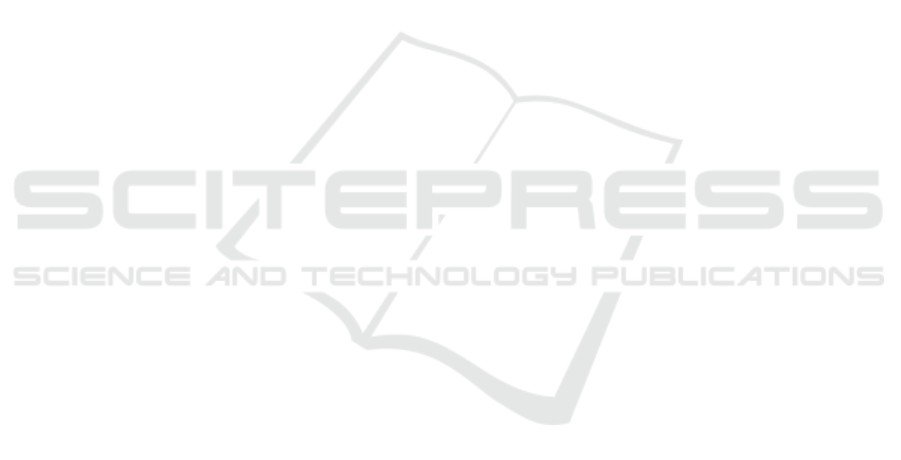
9 CONCLUSION
Correlation Coordinate Plots have been developed
with the specific task of correlation identification in
mind. They have distinct advantages when compared
to general task visualizations such as SCP and PCP.
The advantages, as confirmed by our user study, in-
clude:
• providing simple visual cues that make identifica-
tion of the existence and direction of correlation
fairly trivial;
• improving estimation of correlation strength by
focusing the coordinate system on model fit; and
• improving identification of linear, nonlinear, and
uncorrelated data by reducing ambiguity in the vi-
sualization.
In addition, the Snowflake Visualization showed
significant performance improvements over SPLOMs
and PCPs. The Snowflake Visualization is an efficient
focus+context style layout representing a fair compro-
mise between space efficient design, comprehensive
visualization, and reduced user interaction for show-
ing all pairwise correlations in multi-attribute data.
In conclusion, we believe that the CCP and
Snowflake Visualization represent complementary
approaches to existing techniques, replacing existing
approaches only where correlation is the major fea-
ture of focus in data. We believe that more of these
task specific approaches are on the horizon and will
provide data analysts better, faster access to relevant
information in their data.
ACKNOWLEDGEMENTS
We would like to thank our reviewers and colleagues
who gave us valuable feedback on our approach. We
would also like to thank our funding agents, NSF
CIF21 DIBBs (ACI-1443046), Lawrence Livermore
National Laboratory, and Pacific Northwest National
Laboratory Analysis in Motion (AIM) Initiative.
REFERENCES
Anscombe, F. J. (1973). Graphs in statistical analysis. In
American Statistical Association, pages 17–21.
Aris, A. and Shneiderman, B. (2007). Designing semantic
substrates for visual network exploration. In InfoVis,
pages 281–300.
Bertini, E., Tatu, A., and Keim, D. (2011). Quality metrics
in high-dimensional data visualization: An overview
and systematization. IEEE Trans. on Visualization and
Comp. Graphics, 17(12):2203–2212.
Bezerianos, A., Chevalier, F., Dragicevic, P., Elmqvist,
N., and Fekete, J.-D. (2010). Graphdice: A system
for exploring multivariate social networks. Computer
Graphics Forum, 29(3):863–872.
Buering, T., Gerken, J., and Reiterer, H. (2006). User in-
teraction with scatterplots on small screens - a com-
parative evaluation of geometric-semantic zoom and
fisheye distortion. IEEE Trans. on Visualization and
Comp. Graphics, 12(5):829–836.
Chen, Y. A., Almeida, J. S., Richards, A. J., Muller, P., Car-
roll, R. J., and Rohrer, B. (2010). A nonparametric
approach to detect nonlinear correlation in gene ex-
pression. Journal of Computational and Statistical
Graphics, 19(3):552–568.
Dang, T. N. and Wilkinson, L. (2014). Transforming
scagnostics to reveal hidden features. IEEE Trans.
on Visualization and Comp. Graphics, 20(12):1624–
1632.
Elmqvist, N., Dragicevic, P., and Fekete, J.-D. (2008).
Rolling the dice: Multidimensional visual exploration
using scatterplot matrix navigation. IEEE Trans. on
Visualization and Comp. Graphics, 14(6):1539–1148.
Fanea, E., Carpendale, M. S. T., and Isenberg, T. (2005).
An interactive 3d integration of parallel coordinates
and star glyphs. In InfoVis, pages 149–156.
Friendly, M. (2002a). Corrgrams: Exploratory displays
for correlation matrices. The American Statistician,
56(4):316–324.
Friendly, M. (2002b). Corrgrams: Exploratory displays for
correlation matrices. Ame. Stats, 1.
Geng, Z., Peng, Z., S.Laramee, R., Roberts, J. C., and
Walker, R. (2011). Angular histograms: Frequency-
based visualizations for large, high dimensional data.
IEEE Trans. on Visualization and Comp. Graphics,
17(12):2572–2580.
Harrison, L., Yang, F., Franconeri, S., and Chang, R.
(2014). Ranking visualizations of correlation using
weber’s law. IEEE Trans. on Visualization and Comp.
Graphics, 20(12):1943–1952.
Hartigan, J. A. (1975). Printer graphics for clustering.
JSCS, 4(3).
Heinrich, J., Stasko, J., and Weiskopf, D. (2012). The par-
allel coordinates matrix. In EuroVis - Short Papers,
pages 37–41.
Heinrich, J. and Weiskopf, D. (2013). State of the art of par-
allel coordinates. In Eurographics STAR, pages 95–
116.
Holten, D. and van Wijk, J. J. (2010). Evaluation of cluster
identification performance for different pcp variants.
EuroVis, 29(3).
Hong, X., Wang, C.-X., Thompson, J. S., Allen, B., Malik,
W. Q., and Ge, X. (2010). On space-frequency cor-
relation of uwb mimo channels. IEEE Trans. on Veh.
Tech., 59(9):4201–4213.
Huang, T.-H., Huang, M. L., and Zhang, K. (2012). An
interactive scatter plot metrics visualization for deci-
sion trend analysis. In Conf. on Machine Learning,
Applications, pages 258–264.
Inselberg, A. (1985). The plane with parallel coordinates.
The Visual Computer, 1(2):69–91.
IVAPP 2016 - International Conference on Information Visualization Theory and Applications
72