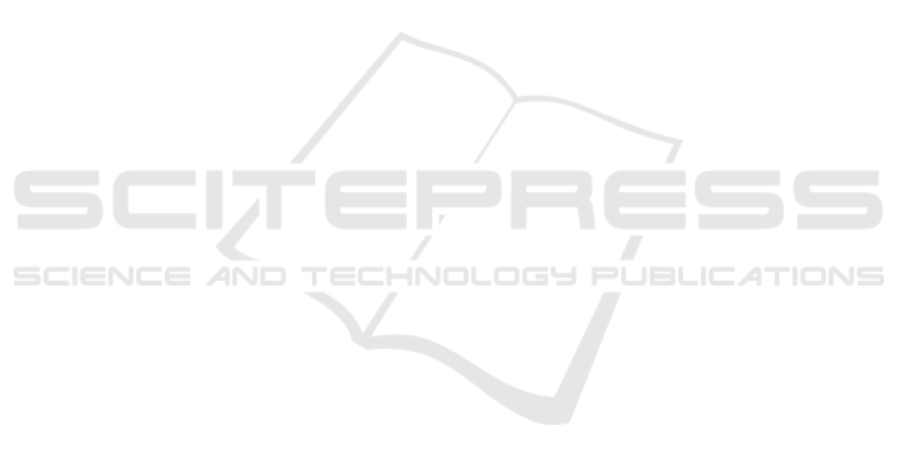
ACKNOWLEDGEMENTS
This work has received supports from the french
Agence Nationale de la Recherche, ASPIQ project
reference ANR-12-BS02-0003. This work has also
received support from the european project H2020
Marie Sklodowska-Curie Actions (MSCA) research
and Innovation Staff Exchange (RISE): AniAge
(High Dimensional Heterogeneous Data based An-
imation Techniques for Southeast Asian Intangible
Cultural Heritage Digital Content), project number
691215.
REFERENCES
Ajroud, A., Omri, M., Youssef, H., and Benferhat, S.
(2012). Loopy belief propagation in bayesian net-
works : origin and possibilistic perspectives. CoRR,
abs/1206.0976.
Amor, N. B., Benferhat, S., Dubois, D., Mellouli, K., and
Prade, H. (2002). A theoretical framework for pos-
sibilistic independence in a weakly ordered setting.
International Journal of Uncertainty, Fuzziness and
Knowledge-Based Systems, 10(2):117–155.
Amor, N. B., Benferhat, S., and Mellouli, K. (2003). Any-
time propagation algorithm for min-based possibilis-
tic graphs. Soft Comput., 8(2):150–161.
Anzilli, L. (2013). A possibilistic approach to invest-
ment decision making. International Journal of Un-
certainty, Fuzziness and Knowledge-Based Systems,
21(02):201–221.
Benferhat, S., Khellaf, F., and Zeddigha, I. (2013). A pos-
sibilistic graphical model for handling decision prob-
lems under uncertainty. In The 8th conference of the
European Society for Fuzzy Logic and Technology,
EUSFLAT, Milano, Italy.
Borgelt, C., Gebhardt, J., and Kruse, R. (1998). Inference
methods. In Handbook of Fuzzy Computation, chapter
F1.2. Institute of Physics Publishing, Bristol, United
Kingdom.
Bouchon-Meunier, B., Coletti, G., and Marsala, C. (2002).
Independence and possibilistic conditioning. Annals
of Mathematics and Artificial Intelligence, 35:107–
123.
Boutouhami, K. and Khellaf, F. (2015). An approximate
possibilistic graphical model for computing optimistic
qualitative decision. In In proceedings of the Interna-
tional Conference on Artificial Intelligence and Appli-
cations AIFU, Dubai UAE, pages 183–196.
de Campos, M. L. and Huete, F. J. (1999). Indepen-
dence concepts in possibility theory. Fuzzy Sets Syst.,
103:487–505.
Denardo, E., Feinberg, E., and Rothblum, U. (2012). Split-
ting in a finite markov decision problem. SIGMET-
RICS Performance Evaluation Review, 39(4):38.
Dubois, D., Berre, D. L., Prade, H., and Sabbadin, R.
(1998). Logical representation and computation of op-
timal decisions in a qualitative setting. In AAAI-98,
pages 588–593.
Dubois, D., Fargier, H., and Prade, H. (2013). Decision-
making under ordinal preferences and comparative
uncertainty. CoRR, abs/1302.1537.
Dubois, D., Godo, L., Prade, H., and Zapico, A. (1999).
On the possibilistic decision model: from decision un-
der uncertainty to case-based decision. International
Journal of Uncertainty, Fuzziness and Knowledge-
Based Systems, 7(6):631–670.
Dubois, D. and Prade, H. (1988). Possibility Theory: An
Approach to Computerized Processing of Uncertainty
. Plenum Press, New York.
Dubois, D. and Prade, H. (1995). Possibility theory as a
basis for qualitative decision theory. In Proceedings
of the 14th international joint conference on Artificial
intelligence - Volume 2, pages 1924–1930, San Fran-
cisco, CA, USA. Morgan Kaufmann Publishers Inc.
Dubois, D., Prade, H., and Sabbadin, R. (2001). Decision-
theoretic foundations of qualitative possibility the-
ory . European Journal of Operational Research,
128(3):459–478.
Garcia, L. and Sabbadin, R. (2006). Possibilistic influence
diagrams. In 17th European Conference on Artifi-
cial Intelligence (ECAI’06), pages 372–376, Riva del
Garda, Italy. IOS Press.
Giang, P. and Shenoy, P. (2005). Two axiomatic approaches
to decision making using possibility theory. European
Journal of Operational Research, 162(2):450–467.
Guezguez, W. (2012). Possibilistic Decision Theory:
From Theoretical Foundations to Influence Diagrams
Methodology. Phd thesis, Paul Sabatier University,
France.
Guezguez, W., Amor, N. B., and Mellouli, K. (2009). Qual-
itative possibilistic influence diagrams based on qual-
itative possibilistic utilities. European Journal of Op-
erational Research, 195(1):223–238.
Howard, R. and Matheson, J. (1984). Influence Diagrams.
In Readings on the Principles and Applications of De-
cision Analysis, pages 721–762. Strategic Decisions
Group.
Huang, C. and Darwiche, A. (1996). Inference in belief net-
works: A procedural guide. Int. J. Approx. Reasoning,
15(3):225–263.
Lauritzen, S. and Nilsson, D. (2001). Representing and
solving decision problems with limited information.
Manage. Sci., 47(9):1235–1251.
Shenoy, P. (1994). A comparison of graphical techniques
for decision analysis. European Journal of Opera-
tional Research, 78:1–21.
Sniedovich, M. (2010). Dynamic Programming: Founda-
tions and Principles; 2nd ed. Pure and Applied Math-
ematics. CRC Press, Hoboken.
Tatman, J. and Shachter, R. (1990). Dynamic programming
and influence diagrams. IEEE Transactions on Sys-
tems, Man and Cybernetics, 20(2):365–379.
Whalen, T. (1984). Decision making under uncertainty
with various assumptions about available informa-
tion. IEEE Trans. on systems, Man and Cybernetics,
(14):888–900.
On the Decomposition of Min-based Possibilistic Influence Diagrams
127