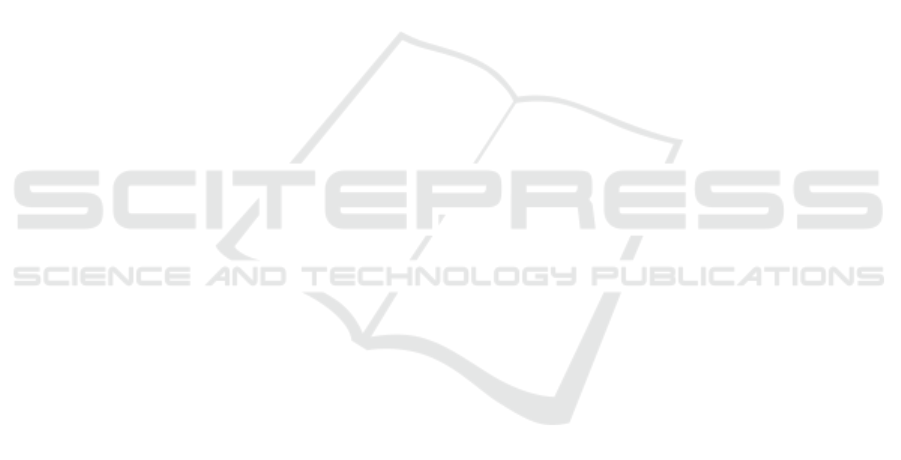
We observe how the performance changes by in-
creasing the number of adaptation utterances; using
only one utterance as the adaptation data decreases
the performance resulting in a higher WER, with two
and more utterances up to 6, the performance gets im-
proved gradually. Afterwards, with more than 6 utter-
ances, no more gain is obtained. The line is expected
to reach the value of 25% (the WER in Table 2) if
the adaptation data was increased. We also observe
that the adapted model reaches the Google ASR per-
formance with two utterances and outperforms it with
more adaptation utterances.
7 CONCLUSIONS
Here we presented a large vocabulary continuous
speech recognition system based on a GMM-HMM
system. We implemented adaptation methods to im-
prove the system. Two methods, lVTLN and cMLLR,
were used for unsupervised acoustic model adapta-
tion. The performance of these systems were com-
pared with the speaker independent system by testing
on the Ester and Etape data sets. The basic model,
which was a triphone model, was improved by apply-
ing SAT and lVTLN/cMLLR. It was shown that the
performance was improved by a relative 9.44 percent
reduction in WER by using cMLLR. In the end the ba-
sic model and the adapted model using cMLLR were
compared with the Google ASR. It was shown that
the adaptation with cMLLR could improve the basic
system to overpass Google ASR.
We also observed in general the system worked
better for the data set including more planned speech.
This shows the importance of a good language model.
Therefore, we believe further gain could be obtained
by improving the language model, e.g. combining the
Google n-gram counts with n-gram language model
from training set using interpolation methods.
REFERENCES
Ahadi, S. and Woodland, P. C. (1997). Combined bayesian
and predictive techniques for rapid speaker adaptation
of continuous density hidden markov models. Com-
puter speech & language, 11(3):187–206.
Allauzen, C., Riley, M., Schalkwyk, J., Skut, W., and
Mohri, M. (2007). Openfst: A general and efficient
weighted finite-state transducer library. In Imple-
mentation and Application of Automata, pages 11–23.
Springer.
Anastasakos, T., McDonough, J., Schwartz, R., and
Makhoul, J. (1996). A compact model for speaker-
adaptive training. In Spoken Language, 1996. ICSLP
96. Proceedings., Fourth International Conference on,
volume 2, pages 1137–1140. IEEE.
Chen, S. F. and Goodman, J. (1999). An empirical study of
smoothing techniques for language modeling. Com-
puter Speech & Language, 13(4):359–393.
Davis, S. B. and Mermelstein, P. (1980). Comparison
of parametric representations for monosyllabic word
recognition in continuously spoken sentences. Acous-
tics, Speech and Signal Processing, IEEE Transac-
tions on, 28(4):357–366.
Eide, E. and Gish, H. (1996). A parametric approach to vo-
cal tract length normalization. In Acoustics, Speech,
and Signal Processing, 1996. ICASSP-96. Conference
Proceedings., 1996 IEEE International Conference
on, volume 1, pages 346–348. IEEE.
Galliano, S., Geoffrois, E., Gravier, G., Bonastre, J.-F.,
Mostefa, D., and Choukri, K. (2006). Corpus descrip-
tion of the ester evaluation campaign for the rich tran-
scription of french broadcast news. In Proceedings of
LREC, volume 6, pages 315–320.
Galliano, S., Gravier, G., and Chaubard, L. (2009). The
ester 2 evaluation campaign for the rich transcription
of french radio broadcasts. In Interspeech, volume 9,
pages 2583–2586.
Gauvain, J.-L. and Lee, C.-H. (1994). Maximum a poste-
riori estimation for multivariate gaussian mixture ob-
servations of markov chains. Speech and audio pro-
cessing, ieee transactions on, 2(2):291–298.
Gravier, G., Adda, G., Paulson, N., Carr
´
e, M., Giraudel,
A., and Galibert, O. (2012). The etape corpus for
the evaluation of speech-based tv content processing
in the french language. In LREC-Eighth international
conference on Language Resources and Evaluation,
page na.
Kumar, N. and Andreou, A. G. (1998). Heteroscedastic
discriminant analysis and reduced rank hmms for im-
proved speech recognition. Speech communication,
26(4):283–297.
Leggetter, C. J. and Woodland, P. C. (1995). Maximum
likelihood linear regression for speaker adaptation of
continuous density hidden markov models. Computer
Speech & Language, 9(2):171–185.
Povey, D., Ghoshal, A., Boulianne, G., Burget, L., Glem-
bek, O., Goel, N., Hannemann, M., Motl
´
ı
ˇ
cek, P., Qian,
Y., Schwarz, P., et al. (2011). The kaldi speech recog-
nition toolkit.
Prasad, N. V. and Umesh, S. (2013). Improved cep-
stral mean and variance normalization using bayesian
framework. In Automatic Speech Recognition and Un-
derstanding (ASRU), 2013 IEEE Workshop on, pages
156–161. IEEE.
Shinoda, K. and Lee, C.-H. (1997). Structural map speaker
adaptation using hierarchical priors. In Automatic
Speech Recognition and Understanding, 1997. Pro-
ceedings., 1997 IEEE Workshop on, pages 381–388.
IEEE.
Stolcke, A. et al. (2002). Srilm-an extensible language mod-
eling toolkit. In INTERSPEECH.
ICPRAM 2016 - International Conference on Pattern Recognition Applications and Methods
282