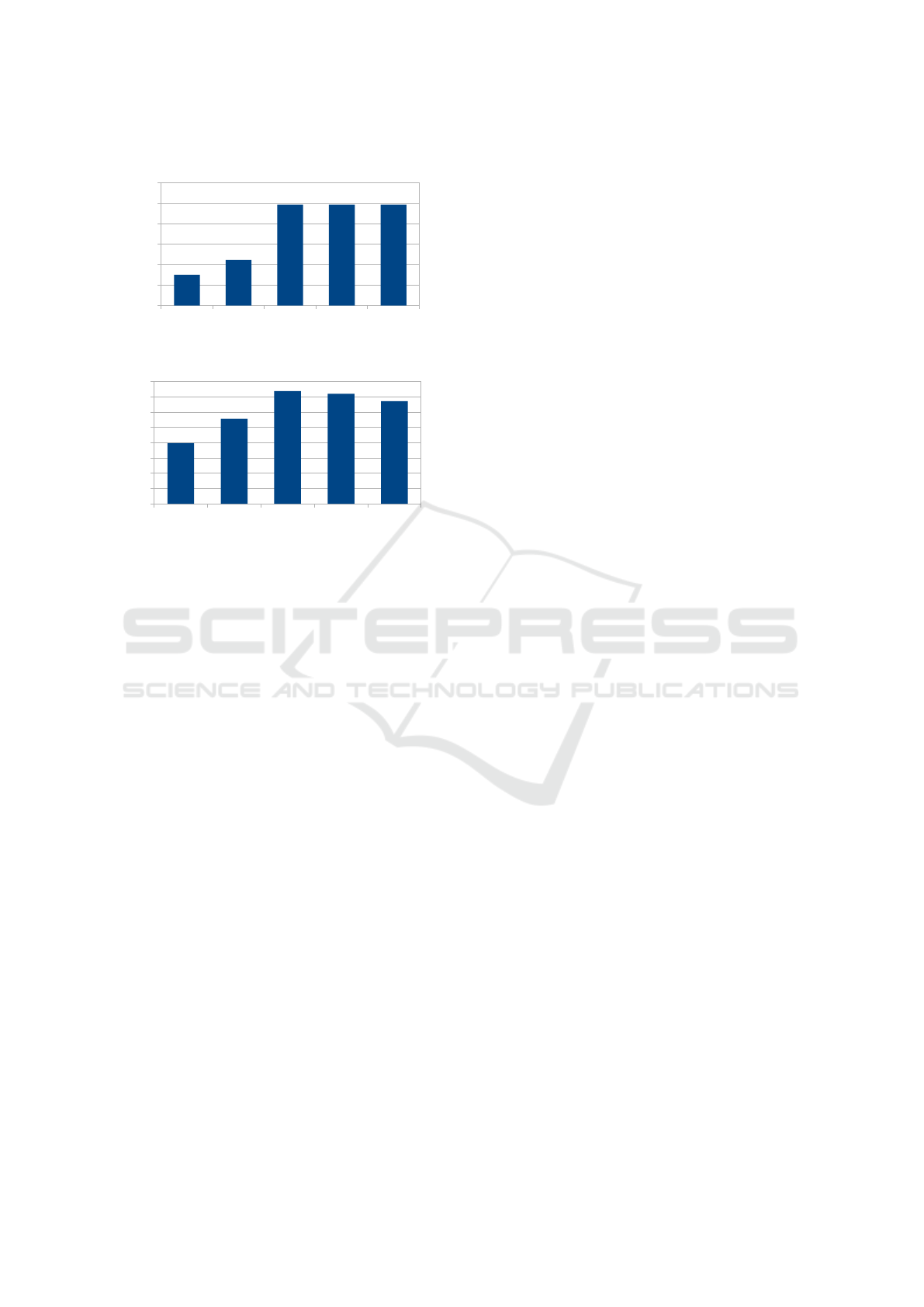
5 features/subset
10 features/subset
15 features/subset
20 features/subset
all 24 features without subset
0
50
100
150
200
250
300
350
400
runtime per query (in µs)
µs
5 features/subset
10 features/subset
15 features/subset
20 features/subset
all 24 features without subset
0
2000
4000
6000
8000
10000
12000
feature extraction of 1000 samples
ms
Figure 7: (Top) Runtime of a single matching query ordered
by the number of features per subset. (Bottom) Runtime of
feature extraction process with 1000 test images.
select pose-sensitive, significant local subsets, which
fit optimal to the depending pose-space region. In a
simple experiment it could be shown, that the method
decrease the runtime of feature extraction up to 50%
and of the matching process up to 41%. Depending
on the number of features and matching-method ef-
ficiency can even be improved. During this test-run
the accuracy of the pose matching was increased as
well, in case the number of features per subset was
not chosen to small. For future it is planned to ap-
ply this method to a fish tracking system with multi-
ple degree-of-freedom fish models and contour- and
keypoint-based features.
