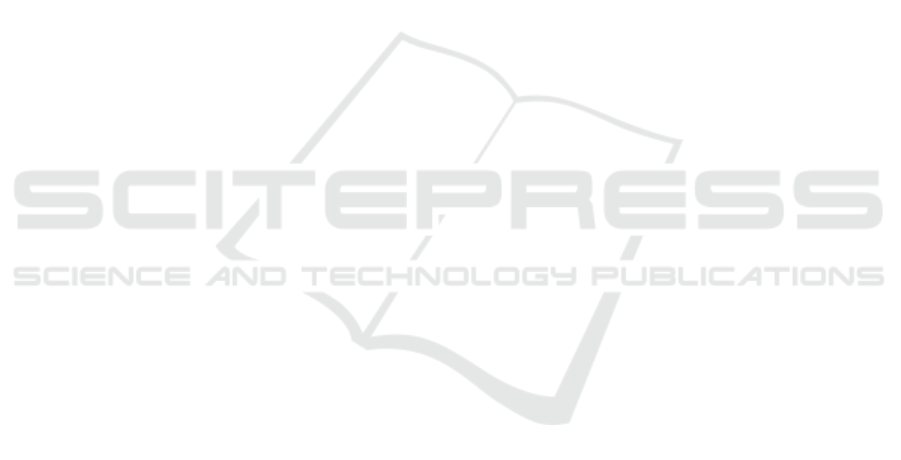
of intensity-based AAM cover a large span of val-
ues and that similar USM-based approaches lead to
significantly different results suggests that intensity-
based AAM and the analyzed preprocessing filters
lack robustness and are prone to the bias introduced
by initialization and preprocessing parameters. On
the contrary, the two analyzed feature-based AAM
proved to be more robust. Using DSIFT and HOG
to train the model drastically improves fitting perfor-
mance regardless of preprocessing; the fact that the
results do not differ significantly for all preprocess-
ing algorithms shows that the extracted features de-
scribe image content very robustly. The results sug-
gest that using preprocessing for feature-based AAM
does not result in a significant performance increase
and that the preprocessing step can be omitted, espe-
cially when considering the additional computational
requirements to run the preprocessing filter.
Although quantitative analysis has shown a mea-
surable difference in fitting performance on seen and
unseen images the ability of the AAM to model un-
trained faces still allows for precise landmark detec-
tion in unseen images. The model has been shown
to be robust enough to track an unseen face during a
series of challenging head pose changes in a video se-
quence with the ability to recover even after phases of
fast head movement or extreme out-of-plane rotation.
6 CONCLUSION
In this paper we have shown that AAMs are a vi-
able approach for face tracking in the thermal in-
frared domain. Using a suitable database and a
well-performing combination of algorithms compris-
ing DSIFT for modeling and SIC for fitting yields sta-
ble and robust results. It has been shown that AAMs
can be used for robust single-frame initialized LWIR
face tracking.
REFERENCES
Alabort-I-medina, J., Antonakos, E., Booth, J., Snape, P.,
and Zafeiriou, S. (2014). Menpo: A comprehensive
platform for parametric image alignment and visual
deformable models. In ACM International Confer-
ence on Multimedia, MM ’14, pages 679–682, Or-
lando, Florida, USA. ACM.
Alkali, A. H., Saatchi, R., Elphick, H., and Burke, D.
(2014). Eyes’ corners detection in infrared images for
real-time noncontact respiration rate monitoring. In
WCCAIS 2014, pages 1–5. IEEE.
Antonakos, E., i medina, J. A., Tzimiropoulos, G., and
Zafeiriou, S. (2015). Feature-based lucas-kanade and
active appearance models. IEEE Transactions on Im-
age Processing, 24(9):2617–2632.
Cootes, T. F., Edwards, G. J., and Taylor, C. J. (2001). Ac-
tive appearance models. IEEE PAMI, 23(6):681–685.
Dowdall, J., Pavlidis, I. T., and Tsiamyrtzis, P. (2007).
Coalitional tracking. Comput. Vis. Image Underst.,
106(2-3):205–219.
Filipe, S. and Alexandre, L. A. (2013). Thermal infrared
face segmentation: A new pose invariant method. In
Pattern Recognition and Image Analysis, pages 632–
639. Springer.
Gault, T. R. and Farag, A. A. (2013). A fully automatic
method to extract the heart rate from thermal video.
In CVPRW 2013, pages 336–341. IEEE.
Ghiass, R. S., Arandjelovi
´
c, O., Bendada, A., and
Maldague, X. (2014). Infrared face recogni-
tion: A comprehensive review of methodologies and
databases. Pattern Recognition, 47(9):2807–2824.
Ghiass, R. S., Arandjelovic, O., Bendada, H., and
Maldague, X. (2013). Vesselness features and the in-
verse compositional aam for robust face recognition
using thermal ir. arXiv preprint arXiv:1306.1609.
Gross, R., Matthews, I., and Baker, S. (2005). Generic vs.
person specific active appearance models. Image and
Vision Computing, 23(12):1080–1093.
Kalal, Z., Mikolajczyk, K., and Matas, J. (2012). Tracking-
learning-detection. PAMI, 34(7):1409–1422.
Lahiri, B., Bagavathiappan, S., Jayakumar, T., and Philip,
J. (2012). Medical applications of infrared thermog-
raphy: a review. Infrared Physics & Technology,
55(4):221–235.
Lewis, G. F., Gatto, R. G., and Porges, S. W. (2011). A
novel method for extracting respiration rate and rel-
ative tidal volume from infrared thermography. Psy-
chophysiology, 48(7):877–887.
Matthews, I. and Baker, S. (2004). Active appearance mod-
els revisited. IJCV, 60(2):135–164.
Papandreou, G. and Maragos, P. (2008). Adaptive and con-
strained algorithms for inverse compositional active
appearance model fitting. In CVPR 2008, pages 1–8.
IEEE.
Zhou, Y., Tsiamyrtzis, P., Lindner, P., Timofeyev, I., and
Pavlidis, I. (2013). Spatiotemporal smoothing as a ba-
sis for facial tissue tracking in thermal imaging. IEEE
Trans. Biomed. Engineering, 60(5):1280–1289.
Zhu, X. and Ramanan, D. (2012). Face detection, pose es-
timation, and landmark localization in the wild. In
CVPR 2012, pages 2879–2886. IEEE.
Zhu, Z., Tsiamyrtzis, P., and Pavlidis, I. (2008). The seg-
mentation of the supraorbital vessels in thermal im-
agery. In AVSS 2008, pages 237–244.
VISAPP 2016 - International Conference on Computer Vision Theory and Applications
158