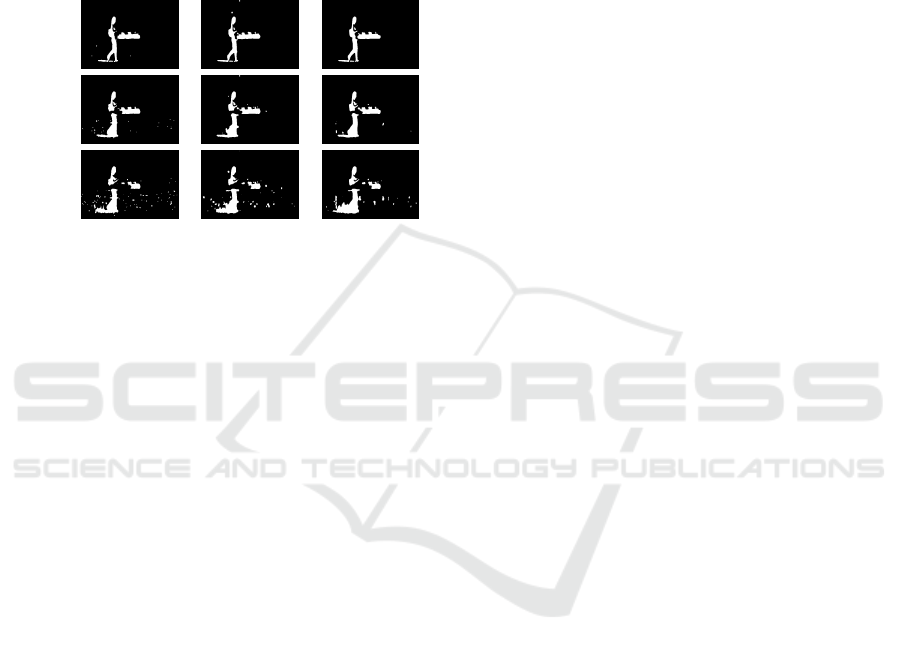
small patch sizes, the detection is more accurate.
For Boat1Person1 sequence, the processing time was
found to be 655 ms per frame at patch size 10x10,
while it was 2251 ms per frame at patch size 5x5. De-
spite better performance at patch size 5x5, the com-
putational overhead of performing background mod-
eling at that patch size is significantly higher than at
10x10 patch size for a small compromise in accuracy.
5x5 10x10 20x20
0.0001
0.005
0.01
Figure 6: Segmentation example of Boat1Person1 video
with different patch sizes and GMM learning rates.
4 CONCLUSIONS
The proposed method of spatio-temporal GMM of DT
is an accurate and robust mechanism for background
modeling and foreground detection. Evaluative per-
formance follows the hypothesis underpinning the
theoretical model. The relationships infused between
key parameters of patch size and learning rate indi-
cate that when the patch size is decreased, the number
of patches and hence the number of DT components
increases, thus yielding higher accuracy of detection
even at fixed learning rate. However, on the contrary,
with decrease in the patch size, the amount of motion
information that is encapsulated within each patch is
reduced, thereby causing slower recognition for the
motion patterns at that chosen learning rate.
REFERENCES
Bhaskar, H., Mihaylova, L., and Maskell, S. (2007). Back-
ground modeling using adaptive cluster density esti-
mation for automatic human detection. Informatics 2,
pages 130–134.
Chan, A., Mahadevan, V., and Vasconcelos, N. (2011). Gen-
eralized stauffer–grimson background subtraction for
dynamic scenes. Machine Vision and Applications,
22(5):751–766.
Chan, A. and Vasconcelos, N. (2009). Layered dynamic
textures. IEEE Transactions on Pattern Analysis and
Machine Intelligence, 31(10):1862–1879.
Dalley, G., Migdal, J., and Grimson, W. (2008). Back-
ground subtraction for temporally irregular dynamic
textures. WACV.
Doretto, G., Chiuso, A., Wu, Y. N., and Soatto, S. (2003).
Dynamic textures. International Journal of Computer
Vision, 51:91–109.
Ferryman, J. and Shahrokni, A. (2009). Pets2009: Dataset
and challenge. Twelfth IEEE International Workshop
on Performance Evaluation of Tracking and Surveil-
lance.
Goyette, N., Jodoin, P., Porikli, F., Konrad, J., and Ishwar, P.
(2012). Changedetection.net: A new change detection
benchmark dataset. In IEEE Computer Society Con-
ference on Computer Vision and Pattern Recognition
Workshops (CVPRW), pages 1–8.
Heikkila, M. and Pietikainen, M. (2006). A texture-based
method for modeling the background and detecting
moving objects. IEEE TPAMI, 28(4):657–662.
Mumtaz, A., Zhang, W., and Chan, A. B. (2014). Joint mo-
tion segmentation and background estimation in dy-
namic scenes. CVPR, pages 368–375.
Otsu, N. (1979). A threshold selection method from gray-
level histograms. IEEE TSMC, 9(1):62–66.
Pokrajac, D. and Latecki, L. J. (2003). Spatiotemporal
blocks-based moving objects identification and track-
ing. IEEE Visual Surveillance and Performance Eval-
uation of Tracking and Surveillance (VS-PETS), pages
70–77.
Stauffer, C. and Grimson, E. (2000). Learning patterns
of activity using realtime tracking. IEEE TPAMI,
22(8):747–757.
Stauffer, C. and Grimson, W. (1999). Adaptive background
mixture models for real-time tracking. CVPR, 2.
Tian, Y.-L. and Hampapur, A. (2008). Robust salient mo-
tion detection with complex background for real-time
video surveillance. WACV/MOTIONS.
Zhang, S., Yao, H., and Liu, S. (2009). Spatial-temporal
nonparametric background subtraction in dynamic
scenes. ICME, pages 518–521.
Zhong, B., Liu, S., Yao, H., and Zhang, B. (2009).
Multl-resolution background subtraction for dynamic
scenes. pages 3193–3196.
Zhong, J. and Sclaroff, S. (2003). Segmenting foreground
objects from a dynamic textured background via a ro-
bust kalman filter. ICCV, 1:44–50.
Zhou, X., Yang, C., and Yu, W. (2013). Moving object
detection by detecting contiguous outliers in the low-
rank representation. IEEE TPAMI, 35(3).
VISAPP 2016 - International Conference on Computer Vision Theory and Applications
410