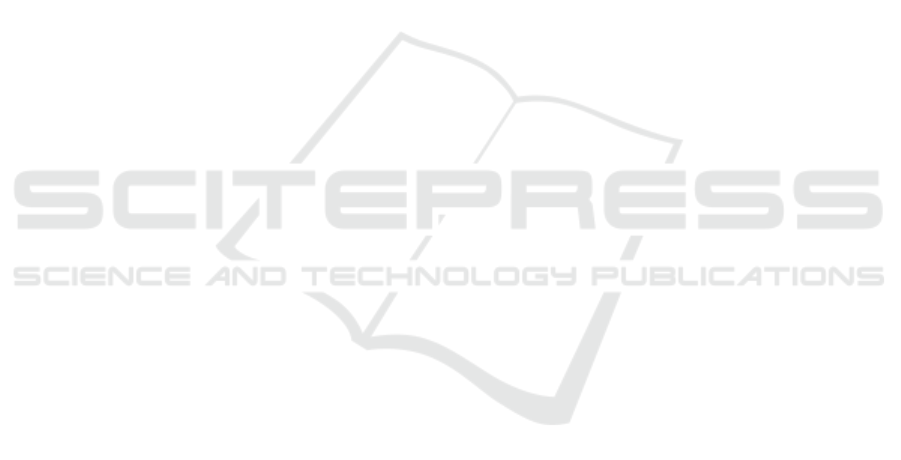
Kappel reported 98.4 % correct localizations in case
of WiFi (Kappel, 2014). In a second test series, we
tried to identify smaller regions with BLE. In these
experiments, the best result was 77.7% correct local-
izations.
Another aspect for the usability of the presented
approach is the setup time of the beacon infrastruc-
ture. While it is short compared with fingerprinting
or parameter tuning for model-based algorithms, the
effort for beacon positioning and test measurements
is not neglectable.
In our use case, the caretakers are notified when a
demented and disoriented person leaves the safe area.
For sending notification messages, the BLE infras-
tructure is not suited. Hence, the existing WiFi infras-
tructure of the building or SMS messages via GSM
have to be used.
In (Fudickar et al., 2011) a dynamic localization
interval is motivated. For example, a resident may
have lunch and is not moving. In this situation, the
localization interval may be increased for further en-
ergy savings. Therefore, we will combine sensor data
from the smartphone’s accelerometer which are al-
ready used for fall detection with the localization sys-
tem. Further, we will use thresholds for the RSS val-
ues and investigate their influence on the positioning
accuracy.
REFERENCES
Bahl, P. and Padmanabhan, V. N. (2000). RADAR: an in-
building RF-based user location and tracking system.
Proceedings IEEE INFOCOM 2000 Conference on
Computer Communications Nineteenth Annual Joint
Conference of the IEEE Computer and Communica-
tions Societies, 2(c):775–784.
Behnke, R. and Timmermann, D. (2008). AWCL: Adap-
tive Weighted Centroid Localization as an efficient
Improvement of Coarse Grained Localization. In 5th
Workshop on Positioning Navigation and Communi-
cation, pages 243–250.
Dementyev, A., Hodges, S., Taylor, S., and Smith, J. (2013).
Power consumption analysis of bluetooth low energy,
zigbee, and ant sensor nodes in a cyclic sleep scenario.
In Proceedings of IEEE International Wireless Sym-
posium (IWS), pages 1–4. IEEE.
Faragher, R. and Harle, R. (2014). An analysis of the ac-
curacy of bluetooth low energy for indoor positioning
applications. pages 201–210, Tampa, Florida, USA.
Fink, A., Beikirch, H., and Voss, M. (2010). Improved In-
door Localization with diversity and filtering based on
received signal strength measurements. Computing,
9(1):9–15.
Fudickar, S., Schnor, B., Felber, J., Neyer, F. J., Lenz, M.,
and Stede, M. (2011). KopAL - An Orientation Sys-
tem For Patients With Dementia. In Gottfried, B. and
Aghajan, H., editors, Behaviour Monitoring and In-
terpretation - BMI, volume 9 of Ambient Intelligence
and Smart Environments, pages 83–104. IOS Press.
ISBN: 978-1-60750-730-7.
Fudickar, S. and Valentin, M. (2014a). Comparing Suit-
ability of Sub 1 GHz and WiFi Transceivers for RSS-
based Indoor Localisation. In 5th International Con-
ference on Indoor Positioning and Indoor Navigation
(IPIN), Busan, Korea.
Fudickar, S. and Valentin, M. (2014b). Most Accurate Al-
gorithms for RSS-based Wi-Fi Indoor Localisation. In
5th International Conference on Indoor Positioning
and Indoor Navigation (IPIN), Busan, Korea.
Gansemer, S., Pueschel, S., Frackowiak, R., Hakobyan, S.,
and Grossmann, U. (2010). Improved RSSI-based Eu-
clidean Dustance Positioning Algorithm for large and
Dynamic WLAN Environments. Computing, 9(1):37–
44.
Gimpel, T., Kiertscher, S., Lindemann, A., Schnor, B., and
Vogel, P. (2015). Evaluation of Threshold-based Fall
Detection on Android Smart Phones. In In Proceed-
ings of the 8th International Conference on Health In-
formatics (HEALTHINF), Lisbon, Portugal.
ITU (2012). Propagation data and prediction methods for
the planning of indoor radio communication systems
and the radio local area networks in the frequency
range 900 MHz to 100 GHz (ITU-T Recommendation
P.1238-7). International Telecommunications Union,
Geneva, Switzerland.
Jianyong, Z., Zili, C., Haiyong, L., and Zhaohui, L. (2014).
RSSI Based Bluetooth Low Energy Indoor Position-
ing. In Proceedings of the 5th International Con-
ference on Indoor Positioning and Indoor Navigation
2014 (IPIN 2014).
Kappel, M. (2014). Indoor-Lokalisierung mit Android
basierten Smartphones. Bachelor thesis, University of
Potsdam.
Scheffler, T., Schindler, S., and Schnor, B. (2013). Using
AOP-based Enforcement of Prioritized XACML Poli-
cies for Location Privacy. International Journal of In-
ternet Technology and Secured Transactions, 5(1):84–
104.
Schindler, S. (2011). Location Privacy. Diploma thesis,
University of Potsdam.
Indoor Positioning: A Comparison of WiFi and Bluetooth Low Energy for Region Monitoring
321