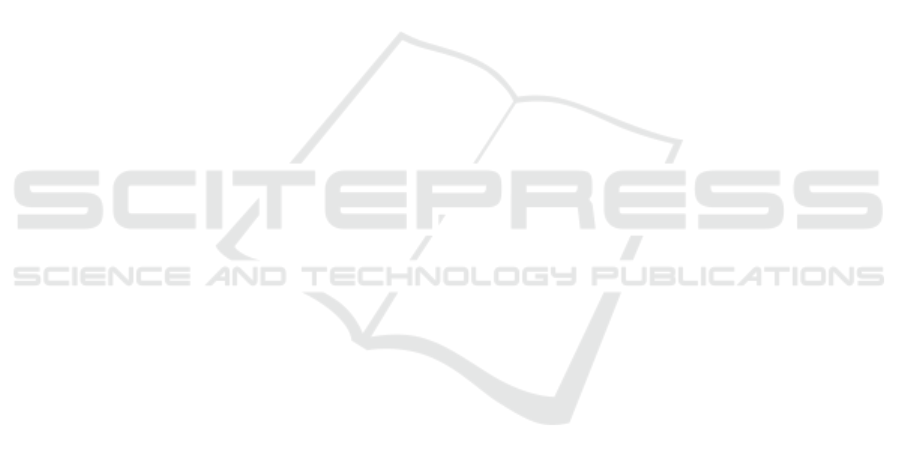
Electronic Imaging, volume 8667. International Soci-
ety for Optics and Photonics.
Hamming, R. W. (1950). Error detecting and error cor-
recting codes. The Bell System Technical Journal,
29(2):147–160.
Heinly, J., Dunn, E., and Frahm, J.-M. (2012). Compara-
tive evaluation of binary features. In Computer Vision
- ECCV 2012, Lecture Notes in Computer Science,
pages 759–773. Springer Berlin Heidelberg.
Jaakkola, T. and Haussler, D. (1998). Exploiting generative
models in discriminative classifiers. In In Advances
in Neural Information Processing Systems 11, pages
487–493. MIT Press.
J
´
egou, H., Douze, M., and Schmid, C. (2008). Ham-
ming embedding and weak geometric consistency for
large scale image search. In European Conference on
Computer Vision, volume I of LNCS, pages 304–317.
Springer.
J
´
egou, H., Douze, M., and Schmid, C. (2010a). Improving
bag-of-features for large scale image search. Interna-
tional Journal of Computer Vision, 87(3):316–336.
J
´
egou, H., Douze, M., and Schmid, C. (2011). Prod-
uct quantization for nearest neighbor search. Pat-
tern Analysis and Machine Intelligence, IEEE Trans-
actions on, 33(1):117–128.
J
´
egou, H., Douze, M., Schmid, C., and P
´
erez, P. (2010b).
Aggregating local descriptors into a compact image
representation. In IEEE Conference on Computer Vi-
sion & Pattern Recognition.
J
´
egou, H., Perronnin, F., Douze, M., S
`
anchez, J., P
´
erez,
P., and Schmid, C. (2012). Aggregating local im-
age descriptors into compact codes. IEEE Transac-
tions on Pattern Analysis and Machine Intelligence,
34(9):1704–1716.
Kaufman, L. and Rousseeuw, P. (1987). Clustering by
means of medoids. In An introduction to L1-norm
based statistical data analysis, volume 5 of Compu-
tational Statistics & Data Analysis.
Lee, S., Choi, S., and Yang, H. (2015). Bag-of-binary-
features for fast image representation. Electronics Let-
ters, 51(7):555–557.
Leutenegger, S., Chli, M., and Siegwart, R. (2011). BRISK:
Binary Robust invariant scalable keypoints. In Com-
puter Vision (ICCV), 2011 IEEE International Con-
ference on, pages 2548–2555.
Lowe, D. (2004). Distinctive image features from scale-
invariant keypoints. International Journal of Com-
puter Vision, 60(2):91–110.
