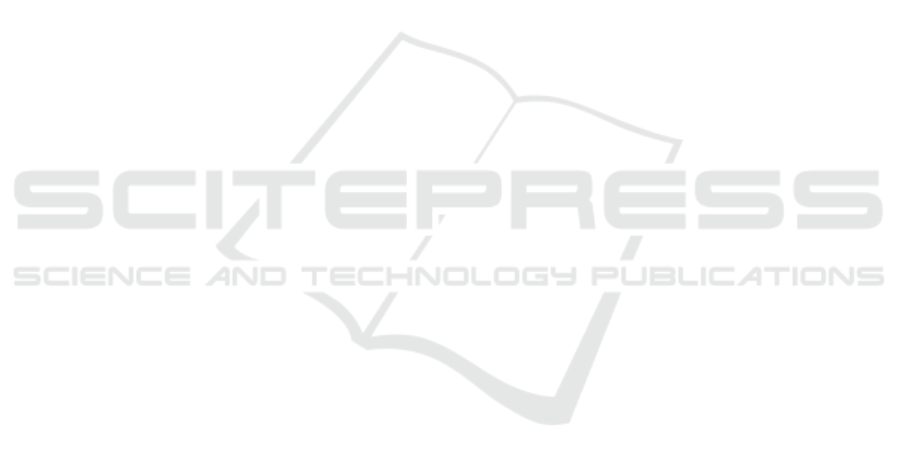
dataset, in order to provide a chance for future com-
parison. The obtained results particularly highlight
the advantage of balancing the training set over using
the original data, particularly for the methods based
on US (NM2, NCR) and combination of OS and US in
feature space. Furthermore, OS in data space outper-
forms the techniques performing in the feature space.
This study also showed that combining color and tex-
ture features will lead to better performance.
REFERENCES
Abbasi, N. R., Shaw, H. M., et al. (2004). Early diagnosis
of cutaneous melanoma: revisiting the abcd criteria.
Jama, 292(22):2771–2776.
American-Cancer-Society (2014). Cancer facts & figures
2014.
Barata, C., Marques, J. S., and Emre Celebi, M. (2013).
Towards an automatic bag-of-features model for the
classification of dermoscopy images: The influence of
segmentation. In Image and Signal Processing and
Analysis (ISPA), 2013 8th International Symposium
on, pages 274–279. IEEE.
Barata, C., Ruela, M., Francisco, M., Mendonc¸a, T., and
Marques, J. (2014). Two systems for the detection of
melanomas in dermoscopy images using texture and
color features. IEEE Systems Journal,, 8(3):965–979.
Batista, G. E., Bazzan, A. L., and Monard, M. C. (2003).
Balancing training data for automated annotation of
keywords: a case study. In WOB, pages 10–18.
Batista, G. E., Prati, R. C., and Monard, M. C. (2004). A
study of the behavior of several methods for balancing
machine learning training data. ACM Sigkdd Explo-
rations Newsletter, 6(1):20–29.
Breiman, L. (2001). Random forests. Machine learning,
45(1):5–32.
Capdehourat, G., Corez, A., Bazzano, A., and Mus
´
e, P.
(2009). Pigmented skin lesions classification using
dermatoscopic images. In Progress in Pattern Recog-
nition, Image Analysis, Computer Vision, and Appli-
cations, pages 537–544. Springer.
Celebi, M. E., Kingravi, H. A., et al. (2007). A method-
ological approach to the classification of dermoscopy
images. Computerized Medical Imaging and Graph-
ics, 31(6):362–373.
Chawla, N. V., Bowyer, K. W., Hall, L. O., and Kegelmeyer,
W. P. (2002). Smote: synthetic minority over-
sampling technique. Journal of artificial intelligence
research, pages 321–357.
Forsea, A., Del Marmol, V., de Vries, E., Bailey, E., and
Geller, A. (2012). Melanoma incidence and mortal-
ity in europe: new estimates, persistent disparities.
British Journal of Dermatology, 167(5):1124–1130.
Guo, Z. and Zhang, D. (2010). A completed modeling of
local binary pattern operator for texture classification.
IEEE Transactions on Image Processing, 19(6):1657–
1663.
He, H., Garcia, E., et al. (2009). Learning from imbalanced
data. Knowledge and Data Engineering, IEEE Trans-
actions on, 21(9):1263–1284.
Laurikkala, J. (2001). Improving identification of diffi-
cult small classes by balancing class distribution.
Springer.
Mani, I. and Zhang, I. (2003). knn approach to unbalanced
data distributions: a case study involving information
extraction. In Proceedings of Workshop on Learning
from Imbalanced Datasets.
Prati, R. C., Batista, G. E., and Monard, M. C. (2009). Data
mining with imbalanced class distributions: concepts
and methods. In IICAI, pages 359–376.
Rastgoo, M., Garcia, R., Morel, O., and Marzani, F.
(2015a). Automatic differentiation of melanoma from
dysplastic nevi. Computerized Medical Imaging and
Graphics, 43:44–52.
Rastgoo, M., Morel, O., Marzani, F., and Garcia, R.
(2015b). Ensemble approach for differentiation of ma-
lignant melanoma. In The International Conference
on Quality Control by Artificial Vision 2015, pages
953415–953415. International Society for Optics and
Photonics.
Tomek, I. (1976). Two modifications of cnn. IEEE Trans.
Syst. Man Cybern., 6:769–772.
Van De Weijer, J. and Schmid, C. (2006). Coloring local
feature extraction. In Computer Vision–ECCV 2006,
pages 334–348. Springer.
Tackling the Problem of Data Imbalancing for Melanoma Classification
39