaxial accelerome-
ter for ambulatory monitoring. IEEE Transactions on
Information Technology in Biomedicine, 10.
Kavanagh, J. and Menz, H. (2008). Accelerometry: A tech-
nique for quantifying movement patterns during walk-
ing. ScienceDirect, Gait & Posture 28, 28.
Kotsiantis, S. B., Zaharakis, I., and Pintelas, P. (2007). Su-
pervised machine learning: A review of classification
techniques.
Li, N., Hou, Y., and Huang, Z. (2013). Implementation of
a real-time human activity classifier using a triaxial
accelerometer and smartphone. International Journal
of Advancements in Computing Technology, 5(4).
Machado, I. (2013). Human activity data discovery based
on accelerometry. Master’s thesis, Faculdade de
Ci
ˆ
encias e Tecnologias da Universidade de Lisboa and
PLUX Wireless Biosignals.
Mathie, M. J., Coster, A. C., Lovell, N. H., and Celler,
B. G. (2004). Accelerometry: providing an integrated,
practical method for long-term, ambulatory monitor-
ing of human movement. Physiological measurement,
25(2):R1.
Moncada-Torres, A., Leuenberger, K., Gonzenbach, R.,
Luft, A., and Gassert, R. (2014). Activity classifica-
tion based on inertial and barometric pressure sensors
at different anatomical locations. Physiological mea-
surement, 35(7):1245.
Muralidharan, K., Khan, A. J., Misra, A., Balan, R. K., and
Agarwal, S. (2014). Barometric phone sensors: more
hype than hope! In Proceedings of the 15th Work-
shop on Mobile Computing Systems and Applications,
page 12. ACM.
Peeters, G. (2004). A large set of audio features for
sound description (similarity and classification) in the
cuidado project.
Reddy, S., Mun, M., Burke, J., Estrin, D., Hansen, M., and
Srivastava, M. (2010). Using mobile phones to deter-
mine transportation modes. ACM Trans. Sen. Netw.,
6(2):13:1–13:27.
Rodrigues, C. (2015). Smartphone-based inertial naviga-
tion system for bicycles. Master’s thesis, Faculdade
de Engenharia da Universidade do Porto.
Silva, J. R. C. (2013). Smartphone based human activity
prediction. Master’s thesis, Faculdade de Engenharia
da Universidade do Porto.
Trier, Ø. D., Jain, A. K., and Taxt, T. (1996). Feature extrac-
tion methods for character recognition-a survey. Pat-
tern recognition, 29(4):641–662.
Ture, M., Tokatli, F., and Kurt, I. (2009). Using kaplan–
meier analysis together with decision tree methods
(c&rt, chaid, quest, c4. 5 and id3) in determining
recurrence-free survival of breast cancer patients. Ex-
pert Systems with Applications, 36(2):2017–2026.
Xiao, L., He, B., Koster, A., Caserotti, P., Lange-Maia,
B., Glynn, N. W., Harris, T., and Crainiceanu, C. M.
(2014). Movement prediction using accelerometers in
a human population.
Body Location Independent Activity Monitoring
197