Perronnin, F. and Dance, C. (2007). Fisher kernels on vi-
sual vocabularies for image categorization. In Com-
puter Vision and Pattern Recognition, 2007. CVPR
’07. IEEE Conference on, pages 1–8.
Perronnin, F., Liu, Y., S
`
anchez, J., and Poirier, H. (2010a).
Large-scale image retrieval with compressed fisher
vectors. In Computer Vision and Pattern Recogni-
tion (CVPR), 2010 IEEE Conference on, pages 3384–
3391.
Perronnin, F., S
`
anchez, J., and Mensink, T. (2010b). Im-
proving the fisher kernel for large-scale image classi-
fication. In Computer Vision - ECCV 2010, volume
6314 of Lecture Notes in Computer Science, pages
143–156. Springer Berlin Heidelberg.
Philbin, J., Chum, O., Isard, M., Sivic, J., and Zisserman, A.
(2007). Object retrieval with large vocabularies and
fast spatial matching. In Computer Vision and Pat-
tern Recognition (CVPR), 2007 IEEE Conference on,
pages 1–8.
Philbin, J., Chum, O., Isard, M., Sivic, J., and Zisserman,
A. (2008). Lost in quantization: Improving partic-
ular object retrieval in large scale image databases.
In Computer Vision and Pattern Recognition, 2008.
CVPR 2008. IEEE Conference on, pages 1–8.
Rublee, E., Rabaud, V., Konolige, K., and Bradski, G.
(2011). ORB: An efficient alternative to SIFT or
SURF. In Computer Vision (ICCV), 2011 IEEE In-
ternational Conference on, pages 2564–2571.
Salton, G. and McGill, M. J. (1986). Introduction to Mod-
ern Information Retrieval. McGraw-Hill, Inc., New
York, NY, USA.
S
`
anchez, J., Perronnin, F., Mensink, T., and Verbeek, J.
(2013). Image classification with the fisher vector:
Theory and practice. International Journal of Com-
puter Vision, 105(3):222–245.
Sivic, J. and Zisserman, A. (2003). Video google: A text re-
trieval approach to object matching in videos. In Pro-
ceedings of the Ninth IEEE International Conference
on Computer Vision, volume 2 of ICCV ’03, pages
1470–1477. IEEE Computer Society.
Thomee, B., Bakker, E. M., and Lew, M. S. (2010). TOP-
SURF: A visual words toolkit. In Proceedings of
the International Conference on Multimedia, MM ’10,
pages 1473–1476. ACM.
Tolias, G. and J
´
egou, H. (2013). Local visual query expan-
sion: Exploiting an image collection to refine local
descriptors. Research Report RR-8325.
Van Gemert, J., Veenman, C., Smeulders, A., and Geuse-
broek, J.-M. (2010). Visual word ambiguity. Pat-
tern Analysis and Machine Intelligence, IEEE Trans-
actions on, 32(7):1271–1283.
Van Opdenbosch, D., Schroth, G., Huitl, R., Hilsenbeck,
S., Garcea, A., and Steinbach, E. (2014). Camera-
based indoor positioning using scalable streaming of
compressed binary image signatures. In IEEE Inter-
national Conference on Image Processing.
Zezula, P., Amato, G., Dohnal, V., and Batko, M. (2006).
Similarity Search: The Metric Space Approach, vol-
ume 32 of Advances in Database Systems. Springer.
Zhang, Y., Zhu, C., Bres, S., and Chen, L. (2013). Encod-
ing local binary descriptors by bag-of-features with
hamming distance for visual object categorization. In
Advances in Information Retrieval, volume 7814 of
Lecture Notes in Computer Science, pages 630–641.
Springer Berlin Heidelberg.
Zhao, W.-L., J
´
egou, H., and Gravier, G. (2013). Oriented
pooling for dense and non-dense rotation-invariant
features. In BMVC - 24th British Machine Vision Con-
ference.
How Effective Are Aggregation Methods on Binary Features?
573