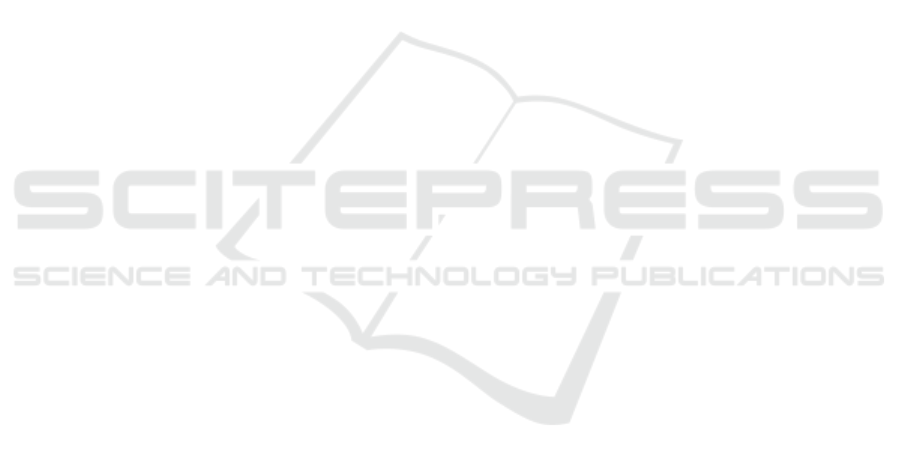
5 CONCLUSIONS
This paper explored the possibility of using the top-k
part-of-speech sequential patterns of variable length
as a feature for authorship attribution. The proposed
approach discovers sequential patterns of parts of
speech to build signatures representing each author’s
writing style. It then uses them to perform automatic
authorship attribution. An experimental evaluation
using 30 books and 10 authors from Project Guten-
berg was carried. Results show that authors can be
accurately classified with more than 70% accuracy us-
ing a very small number of variable-length patterns
(e.g. k = 50). The proposed approach was also shown
to perform better using a small amount of variable-
length patterns than with many fixed-length patterns
such as POS bigrams and trigrams. Our future work
experiments with blog texts, which have a very differ-
ent general style.
ACKNOWLEDGEMENTS
This work is financed by a National Science and En-
gineering Research Council (NSERC) of Canada re-
search grant, and the Faculty of Research and Gradu-
ate Studies of the Universit
´
e de Moncton.
REFERENCES
Agrawal, R. and Srikant, R. (1995). Mining sequential pat-
terns. In Proc. of the Eleventh Intern. Conf. on Data
Engineering, 1995., pages 3–14. IEEE.
Argamon-Engelson, S., Koppel, M., and Avneri, G. (1998).
Style-based text categorization: What newspaper am i
reading. In Proc. of the AAAI Workshop on Text Cate-
gorization, pages 1–4.
Baayen, H., Van Halteren, H., and Tweedie, F. (1996). Out-
side the cave of shadows: Using syntactic annotation
to enhance authorship attribution. Literary and Lin-
guistic Computing, 11(3):121–132.
Boukhaled, M. A. and Ganascia, J.-G. (2015). Using func-
tion words for authorship attribution: Bag-of-words
vs. sequential rules. Natural Language Processing
and Cognitive Science: Proc. 2014, page 115.
Clark, J. H. and Hannon, C. J. (2007). A classifier system
for author recognition using synonym-based features.
In MICAI 2007: Advances in Artificial Intelligence,
pages 839–849. Springer.
Fournier-Viger, P., Gomariz, A., Gueniche, T., , E., and
Thomas, R. (2013). Tks: Efficient mining of top-k se-
quential patterns. In Advanced Data Mining and Ap-
plications, pages 109–120. Springer.
Gamon, M. (2004). Linguistic correlates of style: author-
ship classification with deep linguistic analysis fea-
tures. In Proc. of the 20th international conference
on Computational Linguistics, page 611. ACL.
Garc
´
ıa-Hern
´
andez, R. A., Mart
´
ınez-Trinidad, J. F., and
Carrasco-Ochoa, J. A. (2010). Finding maximal se-
quential patterns in text document collections and sin-
gle documents. Informatica, 34(1).
Han, J., Kamber, M., and Pei, J. (2011). Data mining: con-
cepts and techniques. Elsevier.
Howe, D. C. (2009). Rita: creativity support for computa-
tional literature. In Proc. of the 7th ACM conference
on Creativity and cognition, pages 205–210. ACM.
Koppel, M. and Schler, J. (2003). Exploiting stylistic id-
iosyncrasies for authorship attribution. In Proc. of
IJCAI’03 Workshop on Computational Approaches to
Style Analysis and Synthesis, volume 69, page 72.
Koppel, M., Schler, J., and Argamon, S. (2013). Authorship
attribution: What’s easy and what’s hard? Available
at SSRN 2274891.
Koppel, M., Schler, J., and Bonchek-Dokow, E. (2007).
Measuring differentiability: Unmasking pseudony-
mous authors. Journal of Machine Learning Research,
8(2):1261–1276.
Litvinova, T., Seredin, P., and Litvinova, O. (2015). Using
part-of-speech sequences frequencies in a text to pre-
dict author personality: a corpus study. Indian Journal
of Science and Technology, 8(S9):93–97.
McDonald, A. W., Afroz, S., Caliskan, A., Stolerman, A.,
and Greenstadt, R. (2012). Use fewer instances of the
letter i: Toward writing style anonymization. In Pri-
vacy Enhancing Technologies, pages 299–318.
Mendenhall, T. C. (1887). The characteristic curves of com-
position. Science, pages 237–249.
Mosteller, F. and Wallace, D. (1964). Inference and dis-
puted authorship: The federalist.
Mwamikazi, E., Fournier-Viger, P., Moghrabi, C., and Bau-
douin, R. (2014). A dynamic questionnaire to further
reduce questions in learning style assessment. In Arti-
ficial Intelligence Applications and Innovations, pages
224–235. Springer.
Sidorov, G., Velasquez, F., Stamatatos, E., Gelbukh, A.,
and Chanona-Hern
´
andez, L. (2014). Syntactic n-
grams as machine learning features for natural lan-
guage processing. Expert Systems with Applications,
41(3):853–860.
Stamatatos, E., Fakotakis, N., and Kokkinakis, G. (2000).
Automatic text categorization in terms of genre and
author. Computational linguistics, 26(4):471–495.
Stamatatos, E., Fakotakis, N., and Kokkinakis, G. (2001).
Computer-based authorship attribution without lexical
measures. Computers and the Humanities, 35(2):193–
214.
Toutanova, K., Klein, D., Manning, C. D., and Singer, Y.
(2003). Feature-rich part-of-speech tagging with a
cyclic dependency network. In Proc. of the 2003 Conf.
of the North American Chapter of the ACL on Human
Language Technology-Vol. 1, pages 173–180. ACL.
Yule, G. U. (1939). On sentence-length as a statistical
characteristic of style in prose: With application to
two cases of disputed authorship. Biometrika, 30(3-
4):363–390.
Authorship Attribution using Variable Length Part-of-Speech Patterns
361