ACKNOWLEDGEMENTS
The presented work was developed within the scope
of the interdisciplinary, DFG-funded project virtual
fish of the Institute of Real Time Learning Systems
(EZLS) and the Department of Biology and Didactics
at the University of Siegen.
REFERENCES
Amanatiadis, A., Kaburlasos, V. G., Gasteratos, A., and Pa-
padakis, S. E. (2011). Evaluation of shape descriptors
for shape-based image retrieval. Image Processing,
IET, 5(5):493–499.
Chen, C., Yang, Y., Nie, F., and Odobez, J.-M. (2011). 3d
human pose recovery from image by efficient visual
feature selection. Computer Vision and Image Under-
standing, 115(3):290–299.
Chen, C., Zhuang, Y., and Xiao, J. (2010). Silhouette rep-
resentation and matching for 3d pose discrimination–
a comparative study. Image and Vision Computing,
28(4):654–667.
Chen, C., Zhuang, Y., Xiao, J., and Wu, F. (2008). Adaptive
and compact shape descriptor by progressive feature
combination and selection with boosting. In Computer
Vision and Pattern Recognition, 2008. CVPR 2008.
IEEE Conference on, pages 1–8. IEEE.
Choi, C., Christensen, H., et al. (2010). Real-time 3d
model-based tracking using edge and keypoint fea-
tures for robotic manipulation. In Robotics and Au-
tomation (ICRA), 2010 IEEE International Confer-
ence on, pages 4048–4055. IEEE.
Collet, A., Martinez, M., and Srinivasa, S. S. (2011). The
moped framework: Object recognition and pose esti-
mation for manipulation. The International Journal of
Robotics Research, page 0278364911401765.
Hinterstoisser, S., Benhimane, S., and Navab, N. (2007).
N3m: Natural 3d markers for real-time object detec-
tion and pose estimation. In Computer Vision, 2007.
ICCV 2007. IEEE 11th International Conference on,
pages 1–7. IEEE.
Kazmi, I. K., You, L., and Zhang, J. J. (2013). A survey of
2d and 3d shape descriptors. In Computer Graphics,
Imaging and Visualization (CGIV), 2013 10th Inter-
national Conference, pages 1–10. IEEE.
M
¨
uller, K., Schlemper, J., Kuhnert, L., and Kuhnert, K.-
D. (2014). Calibration and 3d ground truth data gen-
eration with orthogonal camera-setup and refraction
compensation for aquaria in real-time. In VISAPP (3),
pages 626–634.
Payet, N. and Todorovic, S. (2011). From contours to 3d
object detection and pose estimation. In Computer Vi-
sion (ICCV), 2011 IEEE International Conference on,
pages 983–990. IEEE.
Pons-Moll, G. and Rosenhahn, B. (2011). Model-based
pose estimation. In Visual analysis of humans, pages
139–170. Springer.
Poppe, R. and Poel, M. (2006). Comparison of silhou-
ette shape descriptors for example-based human pose
recovery. In Automatic Face and Gesture Recogni-
tion, 2006. FGR 2006. 7th International Conference
on, pages 541–546. IEEE.
Rasines, I., Remazeilles, A., and Iriondo Bengoa, P. M.
(2014). Feature selection for hand pose recognition
in human-robot object exchange scenario. In Emerg-
ing Technology and Factory Automation (ETFA), 2014
IEEE, pages 1–8. IEEE.
Reinbacher, C., Ruether, M., and Bischof, H. (2010).
Pose estimation of known objects by efficient silhou-
ette matching. In Pattern Recognition (ICPR), 2010
20th International Conference on, pages 1080–1083.
IEEE.
VISAPP 2016 - International Conference on Computer Vision Theory and Applications
206