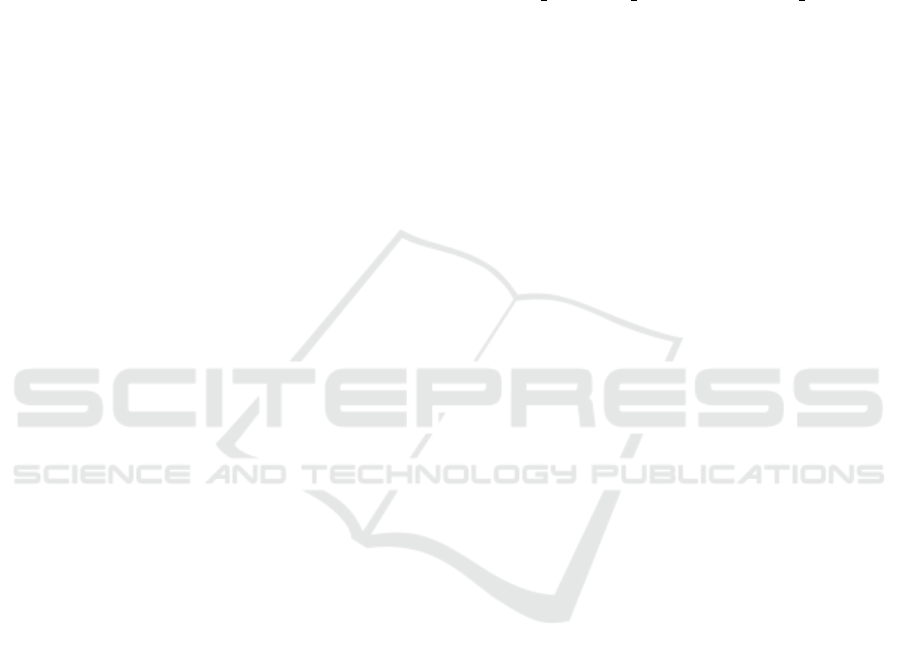
cept for the Semantic Navigator as this tool solely rep-
resents the relationships stored in the UMLS. Occa-
sionally, the statement related information might also
include aggregates of the provenance data, such as the
number of sentences and publications from which a
particular statement is derived, as is the case for Se-
mantic MEDLINE and BioMed Xplorer. Whereas the
BioMed Xplorer is on par with the other tools in re-
lation to the presentation of statement related infor-
mation and the incorporation of provenance data, it
in fact improves over these tools on the presentation
of concepts related information. The concepts related
information presented in BioMed Xplorer UI extends
well beyond the conventional information that is in-
corporated. While, tools such as EBIMed and the Se-
mantic Navigator do not present any of such concepts
related information at all, data items such as (seman-
tic) types, synonyms, and parts of publications that
mention the particular concept are presented by Al-
iBaba, PGviewer, and Semantic MEDLINE. BioMed
Xplorer extends this further through the incorporation
of a wide range of cross-identifiers of concepts, a def-
inition, and a range of data items pertaining to the
clinical features, diagnosis, inheritance, pathogenesis,
and genetics of a disease from OMIM, if available.
The presentation of this wide range of concept related
information in BioMed Xplorer is partially facilitated
through the incorporation of data from external (struc-
tured) data sources, including Linked Life Data and
Bio2RDF, which demonstrates its superiority com-
pared to the other tools developed in prior work.
Among these other tools, the incorporation of infor-
mation from such external sources is either largely
absent (such as in Semantic MEDLINE and Seman-
tic Navigator), or limited to the inclusion of informa-
tion from PubMed (such as in AliBaba, EBIMed, and
PGviewer). Links to external data sources, usually
in the form of cross-references to standardized termi-
nologies, on the other hand, are commonly used by
the tools developed in prior work, with only PGviewer
and the Semantic Network lacking such cross refer-
ences.
For the validation of the ontology, the ontology
developed by (Tao et al., 2012) is considered as the
base to which our developed ontology is compared.
A comparison of the characteristics of the two ontolo-
gies is provided in Table 1. As is clear from this table,
the ontology developed in this research improves the
ontology developed by (Tao et al., 2012) on a number
of aspects, which will be further discussed below, as
such contributing to the validation of the ontology de-
veloped in this research. As was discussed in section
3.1, reification has been applied in both ontologies to
allow triples to involve a particular (bio)medical state-
ment, as a whole, into another statement, and thus
enable meta-statements: statements about statements.
This can be achieved by treating a statement, relat-
ing two (bio)medical concepts to each other through
a relation, as a separate entity to which the subject,
the predicate, and the object of the original statement
are assigned using an object property. The ontology
developed by (Tao et al., 2012) performs this by mak-
ing use of the Association class in combination with
the hass name, has predicate, and haso name prop-
erties. BioMed Xplorer Ontology, on the other hand,
makes use of the official RDF reification vocabulary
that uses the rdf:Statement class in combination with
the rdf:subject, rdf:predicate, and rdf:object proper-
ties. Additionally, the use of this official RDF reifi-
cation vocabulary also contributes to the reuse of ex-
isting vocabularies, one of the key principles of Se-
mantic Web (Shadbolt et al., 2006). To further pro-
mote this base principle of the Semantic Web, the de-
veloped ontology, in addition to the use of the RDF
reification vocabulary, makes extensive use of exist-
ing classes and properties from other vocabularies.
This is a considerable improvement over the ontology
developed by (Tao et al., 2012), as the reuse of exist-
ing vocabularies, aside from the RDF vocabulary, is
not present in their ontology.
Since the purpose of the developed model is to en-
able researchers to explore the body of (bio)medical
knowledge as well as its (disease) related information,
the amount of information captured by the ontology
is of great importance. To this extent, the ontology
developed by (Tao et al., 2012) can be considered as
rather limited due to the fact that there is no direct
evidence of the incorporation of any (disease) related
information beyond the three datatype properties as-
signing names to concepts and relations, as well as
identifiers to publications. The ontology developed in
our research improves on this point by associating 17
data-type properties to the ontology classes that can
be used to capture a wide range of (disease) related
information. This is further facilitated by the incor-
poration of RDF links to the equivalent resources in
external data sources, including Linked Life Data and
Bio2RDF, which contain a wealth of (disease) related
information. No such links are incorporated in the on-
tology developed by (Tao et al., 2012).
Finally, the developed ontology extends the ontol-
ogy developed by (Tao et al., 2012) by incorporat-
ing the sentences from which the represented state-
ments are derived, in addition to those publications
from which these sentences are a part, as a compo-
nent of the provenance data that is associated to the
statements. The incorporation of these sentences pro-
vides additional value to the disease related informa-
BIOINFORMATICS 2016 - 7th International Conference on Bioinformatics Models, Methods and Algorithms
60