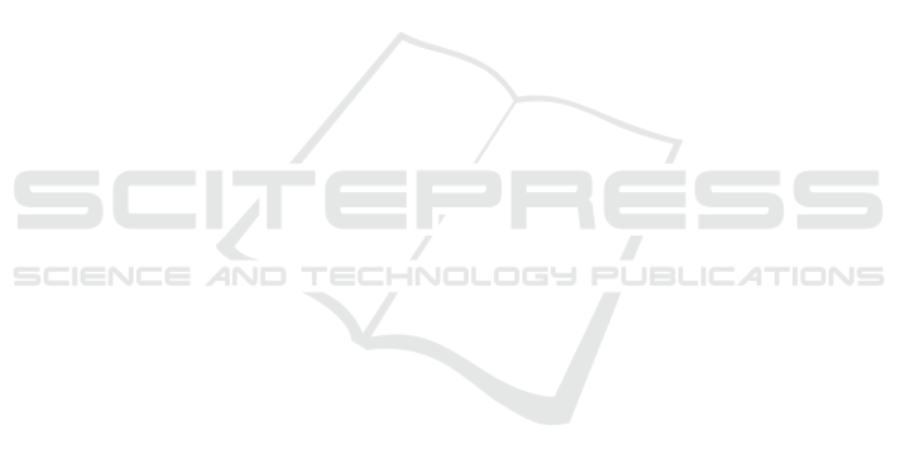
camera allowing less constraining 3D acquisition in
order to approximate more realistic conditions. We
consider also, various expressions, poses and illu-
minations settings. The database is freely available
for research purposes and can be requested at URL
http://www.lifl.fr/FOX/index.php?page=datasets.
The paper is structured as follows. In Section
2, we introduce major datasets in the field of per-
son recognition covering head pose, gender and facial
expressions variations and using 2D, depth, stereo,
or 3D data, emphasizing the need for constructing a
novel dataset narrowing the gap between lab condi-
tions and wild settings. The methodology employed
to design and collect the dataset is described in details
in Section 3. Some experiments, in a face recognition
context, are presented in Section 4 before summariz-
ing the contributions and the new challenges related
to the proposed dataset in Section 5.
2 RELATED DATASETS
Many public datasets are available for face related re-
searches. Existing resources can be 2D or 3D. 3D
data can be divided into two major categories: data of-
fering a complete 3D face models using 3D scanners
and recently available, the data offering a view-based
3D face models using depth sensors (e.g. Microsoft
Kinect) which is our context of study.
The use of 3D data in conjunction with 2D data
has been broadly spread in face analysis research.
Datasets offering 3D information on faces were there-
fore proposed in order to evaluate algorithms in this
field. Table 1 lists some well-known bimodal 2D-
3D datasets and their specifications. We included the
proposed dataset in the bottom of the table in order
to show its contributions. 3D FRGC (Phillips et al.,
2005) and Texas (Gupta et al., 2010) datasets are
face recognition-oriented with some variations in fa-
cial expressions and illumination. Bosphorus dataset
(Savran et al., 2008) is more general and can be
used in different face analysis tasks. It contains a
large variation of head poses and face expressions.
BU-3DFE dataset (Yin et al., 2006) is expression
recognition-oriented. 7 expression shape models are
collected and a corresponding facial texture image
captured from two views is also given. BU-4DFE
dataset (Yin et al., 2008) is an extended version of
the BU-3DFE dataset offering temporal information
by capturing dynamic 3D data.
The above datasets are made via expensive equip-
ments (3D scanners) offering a high quality data
(complete 3D face models). Hence, they require spe-
cific acquisition conditions: sufficient time for scan-
ning, cooperation of the person to be identified in-
stalled in front of the scanner until the end of scan-
ning.
Recently, in order to extend the scope of 3D, the
research interest focuses increasingly on the use of
less restrictive 3D equipments (e.g. Kinect). In Ta-
ble 1, we include 2 recent datasets obtained via Mi-
crosoft Kinect sensor. BIWI database (Fanelli et al.,
2013) is compound of head movements 3D sequences
of 20 subjects under ambiant lighting and neutral ex-
pression. Eurecom dataset (Min et al., 2014) con-
tains still 2D and 3D images of 52 subjects show-
ing few changes in expression and head pose orien-
tation. Very few datasets acquired with depth sensors
are available and none, to the best of our knowledge,
encompasses all variations. In this paper, we present
a new face dataset which encompasses different data
modalities. This dataset presents 2D, 3D and stereo
images of the face, in order to allow testing and com-
paring face analysis methods in low constrained con-
text. The dataset offers both static and dynamic data.
3D dynamic data, also called 4D data, allows the ex-
tension of studies to a time-varying 3D faces. Addi-
tionally to these aspects, various face expressions and
head poses are taken from 64 subjects. Face analy-
sis can be performed under varying pose, expression
and illumination. In the remainder, we introduce the
dataset and highlight its usefulness for bimodal face
recognition.
3 THE FoxFaces DATASET
3.1 Acquisition Devices
The dataset has been built using an acquisition system
composed with 3 sensors:
• Infrared Sensor: we used Microsoft Kinect, that
contains a color camera, an infrared light, and an
infrared CMOS sensor (QVGA 320x240, 16 bits)
able to generate a depth map of the scene by esti-
mating the amount of reflected infrared light. The
farther an object, the less light it reflects.
• 3D Time-of-Flight Sensor: we have used Mesa
Imaging SR4000 sensor, that flashes the scene
with infrared light pulses,
• Stereo Camera: we used Point Grey Bumblebee
XB3, a multi-baseline sensor equipped with three
1.3 megapixel cameras. The large baseline offers
a higher precision in higher distances from cam-
era, and the small baseline enhances the matching
of small intervals.
VISAPP 2016 - International Conference on Computer Vision Theory and Applications
534