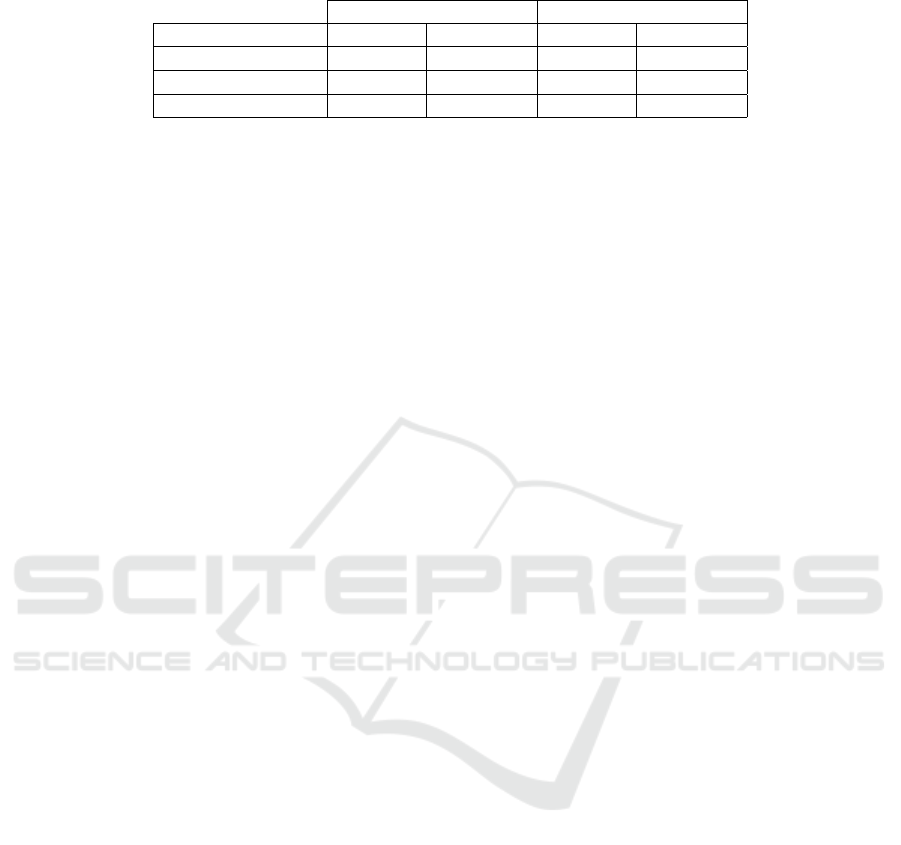
Table 1: MOTA and MOTP comparison.
MOTA MOTP
Sequence Proposed POM-KSP Proposed POM-KSP
Walking 100% 93% 86% 83%
Meeting 98% 54% 77% 75%
Unsteady Lighting 97% 83% 86% 84%
of 20 frames per second if the processing is paral-
lelized in a distributed architecture, i.e., processing
for each camera is implemented as a separate thread
or on a smart camera.
ACKNOWLEDGEMENT
The work was financially supported by FWO through
the project G.0.398.11.N.10 “Multi-camera human
behavior monitoring and unusual event detection”.
REFERENCES
Andriyenko, A. and Schindler, K. (2010). Globally optimal
multi-target tracking on a hexagonal lattice. In Pro-
ceedings of the 11th European Conference on Com-
puter Vision: Part I, ECCV’10, pages 466–479.
Andriyenko, A. and Schindler, K. (2011). Multi-target
tracking by continuous energy minimization. In Com-
puter Vision and Pattern Recognition (CVPR), 2011
IEEE Conference on, pages 1265–1272.
Berclaz, J., Fleuret, F., Turetken, E., and Fua, P. (2011).
Multiple object tracking using k-shortest paths opti-
mization. IEEE Trans. on Pattern Analysis and Ma-
chine Intelligence, 33(9):1806–1819.
Bernardin, K. and Stiefelhagen, R. (2008). Evaluating mul-
tiple object tracking performance: The clear mot met-
rics. J. Image Video Process., 2008:1–10.
Bo Bo, N., Deboeverie, F., Eldib, M., Guan, J., Xie, X.,
Nio, J., Van Haerenborgh, D., Slembrouck, M., Van de
Velde, S., Steendam, H., Veelaert, P., Kleihorst, R.,
Aghajan, H., and Philips, W. (2014). Human mobility
monitoring in very low resolution visual sensor net-
work. Sensors, 14(11):20800–20824.
Bo Bo, N., Deboeverie, F., Veelaert, P., and Philips, W.
(2015). Real-time multi-people tracking by greedy
likelihood maximization. In International Conference
on Distributed Smart Cameras (ICDSC2015), Seville,
Spain.
Bredereck, M., Jiang, X., Korner, M., and Denzler, J.
(2012). Data association for multi-object tracking-by-
detection in multi-camera networks. In Distributed
Smart Cameras (ICDSC), 2012 Sixth International
Conference on, pages 1–6.
Gruenwedel, S., Jela
˘
ca, V., Ni
˜
no Casta
˜
neda, J., Van Hese,
P., Van Cauwelaert, D., Van Haerenborgh, D., Vee-
laert, P., and Philips, W. (2014). Low-complexity
scalable distributed multi-camera tracking of humans.
ACM Transactions on Sensor Networks, 10(2).
Henriques, J. F., Caseiro, R., and Batista, J. (2011). Glob-
ally optimal solution to multi-object tracking with
merged measurements. In IEEE International Con-
ference on Computer Vision, ICCV 2011, Barcelona,
Spain, November 6-13, 2011, pages 2470–2477.
Milan, A., Schindler, K., and Roth, S. (2013). Detection-
and trajectory-level exclusion in multiple object track-
ing. In Computer Vision and Pattern Recognition
(CVPR), 2013 IEEE Conference on, pages 3682–
3689.
Pirsiavash, H., Ramanan, D., and Fowlkes, C. C. (2011).
Globally-optimal greedy algorithms for tracking a
variable number of objects. In Proceedings of the
2011 IEEE Conference on Computer Vision and Pat-
tern Recognition, CVPR ’11, pages 1201–1208.
Wojek, C., Roth, S., Schindler, K., and Schiele, B. (2010).
Monocular 3d scene modeling and inference: Under-
standing multi-object traffic scenes. In Proceedings of
the 11th European Conference on Computer Vision:
Part IV, ECCV’10, pages 467–481.
Yang, J., Vela, P. A., Shi, Z., and Teizer, J. (2009). Proba-
bilistic multiple people tracking through complex sit-
uations. In 11th IEEE International Workshop on
PETS, pages 79–86.
Yun, Y., Gu, I.-H., and Aghajan, H. (2012). Maximum-
likelihood object tracking from multi-view video by
combining homography and epipolar constraints. In
The 2012 Sixth International Conference on Dis-
tributed Smart Cameras (ICDSC).
Zhang, L., Li, Y., and Nevatia, R. (2008). Global data asso-
ciation for multi-object tracking using network flows.
In Computer Vision and Pattern Recognition, 2008.
CVPR 2008. IEEE Conference on.
Multiple People Tracking in Smart Camera Networks by Greedy Joint-Likelihood Maximization
611